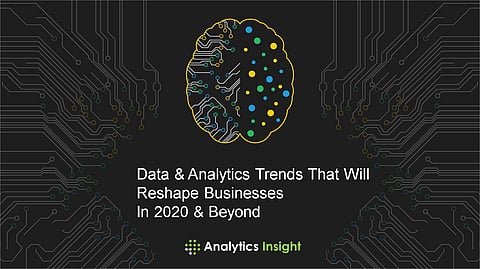
In today's digital world, businesses are flooded with data. Getting insights from the large pool of data helps businesses in making informed decisions that drive growth. New business intelligence, data and analytics technologies have surfaced that businesses need to adopt to gain competitive advantage.
Businesses should not wait for new technologies to mature. They should try them and if they feel that the impact of a particular technology is significant, they should go ahead and put that technology in their day-to-day business. Business intelligence and analytics services providers are embracing new technologies to provide their clients a competitive edge.
Following is the list of top data and analytics trends that businesses should watch out in 2020 and coming years –
Augmented analytics involves the use of technologies such as machine learning, natural language processing and artificial intelligence to augment data preparation, data analytics, and business intelligence.
The insights generated by the use of augmented analytics optimizes the process of making business decisions. The insights are available across the organization, thus decreasing dependence on data scientists and machine learning (ML) professionals, but requiring adequate data literacy for employees.
By the year 2020, augmented analytics will markedly influence purchasing of ML, data science, analytics and business intelligence solutions.
Augmented data management refers to automatic refining of data by making use of technologies such as machine learning and artificial intelligence. By utilizing the process of augmented data management, businesses are able to better organize and maintain data quality. This helps in decreasing data cleaning tasks performed by data scientists and in increasing business productivity.
Augmented data management is expected to cast a significant impact over the coming years. According to Gartner, augmentation will reduce data management manual tasks by 45% by the end of 2022, and by 2023, the need for IT specialists will be reduced by 20%.
Natural Language Processing (NLP) is a technology that is used to make computers understand the natural language of human beings. NLP is a branch of artificial intelligence (AI) and finds use in a variety of applications such as Google Translate, Microsoft Word, Grammarly, Interactive Voice Response (IVR), Google Assistant, Cortana, etc.
Natural language processing provides businesses an easy way to inquire about the data and get explanations of the generated reports. Conversational analytics is an advanced technology based on NLP. It allows a business to both ask questions and get answers verbally.
According to Gartner, by the year 2021, adoption of analytics and business intelligence solutions by the companies will rise from 35% of their employees to more than half of their employees due to natural language processing and conversational analytics. The rise will include new categories of users, especially a company's front-office staff.
Graph analytics makes use of graph structures for understanding and visualizing relationships between entities such as people, objects, etc. Graph analytics models pairwise relationships between entities in a network. It provides insight on the strength of the relationship. It also provides information regarding the direction of the relationship.
Graph analytics finds its use in various fields including detection of fraud, genome research, logistics, network impact analysis, traffic route optimization, authorization and access control, social network analysis, investment management, etc.
Over the next few years, the application of graph processing and graph databases is predicted by Gartner to grow at a rate of 100% per year. This will speed up data preparation and facilitate the use of adaptive data science.
The fields of artificial intelligence and machine learning are dominated by the open-source platforms. But, now the scenario is changing as commercial AI vendors have started to play an important role in these fields. Commercial AI vendors are now, in real sense, providing AI and ML to businesses at an affordable price. These vendors are not only offering connectors to open-source AI platforms, but also capabilities that are currently unavailable with open-source platforms such as project management, model management, etc.
According to Gartner, commercial AI will dominate the AI and ML market over the open-source platforms. By the year 2022, it is predicted that about 75% of new artificial intelligence and machine learning end-user solutions will be developed by making use of commercial AI instead of the open-source AI.
Data fabric is an architecture that helps businesses in simplifying and integrating data management across on-premises and cloud environments. Data fabric makes it easy for businesses to undergo digital transformation. Data fabric provides reusable data services for data visibility, insights, access, control and security.
Businesses are under great pressure to leverage data to attain a competitive edge, but have scarcity of time and skills. Today, data is becoming dynamic and distributed, and businesses are finding it increasingly difficult to manage the vast amount of data. Data fabric helps businesses to unleash the power of data so that they are able to better meet business demands and challenges.
Explainable AI (XAI) aims to address how autogenerated decisions of AI platforms are made. Explainable AI gets into the details of the steps that are deployed by AI systems in making decisions. It tries to understand the strengths, weaknesses and behavior of an AI model and detects biases.
Explainability is needed as black-box approaches of AI systems lack transparency and thus do not foster trust. Regulations are making it difficult for businesses to use black-box approaches as these approaches fail in explaining why a particular decision has been made by an AI system. Explainable AI makes AI systems more transparent and trustworthy. It reduces the risks posed by AI systems to reputation and regulation.
Blockchain also finds it applicability in the field of data and analytics. By utilizing the blockchain technology, businesses get assets and transactions' lineage along with transparency for participants' networks.
There are some disadvantages of using blockchain in the field of data and analytics. The data management capabilities offered by blockchain technology are limited. Businesses cannot use a system based on blockchain technology as a system of record. This implies that a huge effort is needed on the part of the businesses to integrate data, applications and processes. Except the field of cryptocurrency, the blockchain technology has not matured enough in other fields.
Continuous intelligence involves integration of real-time analytics into business operations. The current and historical business data is processed to recommend actions in response to events.
Continuous intelligence systems can now be implemented on a broader scale because of the following three factors – the advent of the cloud, development of advanced streaming software, and the growth data provided by the IoT sensors.
Gartner predicts that by the year 2022, continuous intelligence will be deployed by over 50% of major new business systems. This will help organizations to make use of real-time context data for improving business decisions.
With the rapid growth of data volumes, database management systems used by businesses are starving for memory. Huge memory and faster storage are required to handle current server loads.
Persistent memory technology offers fast and high-capacity memory and storage to enable storing, moving and processing data at unprecedented speed. Persistent memory technology aims to transform big data workloads and analytics possibilities. In the current scenario, a good number of database management system vendors are experimenting with persistent memory technology.
The new data and analytics technologies will help businesses to easily manage vast amount of data and obtain insights that will greatly enhance business efficiency and productivity.
Aditya writes on topics such as technology, IT, software, cloud computing, digital marketing, etc. He prefers a lucid writing style to get the content easily into the readers' minds. He works as a senior writer with a top web development company – Classic Informatics.
Join our WhatsApp Channel to get the latest news, exclusives and videos on WhatsApp
_____________
Disclaimer: Analytics Insight does not provide financial advice or guidance. Also note that the cryptocurrencies mentioned/listed on the website could potentially be scams, i.e. designed to induce you to invest financial resources that may be lost forever and not be recoverable once investments are made. You are responsible for conducting your own research (DYOR) before making any investments. Read more here.