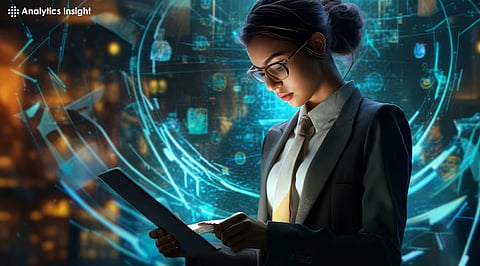
Data science is important because it combines tools, methods, and technology to generate meaning from data. Data, however, is only helpful if harnessed and analyzed for a given purpose; that is where the in-demand field of data science comes in, and there is a demand for data science job titles.
Data science has since combined the ranges of data science statistics domain expertise to extract knowledge from information. The data science job titles themselves are multifaceted, but a data science wrangler, a data science explorer, and a data science miner are some of them. Each has some required skills in a particular stage of the data's journey.
The article delves into the top 10 data science jobs titles and the responsibilities, requirements, and paths associated with them.
The data scientist is the most recognizable title of all data science roles because it is the group's generalist and a combination of statistical modeling, machine learning, and programming skills. Data scientists usually take a lifecycle approach to data.
Data Wrangling: Transformation of raw data into a clean, structured form for analysis.
Exploratory Data Analysis: Making sense of data by finding trends and patterns.
Modeling: Formulation of statistical or machine learning models to answer business questions.
Communication: Communicating insights or findings clearly and concisely to stakeholders.
Skills: Programming languages (Python, R), statistics, machine learning, and data visualization.
Career Path: Data Analyst, Machine Learning Engineer, and Manager of a Data Science team.
Data analysts are one of the top 10 most in-demand data science jobs. They are more like data detectives who deal with a sequence of information and extract any trends or patterns. Usually, their focus is on analyzing past information that gives some insight into a business.
Querying databases and data warehouses
Visualization in the form of text or other graphical representations
Selecting key measures and KPIs
Reporting data findings used to make business decisions
Skills: SQL, data visualization tools: Tableau, Power BI, data wrangling techniques. advanced statistics
Career Path: Business Analyst, Data Scientist, Data Engineer.
Data engineers are data infrastructure architects. They design, develop, and supervise the pipelines used in data collection, storage, and further processing.
Design and management of the data warehouse and data lakes
Creation of a data pipeline for data ingestion and transformation
Design and Implement data quality checks
Experience in using AWS, Azure, and GCP.
Skills Required: Programming languages like Python, Java, or Scala, frameworks of big data – Spark, Hadoop, distributed systems knowledge, and experience in cloud computing.
Career Path: Data Analyst, Data Architect, Big Data Engineer.
Data architects are the strategic thinkers of the data world. They conceptualize and manage an organization's overall data architecture to ensure that data is accessible, secure, and scalable.
Data governance policies and standards.
Design of data models to be able to store and retrieve data efficiently.
Selection and design of proper data technologies.
Collaboration with multiple stakeholders around business functions and across the lines of business
Skills Domain: Data modeling techniques, Database Management Systems, Data Warehousing concepts, Big data frameworks
Career Path: Data Engineer, Data Scientist, Chief Data Officer - CDO.
Machine learning engineers are the constructors who bring machine learning models to life. They take the models developed by data scientists and turn them into applications that are ready for production.
Providing machine learning models in production.
Automation of workflows for machine learning with tools such as MLflow.
Monitoring and supporting machine learning model performance.
Building and managing machine learning infrastructure.
Skills: Languages like Python, libraries for machine learning — TensorFlow, PyTorch, principles of Software Engineering, and experience in cloud computing.
Career Path: Data Scientist, Deep Learning Engineer, Robotics Engineer.
A data storyteller bridges the gap between data and decision-making. The data storytelling process involves taking data-driven findings and turning them into attractive stories throughout their cycle, which draws in decision-makers.
Make compelling data visualization to be clear, concise, and beautiful.
Have data insight communicated in a non-technical way.
Tailoring data stories to specific audiences and objectives.
Skills: Data visualization tools, storytelling techniques, communication skills, business acumen.
Career Path: Data Analyst, Data Scientist
BIDs are the point of contact if the business needs to design and develop any BI tools or dashboards. They translate the needs of the business into viable data through which the company can make decisions.
Designing and developing business intelligence dashboards and reports.
Bringing all data sources together on one platform.
Collaboration with the stakeholders to understand the business needs
Designing the KPIs and deploying them
Skills required: SQL and Python languages, different BI tools like Tableau or Power BI, data visualization, and business acumen.
Career Path: A Data Analyst can transform into a Data Engineer or Business Analyst.
The DBA is the custodian of the data empire. A Database Administrator is responsible for ensuring that the database's planned operation, safety, and performance are performed accordingly.
Back up and retrieve in case of failures.
Optimize database performance to make data access fast.
Secure available data to protect sensitive data from unauthorized exposure.
Skills: SQL, Database administration Tools, Data security principles, and knowledge of database systems like MySQL or PostgreSQL
Career Path: Data Analyst, Data Engineer, Data Architect
Statisticians use statistical methods to identify trends, patterns, and correlation patterns in the data
Design and implement statistical tests.
Identify statistical models and techniques for analyzing data to test hypotheses and reach a conclusion.
Design statistical models that can provide a reliable prediction of the outcome in the future.
Present in a lucid manner the outcome of such a statistical analysis.
Ability to work with statistical data analysis software -R, SAS, probability theory, hypothesis testing, and statistical modeling techniques
Career path: Data Analyst, Data Scientist, Quantitative Analyst
Data and Analytics Managers are the conductors of the data science team. They are responsible for end-to-end management of the data science lifecycle: data acquisition, data analysis, and communication of insights.
Creation of the data science strategy for the organization
Acquisition, hiring, and management of data science talent
Setting priorities driven by business needs for data-science projects
Ensure that data science projects are valuable to the organization.
Skills: Leadership, communication and business knowledge, understanding of different data science disciplines
Career Path: Data Scientist, Data Architect, Chief Data Officer.
Here is a diverse and exciting constellation of occupations available to data scientists. In this age of enhanced relevance to data, a career in data science is expected to remain in high demand for the foreseeable future. If you have a passion for solving problems and making things better through data, you are called to data science.
The data science world spreads its scatter plot of opportunities before you as each function forms a different note in the grand symphony of mining data to generate value for its clients. The article discussed the top 10 data science job titles, together with their unique sets of responsibilities, skill areas, and career progressions. So, from a data wrangler whiz kid to a machine learning maestro, the opportunities in data science are just promising waves at the new doors for you.
There's only one educational path for data science. A bachelor's degree in computer science, statistics, mathematics, or a related field is a solid foundation. Many professionals pursue Master's degrees in data science or related fields to gain a deeper understanding of specific areas.
Programming languages like Python and R, statistical analysis, machine learning expertise, and data visualization are highly sought-after skills. Communication and business acumen are also increasingly crucial for translating data insights into actionable solutions.
Absolutely. Data science is a rapidly growing field with high demand for data science job titles and promising salaries. If you enjoy tackling challenges with data and have a passion for making a difference, data science could be the perfect fit.
Build your skillset through online courses, bootcamps, or self-study. Participate in data science hackathons and personal projects to gain experience. Network with professionals in the field and consider an entry-level data analyst role to launch your data science journey.
Expect to see a surge in specializations like artificial intelligence, deep learning, and natural language processing. Cloud computing and big data frameworks will play an increasingly crucial role. The ability to translate data insights into clear and actionable business strategies will remain the same.