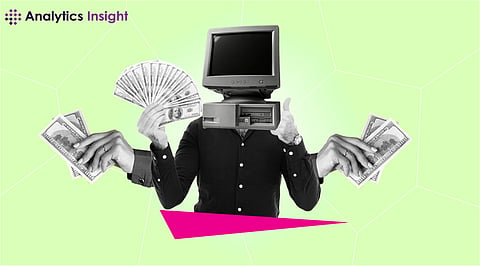
-------
Transitioning from learning to earning in any field is challenging. The job markets have been competitive in recent years but with the right amount of skills, techniques and strategies. The demand for data science is increasing yet challenging to land a career in this field.
Let us look at how we can make the transition from learning to earning in data science. For this to happen, we require skills that improve your resume - both technical and soft skills. Soft skills such as effective communication, and crisis management are important for someone willing to work in the data science industry.
Finding data science careers just after graduation can be challenging but still possible with skills, a good data science portfolio along the best data science networking that can help you through the journey.
Having a degree in data science, courses in data science, or self-learning are a great path if you want to continue earning in data science from the learning phase. A good foundation in the field is great.
While an academic degree does not guarantee work as a data scientist, it does equip you with holistic and structured knowledge. It is much easier to develop this knowledge later in life than to start afresh to become a data scientist.
Data scientists usually have a bachelor's degree in a quantitative discipline like computer science, statistics, mathematics, or even economics.
A master's degree is a sure way of increasing your chances of being employed. You can specialize in it. Specializations include things like business intelligence, data analysis, and machine learning.
The primary responsibilities of a data scientist are to extract and manipulate data as well as create, test, and implement machine learning models.
Data scientists need to use all of this knowledge by using a variety of computer languages and tools for instance: programming, stats, cloud computing, SQL, Python, R, data manipulation, analysis and visualization, MS SQL server, tableau, power BI, AWS, Microsoft Azure, Google cloud etc.
Communication Skills
These involve expressing your ideas as well as hearing what others have to say.
As a data scientist, your first task is to listen to the issues that others are facing. You are the type of psychotherapist who uses facts to assist clients in finding solutions to their issues.
Additionally, non-technical audiences need to be able to understand the technical intricacy of data scientists' work. They assist themselves using visualization tools, thus it's imperative that you properly visualize and show your job.
Collaboration skills
Collaboration with other members of the data team is a common requirement for data scientists. Cross-departmental teams are also a feature of projects.
Adaptability and flexibility, fostering a positive work environment, and skillfully resolving issues
Data science portfolio
Having a portfolio is important as compared to a resume. Most of them, are figuring out how to build one. To start with, find a data science project you are passionate about, it can be solving a problem or something you want to learn.
Go online, talk to someone you know, find people who may have problems in this field, followed by pitching your idea to solve it and continue to do so to build a strong portfolio that will help you get hired in the future.
Data science network:
Along with the educational background, skills and portfolio you will share to the potential recruiters, a data science network can be a lot helpful. Having people connected in the field you are interested to work and earn is essential. To do that, you can go on Linkedin, search for people who work in a similar field, find mentors and take inputs, advices for them and even these connections are helpful for giving you referrals.
Overall, having a good background, skills, portfolio and network can help you make the transition from learning to earning in data science. How to build up skills can be confusing but consider yourself doing some online courses on technical skills and doing some online professional certifications that can give a whole good picture of data science. Along with these skills comes building the portfolio and network.