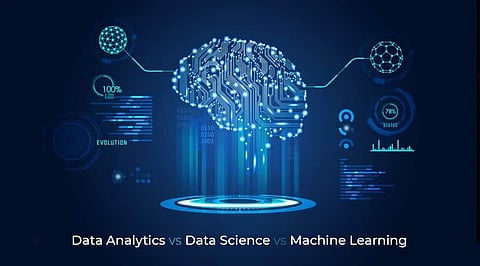
We live in a time where technology is advancing at a breakneck pace. Computing power has been rising at an exponential rate, allowing us to use it forevermore sophisticated tasks. Data analytics, Data Science and Machine Learning are three disciplines that have evolved in tandem with this tremendous expansion. But what is the difference between these 3 technologies that are so tightly linked? Let's find that out in the sections below.
Let's take a look at what each word implies, starting with a description of data analytics. Data analytics is the study of analyzing large amounts of data to identify patterns, answer questions, and make conclusions. It's a diverse and complicated area that frequently entails the use of specialized software, algorithms, and automation.
Data analytics principles may be applied to just about every sector. Data analysts are employed by a variety of organizations to assist them in making educated and data-driven choices regarding various aspects of their operations. Existing data from previous occurrences are usually analyzed, allowing existing trends to be recognized.
Advanced statistics, diagnostic analytics, data modeling, and prescriptive analytics are some of the many forms of data analytics.
Data science is a concept that covers data purification, preparation, and analysis and is used to deal with huge data. A data scientist collects data from a variety of sources and uses machine learning, data modeling, and sentiment analysis to extract useful information from it. They can give accurate forecasts and insights that may be utilized to support key business decisions since they understand data from a business perspective.
Machine learning is the process of extracting data, learning from it, and forecasting future trends for a certain topic using algorithms. Statistical analysis and predictive analysis are two types of machine learning programs that are used to identify trends and uncover hidden patterns from data.
Facebook is an excellent example of machine learning deployment. Facebook's machine learning algorithms track every user's activity on the social media site. The algorithm analyses one's interests and suggests articles and notifications on the social media feeds based on previous behavior.
Machine learning is included under data science since it is a wide phrase that encompasses a variety of fields. Regression and guided clustering are two approaches used in machine learning. The data in data science, however, may or may not come from a machine or a mechanical operation. The primary distinction between the two is that data science, as a wider phrase, encompasses not only algorithms and analytics but also the whole data processing technique.
Data science encompasses a wide range of fields, including software engineering, data engineering, data analytics, machine learning, data analytics, predictive analytics, and more. Big data is defined as the retrieval, collecting, ingestion, and transformation of enormous volumes of data. Data science is in charge of giving enormous data structure, looking for compelling patterns, and advising decision-makers on how to successfully implement changes to meet business objectives. Machine learning and data analytics are 2 of the numerous technologies and techniques that data science employs.
Data science is a broad phrase that includes data analytics, machine learning, data mining, and a variety of other related fields. Data analysts extract relevant insights from diverse data sources, whereas data scientists are supposed to anticipate the future based on historical trends. A data scientist asks questions, whereas a data analyst looks for answers to the ones that have already been asked.
If these data-driven topics of interest pique your attention, you might want to consider a similar career path. But what types of employment are available in each field? We've chosen a few samples from each category:
Data Analyst
The job of a data analyst is to process raw data and turn it into useful information. They strive to spot patterns and convey them in a clear and understandable manner.
BI Analyst
Analysts in business intelligence seek to give data insights that can help businesses make better decisions. They employ a number of approaches and techniques to enable businesses to make data-driven decisions.
Data Scientist
Data scientists must comprehend business issues and provide the finest solutions through data analysis and processing. For example, they are required to do predictive analysis and comb-over "unstructured/disorganized" data with a fine-toothed comb in order to provide actionable insights. They could also do so by spotting trends and patterns that might aid businesses in making better judgments.
Data Architect
A data architect designs data management plans so that databases may be readily linked, consolidated, and secured with the greatest security methods possible. They also make certain that the data engineers have the most up-to-date tools and systems with which to operate.
Machine learning engineer
Aspects of software engineering and data science collide in this job. Engineers that work in machine learning build algorithms and systems that assist computers in learning on their own.
NLP scientist
Natural language processing (NLP) is a technique for assisting computers in comprehending natural human speech. NLP researchers develop algorithms to aid in the comprehension of human language.
We've seen that data analytics, data science, and machine learning all have parallels and distinctions. Despite the fact that the subjects are closely related in many ways, each has its own implications, scope, and fields of specialization.
With so many career options in these fields, we're confident you'll find something that meets your objectives and complements your skills and interests. Now that you've learned the fundamentals of AI, machine learning, and analytics, it's time to get some hands-on experience with these exciting and rapidly evolving technologies.
Join our WhatsApp Channel to get the latest news, exclusives and videos on WhatsApp
_____________
Disclaimer: Analytics Insight does not provide financial advice or guidance. Also note that the cryptocurrencies mentioned/listed on the website could potentially be scams, i.e. designed to induce you to invest financial resources that may be lost forever and not be recoverable once investments are made. You are responsible for conducting your own research (DYOR) before making any investments. Read more here.