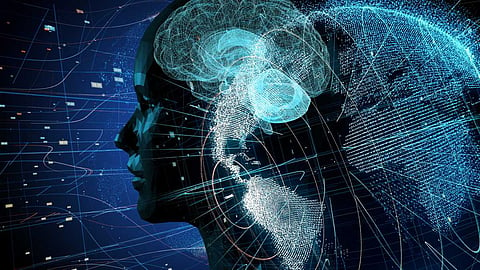
Artificial Intelligence is anticipated to shape the world's future as everything from vehicles to legitimate frameworks enfold smart innovations. Some sci-fi has anticipated that artificial intelligence would someday control over the world and turn on people, however, specialists caution there's an unquestionable progressively impending risk, called as biased AI. That is, when programs — which are theoretically neutral and without prejudice, depend on deficient algorithms or inadequate information to create unfair biases against specific individuals.
Case in point, facial recognition innovation has stood out as truly newsworthy for not being racially inclusive. About 35% of pictures for darker-skinned ladies confronted blunders on facial recognition software, as indicated in a study by Massachusetts Institute of Technology. Similarly, lighter-skinned men just confronted a mistake rate of around 1%.
Bias was likewise at the center of Google's decision to stop gender-based pronouns from its Smart Compose feature, one of its AI-empowered advancements. The potential issues of AI preference go a lot further, also, show how a portion of the inclinations held in reality can impact innovation.
Artificial intelligence programming is only as good as the data it is trained to analyze. If an organization just puts in data points around one player on the planet, at that point the subsequent program won't have the capacity to work too in different spots.
According to Eugene Tan Kheng Boon, associate professor of law at Singapore Management University, there is a risk that an AI that is trained on information from one population will perform less well when connected to information from an alternate population. For instance, there is a chance that some AI applications that are created in Europe or America will perform less well in Asia. Then, one expert noted Asian nations' increasing progress in AI implies more instances of bias issues are probably going to arise from the locale. So, one could envision, for a model, data that originates from China and India, with joined population of 2.6 billion individuals when that information turns out to be broadly accessible and utilized, there will be biases that we probably won't find in the West however might be exceptionally notable or extremely delicate in our part of the world.
An extra challenge is that inclinations can be made inside AI frameworks and afterward move toward becoming intensified as the algorithms advance.
By definition, AI algorithms are not static. Or maybe they learn and change after some time. At first, an algorithm may settle on decisions utilizing just a relatively easy group of calculations dependent on few data sources. As the framework picks up understanding, it can expand the amount and assortment of data it utilizes as input, and subject that information to progressively refined processing. This implies that an algorithm can end up being substantially more intricate than when it was at first deployed. Remarkably, these progressions are not because of human involvement to change the code, but instead to programmed alterations made by the machine to its own behavior. At times, this development can cause bias.
Take for instance programming for settling on home loan approval decisions that utilize input data from two adjacent neighborhoods, one middle income, and the other lower-income. All else being equivalent, a haphazardly chosen individual from the middle-income salary will probably have higher pay and thusly a higher borrowing limit than an arbitrarily chosen individual from the lower-income neighborhood.
Now think about what happens when this algorithm, which will develop in intricacy with the progression of time, settles on a huge number of home loan decisions over a period of years amid which the land market is rising. Loan approvals will support the occupants of the middle salary neighborhood over those in the lower-pay neighborhood. Those approvals, thus, will broaden the wealth dissimilarity between the neighborhoods, since loan beneficiaries will disproportionately benefit from rising home values, and along these lines see their future borrowing power rise much more.
According to Microsoft's Cook, stakeholders from different fields need to continually participate in dialogs of what comprises comprehensive AI, a human worry that ought not to be taken care of just by specialists in innovation. A "multi-disciplinary methodology" is required to ensure that one has the humanists working with the technologists. That way we'll get the most comprehensive AI. Human decisions are not founded on zeros and ones, but on social context and social foundation. The discussion around the right moral principles to apply to AI ought to include innovative companies, governments and common society, Cook included.
While AI can possibly bring tremendous advantages, the challenges talked about above, incorporating understanding when and in what form bias can affect the data and algorithms utilized in AI frameworks, will require consideration. Also, systems are to be articulated for surveying whether AI bias is really present in situations where it is suspected.
Join our WhatsApp Channel to get the latest news, exclusives and videos on WhatsApp
_____________
Disclaimer: Analytics Insight does not provide financial advice or guidance. Also note that the cryptocurrencies mentioned/listed on the website could potentially be scams, i.e. designed to induce you to invest financial resources that may be lost forever and not be recoverable once investments are made. You are responsible for conducting your own research (DYOR) before making any investments. Read more here.