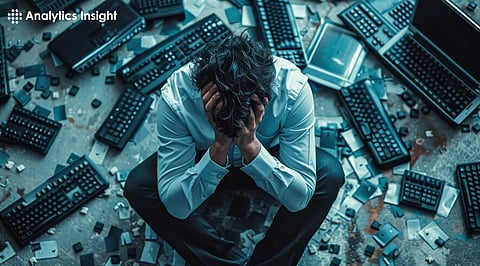
There are many myths and realities about data science that can scare away anyone aspiring to become a data scientist. This post aims at differentiating Data science myths vs. reality. to help you understand the truth about embarking on the field. Furthermore, this article helps unravel what data science involves in detail.
Many people have a common data science myth that you must have a master’s or Ph.D. degree in this area—this is wrong completely. In fact, even people holding undergraduate degrees can be employed as Data Scientists. However, mastery of relevant skills is more important. You need to gain expertise in all necessary skills; so when starting as a newbie it is advisable that you work on real projects from start to finish and then apply for internships so that later on it would be easier to land a permanent job. Though having a master’s degree or PhD can increase your chances of landing a lucrative job, it is crucial to remember that there are still opportunities like getting certification courses for those who do not have them. From this we can infer that it is helpful but not necessary for people to have masters and Ph.D.
Once again, the answer is the same: it is not necessary to have a certificate, but it's good if you do. This is because real-world data is quite different from what we study while doing courses and knowing data science matters more than possessing a certificate. A certificate on a resume tells an employer about a course that one has done that they are likely to be quizzed about its concept before employment other than the work experience. In summary, it is clear that expertise in data science is better than having a piece of paper showing that you passed somewhere.
This is another data science myth that should be dispelled. The reality of data science is not a single job it takes on many forms. Be sure to use your past experience for good. Don't underrate the experience you have gained from your previous job if you want to change fields and try the path to data science. Project works and internships for people who are just starting out help a lot.
You don’t have to come from one of these, any other person from different backgrounds have made it in this field. Especially, people with no technology background are now able to secure placements in this field due to up-skilling in software development. In the modern days, you can easily learn how to program because of so many available free resources. What matters most is the volume and quality of effort used in acquiring them, it will secure you a job regardless of your background.
That is absolutely false. In data science we do things like: Talking with the product owner, gathering requirements, understand what important data is about, following project lifecycle, and storing data optimally in other databases. Similarly, the lifecycle process involves steps such as: Data collection, Data cleansing, Data pre-treatment, Exploratory data analysis (EDA) Feature engineering Model, training Model assessment, Model update with hyperparameter.
This is yet another misconception in the AI realm. It is essential to realize that the primary goal of Kaggle competitions is to demonstrate to yourself that you possess the requisite data science project lifecycle skills, rather than model deployment. Data in real life often keeps changing hence there is always a need to figure out which form it takes at particular times as well as conduct model optimization. Nevertheless undertaking challenges on Kaggle or participating in Hackathons would be beneficial as it gives one a strong foundation on the project life cycle.
There’s a popular misconception or data science myth about this. Given your understanding of what data science projects entail in terms of process, the time consumption for that work can be seen from above. The data scientist spends most of his/her time Cleaning and Organizing data used for analysis which can take up to 60% of this time. The model building process takes less than 5% of the total time allocated for any given task which is an evidence that it does not consume many hours compared to resting parts of such activities. Therefore we can now infer that majority of this time is focused on other activities that are meant to make meaningful sense from those models that have already been built.
The above statement is one of the biggest misconceptions. You do not have to know everything about coding anymore; there are many open sources around nowadays making it easier to master them easily in case you hit roadblocks you can just google for help. Do bear in mind as for memorization, no single line of code needs to be stored in mind; you must only develop excellent logic skills.
That's not true at all. Data Science Facts says you have to work hard to develop your skills if you are a beginner. To get practical knowledge, start working on various projects. Demonstrate your ability by applying for internships that match your potential and capabilities. Developing a strong portfolio will also require working on full project life-cycles. The only advice you need is to stick to it because the moment you stop is when everything crumbles down.
Being a data scientist hardly depends on academic training and qualifications. Being one is not only reserved for particular people, but also is about varying learning approaches that need to be mastered by everyone including personal and college inexperienced minds. In order to succeed, the key is acquiring new knowledge, changing as well as applying data science methods when handling difficult situations. Developing skills and getting practical knowledge is what can help anyone make a significant profession out of numbers if they are passionate about it.
Join our WhatsApp Channel to get the latest news, exclusives and videos on WhatsApp
_____________
Disclaimer: Analytics Insight does not provide financial advice or guidance. Also note that the cryptocurrencies mentioned/listed on the website could potentially be scams, i.e. designed to induce you to invest financial resources that may be lost forever and not be recoverable once investments are made. You are responsible for conducting your own research (DYOR) before making any investments. Read more here.