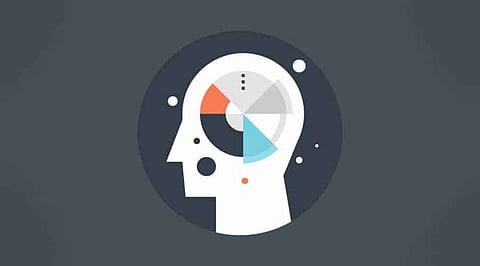
There is a renaissance occurring in the field of artificial intelligence. For some drawn-out specialists in the field, it isn't excessively self-evident. Many are making against the advancements of Deep Learning. Deep Learning is anyway an amazingly radical departure from classical methods.
Old style A.I. procedures has zeroed in generally on the legitimate premise of cognition, Deep Learning by contrast works in the territory of cognitive intuition. Deep learning frameworks display behavior that seems biological despite not being founded on biological material. It so happens that humankind has fortunately discovered Artificial Intuition as Deep Learning.
Artificial intuition is a simple term to misconstrue since it seems like artificial emotion and artificial empathy. In any case, it contrasts fundamentally. Scientists are dealing with artificial intuition so that machines can impersonate human behavior all the more precisely. Artificial intuition plans to distinguish a human's perspective in real-time. Along these lines, for instance, chatbots, virtual assistants and care robots can react to people all the more appropriately in context. Artificial intuition is more similar to human intuition since it can quickly evaluate the totality of a situation, including subtle indicators of a specific activity.
Modern-day companies use machine learning to distinguish outliers and patterns that speak to potential threats and vulnerabilities. An exemplary challenge for cybersecurity vendors is that a high level of false positives can cause "alert fatigue." Alert fatigue is perilous in light of the fact that it makes people overlook a danger they're attempting to forestall. The other issue is false negatives that neglect to distinguish the unwanted behavior.
Anderson describes Reductionist strategies as having the below mentioned characteristics:
• Completeness: We endeavor to find the most ideal solution.
• Culmination: We endeavor to get all solutions.
• Repeatability: We hope to get a similar outcome each time we repeat an examination under similar conditions.
• Timeliness: We hope to get the outcome in limited time.
• Parsimony: We endeavor to find the easiest hypothesis that completely clarifies the accessible information.
• Transparency: We need to see how we showed up at the outcome.
• Scrutability: We need to comprehend the outcome.
In spite of all the cybersecurity investments organizations make, they're regularly one step behind cybercriminals on the grounds that a few patterns are too unpretentious to even think about detecting.
Once in a while a step change is important to have a critical effect. That is the thing that Ronald Coifman, Phillips professor of mathematics at Yale University, and Amir Averbuch, professor of computer science at Tel Aviv University, have been endeavoring to accomplish for as far back as decade. They built up a bunch of "artificial intuition" algorithms that find faint signs in big data that other approaches miss.
Anderson guesses that the logic-based methodology should be deserted for an option 'model-free' approach. That is, instinct-based cognition can't emerge from reduction based principles. What Anderson portrays a 'sans model' are 'unintelligent parts", that is she composes:
In the event that you are endeavoring to construct an intelligent system from insightful parts, at that point you are simply pushing the issues down one level.
Anderson proposes a few 'without model' components, that the blend of which, can prompt emergent behavior that we see in intuition.
"Computational instinct" is most likely a more precise term since team's algorithms analyze relationships in data as opposed to dissecting data values, which is commonly how AI works. In particular, algorithms can recognize new and already undetected patterns, for example, cybercrime happening in what gives off an impression of being benign transactions. For instance, Coifman and Averbuch's algorithms have recognized $1 billion worth of nominal money transfers (e.g., $25 worth) from millions of bank accounts in various nations that supported a notable terrorist group.
Join our WhatsApp Channel to get the latest news, exclusives and videos on WhatsApp
_____________
Disclaimer: Analytics Insight does not provide financial advice or guidance on cryptocurrencies and stocks. Also note that the cryptocurrencies mentioned/listed on the website could potentially be scams, i.e. designed to induce you to invest financial resources that may be lost forever and not be recoverable once investments are made. This article is provided for informational purposes and does not constitute investment advice. You are responsible for conducting your own research (DYOR) before making any investments. Read more here.