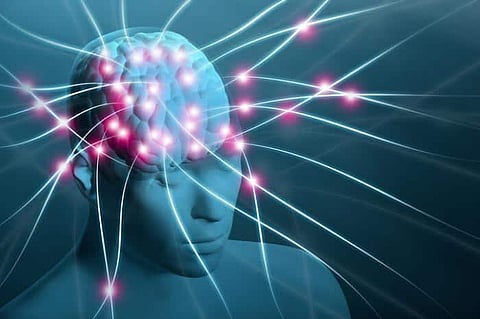
Deep learning which is also termed as hierarchical learning or deep structured learning uses a layered algorithmic architecture to analyze data. Its peculiarity helps organizations and researchers achieve the unachievable in a most innovative manner. Recently, researchers at the University of Eastern Finland developed a new deep learning model that can identify sleep stages as accurately as an experienced physician. This discovery paves the way for better diagnosis and treatments of sleep disorders, including obstructive sleep apnea.
To note, obstructive sleep apnea (OSA) is a nocturnal breathing disorder that causes a major burden on public health care systems and national economies. As noted by a report, it is estimated that up to one billion people worldwide suffer from obstructive sleep apnea, and the number is expected to grow due to population aging and increased prevalence of obesity. When untreated, OSA increases the risk of cardiovascular diseases and diabetes, among other severe health consequences.
Therefore, it wise to have a system that can identify sleep stages for the diagnostics of sleep disorders, including obstructive sleep apnea. Traditionally, sleep is manually classified into five stages, which are wake, rapid eye movement (REM) sleep and three stages of non-REM sleep. However, manual scoring of sleep stages is time-consuming, subjective and costly as well.
Hence to win against such challenges, researchers used polysomnographic recording data from healthy individuals and individuals with suspected OSA to develop an accurate deep learning model for automatic classification of sleep stages. In addition, they wanted to find out how the severity of OSA affects classification accuracy.
In healthy individuals, the model was able to identify sleep stages with an 83.7 percent accuracy when using a single frontal electroencephalography channel (EEG), and with an 83.9 percent accuracy when supplemented with electrooculogram (EOG). In patients with suspected OSA, the model achieved accuracies of 82.9 percent (single EEG channel) and 83.8 percent (EEG and EOG channels). The single-channel accuracies ranged from 84.5 percent for individuals without OSA to 76.5 percent for severe OSA patients. The accuracies achieved by the model are equivalent to the correspondence between experienced physicians performing manual sleep scoring. However, the model has the benefit of being systematic and always following the same protocol, and conducting the scoring in a matter of seconds.
According to the researchers, deep learning enables automatic sleep staging for suspected OSA patients with high accuracy.
The Sleep Technology and Analytics Group, STAG, at the University of Eastern Finland solves sleep diagnostics challenges by using a variety of different approaches. The methods developed by the group are based on wearable, non-intrusive sensors, better diagnostic parameters and modern computational solutions that are based on artificial intelligence. The new methods developed by the group are hoped to improve OSA severity assessment, promote individualized treatment planning and more reliable prediction of OSA-related daytime symptoms and comorbidities.
As noted by the American Academy of Sleep Medicine, the researchers hope the deep learning model can be used to improve the consistency of sleep staging across providers and systems while also completing the scoring in mere seconds. They also noted the potential cost savings by measuring sleep with fewer channels. Ultimately, the researchers think their methods could improve sleep apnea severity assessment, promote individualized treatment planning, and more reliably predict sleep apnea-related daytime symptoms and comorbidities. The research originated at the Sleep Technology and Analytics Group at the University of Eastern Finland, which was created to examine challenges in sleep diagnostics.
Join our WhatsApp Channel to get the latest news, exclusives and videos on WhatsApp
_____________
Disclaimer: Analytics Insight does not provide financial advice or guidance. Also note that the cryptocurrencies mentioned/listed on the website could potentially be scams, i.e. designed to induce you to invest financial resources that may be lost forever and not be recoverable once investments are made. You are responsible for conducting your own research (DYOR) before making any investments. Read more here.