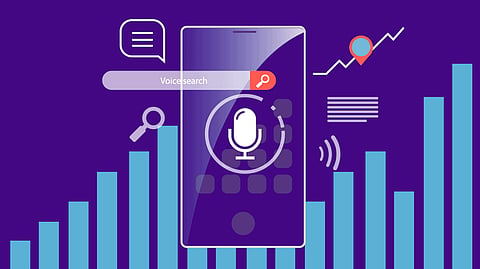
Wake words like "Alexa," "OK Google," and "Siri" and are the new technological lexicon that has revolutionized the human-machine interaction process. The growth of Artificial Intelligence (AI) and Machine Learning (ML)-enabled voice assistants like Alexa, Google Assistant, and Siri have empowered companies to obtain actionable first-party data about their customers. From a business perspective, digital voice assistants help in accessing tailored reports and insights on a real-time basis for marketing, sales forecasting, product, employee, and financial performance, and other such critical business information. These voice reports can be construed by non-technical employees for key decision-making process.
With the continual generation of phenomenal amounts of data, structured and unstructured, organizations are inundated with information from varied sources stashed away in numerous data storage systems. By separating chaff from useful data, advanced cognitive computing technologies like conversational analytics offer accurate first-party intelligence that can be leveraged by companies to understand its customer sentiments and business performance. Through AI/ML and speech recognition technology, voice assistants support users with their verbal-based queries which result in an abundance of voice-generated data. Conversational analytics helps organizations analyze the type of data and deliver personalized reporting and intelligence as it occurs.
Conversational analytics is a Natural Language Processing (NLP)-driven analytics solution that incorporates AI/ML technologies to transliterate data into speech for verbal commands directed through voice chatbots or voice assistants. This voice data is structured for critical analysis of human speech through computational linguistics and data science methods and also for future purposes. Further, deep learning algorithms have aided conversational analytics to support multi-step complex queries by continually learning through experience from large voice datasets.
Data protection and privacy regulations like GDPR and CCPA have prohibited organizations from exploiting third-party data sources, driving them to largely rely on the data generated through their own actions. In such cases, conversational analytics enables an organization to leverage market opportunities through insights from its source of large voice datasets. This actionable information helps organizations to gauge their customer behavior better, like purchase history, buying patterns, brand health, sentiment analysis, and other key metrics. From an internal usage perspective, conversational analytics – embedded in voice-enabled reporting tools – can support an organization in understanding the company's performance with respect to its financial health, marketing success, sales performance, and human resource efficiency. This level of access to important data, by means of an AI-supported conversational interface, allows CEOs and C-level executives to get time-sensitive information on demand.
For conversational analytics to be effective, it requires vast volumes of voice data, created from repeated conversations with voice chatbot or voice assistants. The overwhelming level of data produced through these interfaces not only enables conversational analytics to channel these voice data into insights but also allow it to improve its performance by creating a neural network of algorithm for deep learning. The efficacy of conversational analytics depends upon its capability to access data from multiple sources and make use of it in real-time. For this purpose, technologies like data virtualization supplement conversational analytics by enabling faster data access by offering a catalog of curated data sets. Data virtualization forms a logical data layer that connects and unifies different data types siloed across disparate sources, keeping the data in source systems. This ensures that access to all of the organization's enterprise data is available for analysis and reporting. Also, data virtualization offers integrated data as it changes and grows allowing conversational analytics to generate reports in real-time as well.
With advancements in deep learning models and NLP algorithms, conversational analytics will transcend into context-aware voice interface technology, resulting in improved human behavior analysis like emotion, mood, and sentiment analysis. As this technology develops, so will our ability to have an intelligent conversation with the voice assistant.
Increasingly, companies looking to automate complex rule-based, day-to-day activities are seeking to implement ML-powered robotic process automation with AI-fused conversational analytics. By working in tandem, an organization can benefit from enhanced customer experience as a result of voice chatbots handling the front-end task and software bots, running in the background, performing back-end, process automation tasks.
Ravi Shankar is senior vice president and Chief Marketing Officer at Denodo, a leading provider of data virtualization software. For more information visit https://www.denodo.com or https://twitter.com/denodo.