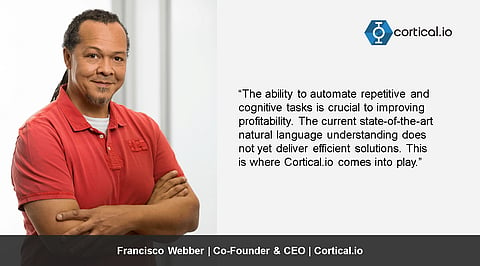
With the progress of digitalization in the economy, Cortical.io focuses on deep understanding of natural language which has become more and more important in recent years. Because of the exponential growth of text data, enterprises need to work shifting from numeric towards text information. Making sense of text information is becoming a key asset for businesses. Take an insurance company for instance: its whole business is dependent on text data since all its products are defined verbosely. All customer interactions happen in natural language. At the moment, the only way to deal with this mass of textual information is to use a human understanding of language. Whenever mass transactions need to be handled, companies tend to outsource these tasks preferably in low-cost countries, to keep costs down. But these tasks can only be performed by skilled people, like attorneys or subject-matter experts, while being extremely repetitive and boring. The ability to automate such repetitive and cognitive tasks is crucial to improving profitability. The current state-of-the-art natural language understanding does not yet deliver efficient solutions to this challenge. This is where Cortical.io comes into play as it offers an intelligent system that allows an automation of all tasks that depend on the understanding of natural language.
Cortical.io's objective is to offer an alternative to the state-of-the-art solutions, because all their attempts to solve the problems related to the understanding of natural language have proven to be either too expensive, or incapable of delivering the required performance in a business context. This is the motivation for the foundation of Cortical.io established in 2011. With the Semantic Folding theory, the company has developed its own approach towards natural language understanding, away from statistics and deep learning. In the beginning, it has struggled to convince large companies to try its technology, because its roots on a brand-new approach nobody had ever heard of.
Initially, it was difficult to trust a start-up from a small European country where kangaroos only live in zoos. But those companies that preferred well-known providers came back to Cortical.io because none of the technologies available on the market could solve their text data problem.
Today, Cortical.io implements natural language understanding solutions for Fortune 100 companies. But the company does not talk only to the big and beautiful. It also offers free tools on its website, for example, for keyword extraction. Developers from all over the world are using this free service every month which proves that there is a huge need on the market for the difference Cortical.io stands for.
Francisco Webber is the founder and CEO of Cortical.io. He has been intrigued by search engines for a long time. Probably because of the multiple experiences he had at the Vienna General Hospital when confronted with the impossibility of finding relevant patient information hidden in data silos. During Webber's early business years, he spent a lot of time searching for the ultimate search engine which would truly understand the meaning of an information request. What he discovered was that the whole field of natural language understanding was still governed by statistical modeling-based information retrieval theories. Users were coping with the limitations of keyword-based engines. In parallel, Webber had been following the research done in computational neurosciences for many years. From the several theories trying to explain how human intelligence works, the Hierarchical Temporal Memory (HTM) theory, described by Jeff Hawkins for the first time in his book On Intelligence, is the one that got the founder and CEO hooked. It was after watching a YouTube video where Hawkins talked about sparse distributed representations that Webber got convinced that he was up to something. And that this something, a new interpretation of how information is processed by the brain, could be the code breaker of all hurdles encountered by natural language understanding solutions.
Basically, Webber founded Cortical.io to test if text can be converted in a numerical representation based on Hawkins's sparse distributed representations, and if yes, whether that makes language computable.
After approximately one year of developing and testing, it became clear how powerful and efficient this new approach is. Webber published a white paper to describe the method of converting text into what he calls semantic fingerprints, and the company disclosed this discovery in a patent. After that, Webber began explaining the principles of Semantic Folding at conferences.
"The problems of language ambiguity and vocabulary mismatch are the two major challenges encountered by machines when processing natural language. Language ambiguity means that words can have many different meanings," said Webber. The term "jaguar" can refer to a car or a large cat, but also to a French fighter jet or an AMD computer architecture. Current machine learning systems only understand the meaning of words in a very superficial semantic manner. As they try to extract meaning by brute force (statistics), they need huge amounts of data and fine-tuning. Semantic Folding requires very little reference literature to train the engine, in a completely unsupervised manner. That means that the company's customers get their individually tailored semantic engine in a matter of days, rather than months. On top of that, Cortical.io's system represents every word with roughly 16,000 semantic features which allows a very precise semantic understanding. Smart text analysis can disambiguate terms in whatever sub-senses required by the use case, like "organ" will not only be "music" or "anatomy", it will be "church", "composer", "musical instrument", etc. as well.
Vocabulary mismatch is another nut that is hard to crack. Similar meanings can be expressed in many ways. For example, an investment banker who has closed a deal, can write in his email "signed contract" or "done deal": both expressions mean the same, but, as they do not use the same words, other systems would not be able to associate them. Cortical.io can. Out of the activated semantic features attached to each of these expressions, a certain percentage, say 30%, overlaps. By measuring this semantic overlap, the company's system understands that both expressions are related and will trigger whatever action is required.
