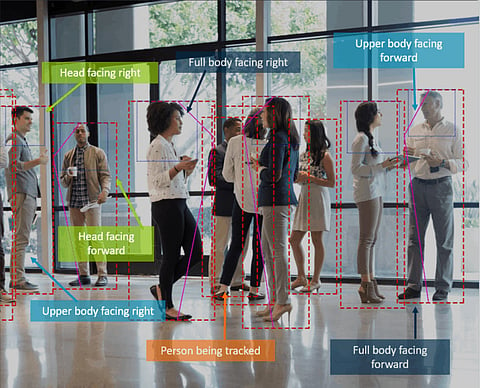
Analyzing live videos by leveraging deep learning is the trendiest technology aided by computer vision and multimedia analysis. Analysing live videos is a very challenging task and its application is still at nascent stages. Thanks to the recent developments in deep learning techniques, researchers in both computer vision and multimedia communities have been able to gather momentum to drive business processes and revenues.
Whenever you want to express by non-verbal signs you use your hands to communicate, like whenever a traffic cop raises his hand you know he is signalling you to stop till directed. Though easier for mankind, technology finds it harder to work on people's reaction to predict their next behaviour.
Agency for Science, Technology and Research or A*STAR researchers from the Ministry of Trade and Industry, Singapore have developed a detector that can successfully pick out where human actions will occur in videos, in almost real-time through deep learning technologies.
Hongyuan Zhu, a computer scientist at A*STAR's Institute for Infocomm Research adds, "Harnessing live videos to understand human intentions need an improved image analysis technology to be employed to a wide range of applications". Live video analysis will power driverless cars to detect police officers and interpret their actions quickly and accurately for a safe commute. Further, these autonomous systems could also be trained to single out suspicious activities like fighting, theft and alert security officers accordingly. This will be a boom for social safety and a blessing for law and order authorities
Credit to deep learning techniques, computers can detect objects in static images accurately through the use of artificial neural networks to process complex image information. However, videos with moving objects are more challenging for computers to intercept information. Hongyuan Zhu further adds, "Understanding human actions in videos is a necessary step forward to build smarter and friendlier machines".
The earlier techniques used for locating and analysing potential human actions in videos did not use deep learning frameworks thus were slow and inaccurate. To overcome this, the A*STAR's Institute researchers developed the YoTube detector which combines two types of neural networks that run parallel, a static neural network which is proved to be accurate to process still images, and a recurring neural network used for speech processing data to understand speech recognition algorithms. A*STAR asserts that its method is the first to bring detection and tracking together in one deep learning pipeline.
To prove, the team tested YoTube on more than 3,000 videos routinely that are used in computer vision experiments. They reported that YoTube outperformed state-of-the-art detectors at correctly picking out potential human actions by approximately 20 percent for videos showing general everyday activities and around 6 percent for sports videos.
YoTube has overcome many challenges towards where it stands today. However, the deep learning powered detector occasionally makes mistakes. Mistakes or loopholes include the instances when the people in the video are small, or if there are many people in the background. Nonetheless, A*STAR's Zhu concludes by saying, "We have demonstrated that we can detect most potential human action regions in an almost real-time manner."
Join our WhatsApp Channel to get the latest news, exclusives and videos on WhatsApp
_____________
Disclaimer: Analytics Insight does not provide financial advice or guidance. Also note that the cryptocurrencies mentioned/listed on the website could potentially be scams, i.e. designed to induce you to invest financial resources that may be lost forever and not be recoverable once investments are made. You are responsible for conducting your own research (DYOR) before making any investments. Read more here.