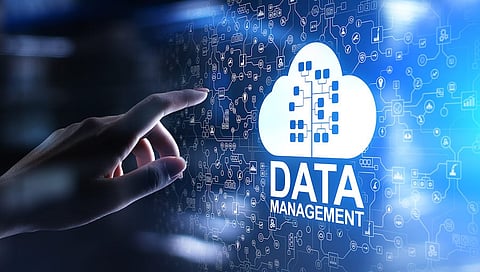
Poor quality data or incomplete data can break your business. It can lead to unwise business decisions and can even be detrimental to the future of your business.
Recent research has shown that poor data quality can cost businesses up to $14.2 million annually in terms of productivity, financial, reputational damages and missed opportunities. On an average, the average cost of bad data is about 30% higher than the revenue generated by a business. Given how business models are largely data driven, poor data quality and master data management can become a systemic problem.
Data can be said to be of good quality if it is complete, valid, up to date, unique, accurate and consistent. All of this depends on the context in which it is to be used. It is possible for the same data set to be considered good in one context and poor in another. For example, knowing how many pieces of an SKU a store has sold in the month may be enough to place a reorder for the next month but this data alone does not give any information about whether the store has made a profit or not.
This is where master data management comes in. The goal here is to combine data from different sources such as the supplier, customer, manufacturer, retailer, etc. and put it together cohesively. This helps maintain consistency across systems in cases where companies work with different SCM or CRM systems and make systems easy to integrate following acquisitions and corporate mergers. It also optimizes the customer experience and addresses their needs in the best possible way.
Improving data quality and master data management requires a holistic approach. This involves looking at the organization, the data quality assurance processes, and technology being used.
The first step towards improving data quality is to define guidelines for the same. Guidelines help prevent inconsistencies and present data in a clear, consistent manner. It also helps prevent the creation of multiple copies that may be expensive to clean up later. Setting clear guidelines also ensures that systems can talk to each other.
Developing a company culture that recognizes the importance of data for generating insights is essential for improving data quality. Roles must be clearly defined within the organization to ensure that data is collected and treated carefully. These roles may be identified on the basis of task assignment and employee capability. The typical roles that would need to be identified include:
• Data Owner: This refers to the person who will be the point of contact for data domains. This is the person who will ensure data quality, define data requirements and accessibility and give other people access to data.
• Data Steward: The steward will define the requirements and rules for handling data as well as coordinate data delivery. A steward's responsibility includes tasks such as checking for duplicate entries, etc.
• Data Manager: Data managers are usually part of the IT team. His responsibilities include implementing the ideas of the data owner, managing the technological infrastructure and protecting access to data.
• Data Users: Data users refer to everyone else who needs to have access to data from time to time.
Quality checks for data cannot be conducted once and then forgotten about. Maintaining data quality must be an ongoing project. The best way to do this is to design an iterative cycle of analyzing, cleansing and monitoring of data. The phases of a data quality cycle can be explained as:
• Define Data Quality Goals
Data quality goals or metrics should be defined as per business needs. The metrics will form a key part of the overall data quality strategy for the business. Some of the questions these goals must answer include – How is the data domain defined? How can the completeness of data be defined? What degree of completeness if required for various tasks?
• Analyze Data
Once the metrics are defined, data must be analyzed against them. Some of the questions which need to be addressed include:
• Clean Data
Data must be cleaned and standardized to meet the data quality goals. This is typically done according to individual business rules.
• Enrich Data
Data may then be enriched with other data such as socio-demographic or geographic information. Data enrichment creates a more complete data set.
• Monitor And Check Data
Data must be constantly monitored and checked to ensure that it maintains the appropriate data quality. Data monitoring can be performed automatically through software that follows pre-defined business rules.
Technology can be a great helper in improving data quality. Software that is used for data quality management should ideally be aligned with the data quality cycle and assist data users in accessing the relevant data. Software tools can help with data quality by providing:
• Data profiling
• Data quality tasks like cleansing, standardizing, parsing, de-duplicating, matching, managing hierarchy and identity resolution
• User-specific workflow support/ interfaces
• Integrating and synchronizing application models
• Data cleansing and enrichment
• Data distribution and synchronization
• Defining metrics and monitoring components
• Managing Data Lifecycle
• Reporting components
It may seem as though the organizations and technological adjustments needed to maintain high data quality are too complex but this should not make you shy away from data optimization projects. In an era of digitization, only those companies that take decisions driven by data will be able to foresee a bright future.
As a company that values data quality all you need to do is take the first step towards launching a data optimization project. The rest will evolve in due time.
Since 1985, Melissa has combined address management and contact data quality expertise with the latest innovations to help businesses do better business through clean customer contact data. Our enterprise solutions provide wide capabilities that clean, verify, dedupe and enrich contact information – addresses, names, emails and phone numbers – in real-time or batch for full spectrum data quality.