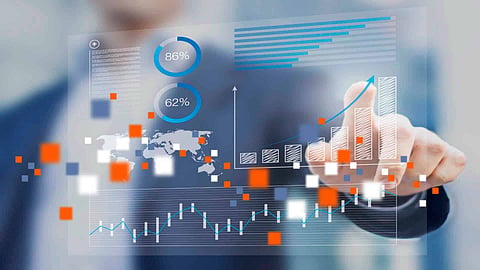
Big data has become a major component in the tech world today thanks to the actionable insights and results businesses can achieve with such multi-purpose data. However, the creation of such large datasets also calls for better understanding and having the proper tools on hand to parse through them to uncover the right information. To better comprehend big data, the fields of data science and analytics have gone from largely being relegated to academia, to instead becoming integral elements of Business Intelligence and big data analytics tools.
However, it can be confusing to differentiate between analytical capabilities and data science. Despite the two being interconnected, they provide different results and pursue different approaches. Here is how data science is different from the other five analytical approaches.
While data analysis emphasizes on correlative analysis to predict relationships between data sets or known variables to discover how a particular event can occur in the future, data science emphasizes on providing strategic actionable insights into the world where people don't know what they don't know.
Data mining is a subset of data science that refers to the process of collecting data and searching it for patterns in data. The main goal is to design algorithms that extract insights from large unstructured data sets and validate the findings by applying identified patterns to novel subsets of data. On the other hand, data science is dependent on data mining. Rather data mining can be considered the first step of data science.
For data science, the major refining tool is machine learning which is a mixture of statistics, computer science, and mathematics. Whereas, data science is a broader discipline that materializes around machine learning concepts which include interaction with existing systems like production databases, data acquisition, and data cleaning.
Statistics, an important part of data analytics, is a branch of mathematics for providing theoretical and practical support to data mining, business intelligence, and data analysis tools. While when we talk about Statistics for data science the same questions are answered using similar statistical techniques on huge unstructured data sets by using high computational resources. In a nutshell, data science neatly is woven to other subdomains like statistical learning, computational statistics, statistical computing, Bayesian statistics, and ensemble models.
Artificial Intelligence spans various knowledge domains like robotics, cognitive science, natural language processing, human-computer interaction, pattern recognition, etc. However, to note, AI is a core part of data science and very well intersects with pattern recognition and the design of intelligent systems that perform various tasks.
Join our WhatsApp Channel to get the latest news, exclusives and videos on WhatsApp
_____________
Disclaimer: Analytics Insight does not provide financial advice or guidance. Also note that the cryptocurrencies mentioned/listed on the website could potentially be scams, i.e. designed to induce you to invest financial resources that may be lost forever and not be recoverable once investments are made. You are responsible for conducting your own research (DYOR) before making any investments. Read more here.