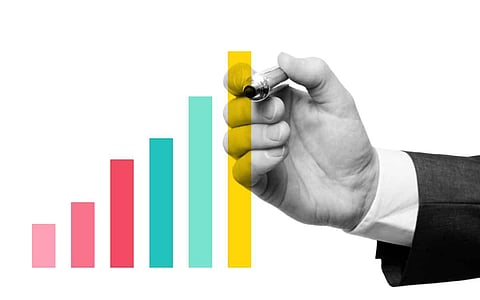
There are a lot of reasons behind organizations to implement data science and AI into their business. It can enable you to all the more likely comprehend and foresee client behavior, automate dreary manual tasks, distinguish mistakes and peculiarities quicker, assess business choices with information rather than mere instinct, get an edge over contenders, give marketing efforts more punch, check a box of investors, pull in more ability for the company, or gloat about it at gatherings. Numerous organizations face the challenge of building up a data science team without any preparation and it tends to be difficult to make sense of how to begin.
At an early stage in your journey, itʼs crucial to set up procedures to clean and change the data before you can begin sustaining it into your data science layer. For instance, letʼs state a recently launched online business startup needs to use AI and ML to all the more likely comprehend its audienceʼs purchasing behavior. The data science team can gather the data from transaction logs, process it, and clean it.
They can examine this data to then produce insights on why individuals purchase certain things and anticipate what they are probably going to purchase later on. This furnishes the company with key noteworthy insights into their customer base early-on as opposed to having to wait. Along these lines, youʼve settled on the choice to bring the jump into AI, and youʼve recognized your AI vision and the conceivable use cases to begin with. It's now time to build a data science team.
Making a data science team is the most significant part of any company but there is no correct method to do it. There are numerous designations jobs and duties that go under this synthesis and it relies upon the need of the company to choose on structuring the data science team. A part of the parameters that can be considered is the context and development phase of each organization, the requirement for setting up a data science group and more.
Augmenting data and analytics capacities in a company to a great extent relies upon how the team structure is formed. It is in this manner amazingly critical to set it the correct method to boost operational speed and efficiency. For a data science team to be made in a productive manner it initially requires to enlist the correct sort of individuals. Numerous organizations likewise favor training their previously existing workers to satisfy the hopes of the jobs that the data science team is relied upon to perform.
Particularly in the early stages of an organization, you won't have the option to foresee precisely which use cases you will experience and which aptitudes you will require. If you have a choice between a candidate with deeper and one with more extensive information, your company will most likely profit more from the one with more extensive information. Somebody who knows a tad about an expansive scope of themes (for example, hypothesis testing, data cleaning, natural language processing, image classification, anomaly detection, clustering, time series forecasting, neural networks, object-oriented programming, databases, unit testing, containerization, REST APIs, cloud computing, distributed systems or data visualization) will have a simpler time in another data science team than somebody who is a specialist at explicitly something or other yet guileless about the others.
Generalists may require more time to get to a decent answer for a project, yet will be progressively flexible, have a superior eye to recognize new business opportunities and have the option to communicate to partners with more context.
Numerous organisations are systematizing the additional role of data storytellers. These individuals go past visualization dashboards by making an unmistakable narrative from the data insights. They give context to make insights absorbable for everybody over the business.
Organisations are likewise going to arts and social disciplines to add a human dimension to data science. For instance, a behavioral psychologist is better ready to reveal the hidden explanations behind customer choices like churn, purchases, and engagement. At the point when joined with data insights, this human perspective enables companies to have a deeper comprehension of their customer base and settle on genuinely significant decisions.
Employing a data scientist isn't a simple task and utilizing ability that is as of now in-house comes as the most ideal approach to satisfy the need of hiring data scientists. Some of the duties, for example, data preparation, training models, creating user interfaces, and model deployment can be practiced with the assistance of individuals working in the IT office. The in-house IT pros can be trained on abilities, for example, realising the predictive analytics, machine learning and others to carry on the not-so-complicated tasks.
While it might have disadvantages, for example, limited exposure to machine learning techniques and data cleaning methods, or there might be a need to pay for basic procedures, for example, model training, testing or forecast, sourcing out data scientists from within the company is perhaps the most ideal ways.
Join our WhatsApp Channel to get the latest news, exclusives and videos on WhatsApp
_____________
Disclaimer: Analytics Insight does not provide financial advice or guidance. Also note that the cryptocurrencies mentioned/listed on the website could potentially be scams, i.e. designed to induce you to invest financial resources that may be lost forever and not be recoverable once investments are made. You are responsible for conducting your own research (DYOR) before making any investments. Read more here.