The training of the engine is a crucial aspect because it traditionally binds huge investments. Cortical.io system needs little reference material to deliver accurate results. An initial investment frightening many customers before the beginning of a natural language understanding project falls out when working with the company. Moreover, once the engine is trained with, say "investmentbankerish", it can be applied to much different use cases and help several departments within the company. For instance, the company has trained a semantic engine for the compliance team and set up an email and chat monitoring system. If the marketing department needs a news filtering system, Cortical.io can easily adapt the same engine to their specific use case which is impossible with other machine learning-based systems. These systems necessitate a completely new setup, with new data and parameters. The implementation of Cortical.io's technology in an enterprise generates a competitive edge that can be determinant from a strategy point of view as it allows a fast implementation and quick first results
The company's technology is very generic. It can be applied to any text data, in any language and in any domain. That means a huge range of potential applications. For obvious reasons, Cortical.io has developed solutions that tailored to what the customers have asked for. Many of them come from the banking sector and must comply with an increasing number of regulations. One of their problems concerns the millions of contracts that need to be reviewed on a regular basis. To help them, Cortical.io has developed a tool that automatically classifies contracts or any other legal documents, based on defined entities like dates, amounts, and ratios. The company calls this Contract Intelligence. For example, a Big Four accounting firm is using it to comply with new regulations about lease account standards. Contract Intelligence solution by Cortical.io helped them to substantially reduce lease-processing costs.
The company's customers also need solutions that help them find quickly the right information wherever it is buried, in whatever way it is formulated: this is where its semantic search engine comes into action. Technical documentation is particularly tricky because of the technical jargon that constantly evolves, because this technical jargon is only used by experts, and because of the masses of new information about new product features that are added on a regular basis. Cortical.io's semantic search engine handles these problems very elegantly. For example, it has developed a handbook search tool for a German car manufacturer. The main challenge was to overcome the incompatibility of the language used by the car user, an average person, and the handbook authors, highly specialized car experts. Cortical.io has trained the engine with technical documentation but also with chats from forums about cars. In the end, customers could search "where is the donut" and the system would put you on the page "where to find the spare wheel".
According to Webber, the main concern of companies that seriously consider starting a natural language understanding project consists of the risk they are taking. This relates to huge investment in finding and preparing the training data, months in fine-tuning the parameters and testing the system, all of this for an uncertain outcome. With Cortical.io, first results can be seen within weeks of the beginning of a proof of concept. That minimizes the risk of starting a project to an acceptable level.
The other aspect is that all other approaches rely on brute force: the more the data, the better the performance of the system. Statistical approaches do not care about the quality of the material, they just add more if the results are not good enough. By trying to be a little bit smarter, the users can be so much more efficient, especially in areas where not enough training data is available. For instance, if a bank wants to put in place a system for email compliance monitoring, it is impossible to get thousands of fraudulent emails to train the engine. These domains are a challenge for mainstream machine learning approaches. With Cortical.io, businesses now have the possibility to get a working solution.
Computer systems work well with numbers and this is what makes them so powerful in the era of Big Data, but what about the text? How to convert a word into a numeric value, without losing any of its senses and contexts? What is the numeric value of the term "cat"? This is called the representational problem. How to capture the meaning of a word within a series of 0's and 1's? This is one of the central questions of natural language understanding.
Since the only system capable of fully understanding natural language is the human brain, it seems rather obvious to try mimicking the way it processes information. This is what its Semantic Folding theory does, it proposes a method of converting text into the same data format as our brain uses to transform input into action. The company calls this new way of representing words, sentences, or even whole documents, a Semantic Fingerprint. Semantic fingerprints are sparse distributed representations that encapsulate all senses and contexts of any given word, which can be visualized as a very long series of bits (128×128), where only a few bits are "on" (maximum 2%). Each of those activated bits (1's) stands for a context.
The main advantage of semantic fingerprints is that it makes text computable. Computers can easily compare the positions of the activated bits of two or more semantic fingerprints and measure their overlap. The more bits the fingerprints have in common, the more semantically close they are. This is essential because it means that the system does not look for "x equals y" anymore, it just needs a tiny overlap of bits to decide that x should be associated with y. Coming back to the previous example of "done deal" versus "signed contract", how many bits the two expressions have in common, but also where these common bits are located, so for which context they stand is countable. In other words, users can inspect semantic fingerprints down to the bit level. This makes the company's system transparent and easy to debug.
In addition, because semantic fingerprints are sparsely filled (only a few bits "on"), they can be processed at a fraction of the time other machine learning approaches need. This enables the processing of amounts of text data that are commonly considered too large to be economically processed. With Cortical.io, companies do not have to restrict their analysis to sample data anymore, they can process the whole thing. This is a major risk reduction factor in terms of compliance.
In the near future, the company plans to add the sequence learning offered by Numenta's Hierarchical Temporal Memory framework to its semantic engine. Cortical.io wants its engine to learn about the grammar, which means learning about the sequences of words. This will open a new range of applications, like machine translation and conversational dialogue systems. The company is just at the beginning of what natural language understanding can do to improve daily lives. In the coming years, be prepared to hear more and more about language intelligence!
Join our WhatsApp Channel to get the latest news, exclusives and videos on WhatsApp
_____________
Disclaimer: Analytics Insight does not provide financial advice or guidance on cryptocurrencies and stocks. Also note that the cryptocurrencies mentioned/listed on the website could potentially be scams, i.e. designed to induce you to invest financial resources that may be lost forever and not be recoverable once investments are made. This article is provided for informational purposes and does not constitute investment advice. You are responsible for conducting your own research (DYOR) before making any investments. Read more here.