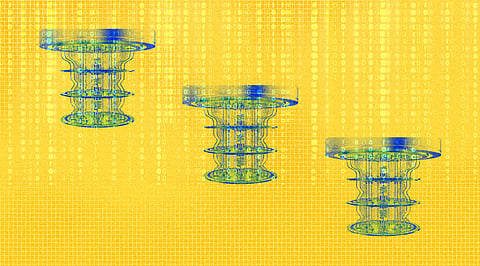
Quantum Computing is one of the most rapidly growing technology. Its aided benefits and applications, especially in Data Science and Machine Learning fields by using AI tools and Algorithms, are offering much easier handling and computing a huge volume of data. Although it's not as common a replacement of a traditional computer, right at the moment, for the commoners, as much useful it is for the Data Scientists, there is no denying that its development will continue to accelerate in the coming decades and soon may become a daily part of our lives.
Quantum Computing is a fusion of quantum physics, computer science, and information theory. The study of quantum computing is a subfield of quantum information science. The quantum circuit model, quantum Turing machine, adiabatic quantum computer, one-way quantum computer, and various quantum cellular automata are all examples of quantum computers (also known as quantum computing systems). The quantum circuit, which is based on the quantum bit, or "qubit," is the most extensively used model. Instead of using binary bits (0 and 1), these qubits are used.
The disadvantage of traditional computing is that the calculations are done only once at a time, which really is inefficient when dealing with massive amounts of data. The qubits are expressed as zero or one in quantum computing, and the concept of superposition, i.e. both at the same time, is used (0 and 1 at the same time). Quantum computing has the property of making computation easier by lowering the number of operations required to solve a complicated and time taking problem. If more bits are used in the computation, there will be a greater advantage of Quantum Computing over traditional computers.
In the scientific literature, there is widespread agreement that quantum computers will aid in the solving of previously impossible issues, particularly in the disciplines of data science and artificial intelligence. However, no flawless quantum computers are currently accessible. Noisy Intermediate-Scale Quantum (NISQ) is the name given to the present generation. These computers have a restricted amount of bits and are susceptible to interference as well as noise. IBM and QuEra Computing were among the first businesses to construct quantum computers with more than 100 qubits in 2021. But what is this generation's practical benefit?
This can be understood in a practical test, in which the authors used the Qiskit and PennyLane frameworks to construct three use cases and confirmed their practical usefulness. In comparison to competitors such as Google's Cirq and Microsoft's Q#, IBM's Qiskit framework has excellent documentation and has the added benefit of being able to execute circuits on a genuine quantum computer for free.
A true data scientist will most certainly work with much more data, yet in general, people are incapable of analyzing massive databases on their own. To assess these big datasets, ML algorithms are deployed. So every time fresh data is given, they figure out how to interpret the changes and look for patterns in the data. As a data scientist continue to add additional data, the amount of time it takes to analyze and compute grows.
The processing capacity of traditional computers limits the computational capability of machine learning algorithms today. Quantum computing can process enormous data sets at much quicker speeds and feed data to AI technologies, which can analyze data at a finer level to find patterns and abnormalities. One advantage of using quantum computers is that we can do more advanced analysis and construct machine learning models. It also makes it so much easier to use more data, allowing data scientists to have a better understanding of the data they are working with.
Join our WhatsApp Channel to get the latest news, exclusives and videos on WhatsApp
_____________
Disclaimer: Analytics Insight does not provide financial advice or guidance on cryptocurrencies and stocks. Also note that the cryptocurrencies mentioned/listed on the website could potentially be scams, i.e. designed to induce you to invest financial resources that may be lost forever and not be recoverable once investments are made. This article is provided for informational purposes and does not constitute investment advice. You are responsible for conducting your own research (DYOR) before making any investments. Read more here.