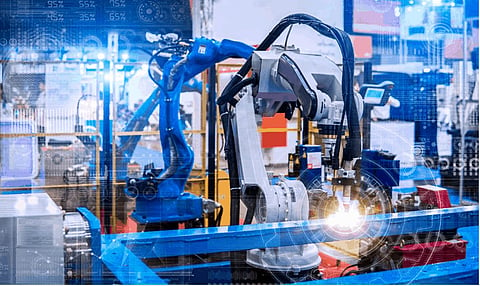
Manufacturing is one of the industries where data means a lot as the entire manufacturing processes require real-time information. The implementation of new, emerging and cost-effective technologies such as sensors, connected devices to the internet and others have generated massive hype around the future of manufacturing. These technological interventions pose a great deal of excitement across the sector, bringing new opportunities where businesses can leverage them to make good out of their investments.
However, in this whole scenario data takes the center stage that demands significant triggered control to configure them in a specific way. This is where big data comes in, which presents several promising and differentiating opportunities and challenges for industrial manufacturers. Since most manufacturing organizations have complex manufacturing processes, often with equally complex relationships across the supply chain with vendors and sub-assembly suppliers, big data offers a great solution by delivering improved capacity utilization and capital efficiency.
Despite this, the sector is still to consider some challenges while making a strategy for integrating Big Data into their operations.
Let's have a look at those challenges which need to be determined.
Leveraging technology is nothing new in manufacturing, but the evolution of new-age technologies makes it easier to perform effectively more than ever before. From automation to cloud computing, all are delivering significant capabilities to manufacturers. Though these emerging technologies generate a huge amount of data that needs to process efficiently. So, understanding the real impact of data often requires a lot of strategies, team efforts and time spend on gleaning in-depth information before real insights can be deduced. Here, all stakeholders must be well-seized of the functioning of the business. It helps develop effective reporting and decision support systems as various processes involved in manufacturing create innumerable data-points.
Often, the reason behind the failure of data models is the dearth of effective use of an organization's data capability. Businesses pour huge capital toward complex data gathering, storing and reporting systems, but these systems do not come out with effective outcomes. So, if the goal is to bring together all the pieces in the production process so that for instance quality defects can abruptly feed back to the design, enabling quick changes to everything, from the bill of materials to the automation design. Then one needs to transmit data so that every participant in the design, production, distribution, and selling process is operating off one integrated data model. This means organization-wide data management strategies are the key to effectively coordinating and focusing efforts.
The introduction of disrupting technologies, at the edges of high-volume adoption, has a much higher impact on manufacturing. Their specialization and custom work on top of novelty boost efficiency. But it is crucial to explore ways to make them work adjacent to well-established and proven systems. Within a modern factory, there are multiple levels of systems working day and night. Some major federal organizations, for instance, are still using legacy systems and application which run on an obsolete mainframe for core business operations such as high-volume data processing. Fine-tuned over the years, these systems are adapted to deliver specific functionality. But today, they require modernization, otherwise, they can be exposed to crashes anytime. It means companies need to start with a clear approach and integrate modern capabilities within the existing design and manufacturing environment efficiently.
Join our WhatsApp Channel to get the latest news, exclusives and videos on WhatsApp
_____________
Disclaimer: Analytics Insight does not provide financial advice or guidance on cryptocurrencies and stocks. Also note that the cryptocurrencies mentioned/listed on the website could potentially be scams, i.e. designed to induce you to invest financial resources that may be lost forever and not be recoverable once investments are made. This article is provided for informational purposes and does not constitute investment advice. You are responsible for conducting your own research (DYOR) before making any investments. Read more here.