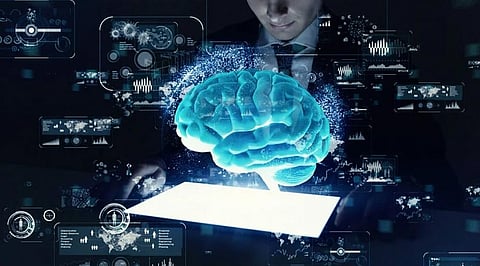
The smartness of the human mind is supposed to be a solid factor for human endurance. The human mind functions as a regulator for a lot of functions the creature requires. Robots can utilize artificial intelligence software, just like people utilize brains.
With regards to the human mind, we are inclined to commit mistakes. Nonetheless, artificial intelligence is once in a while introduced to the public as perfect. But, is artificial intelligence truly perfect? Could AI also commit errors?
Artificial intelligence is getting more noticeable in large-scale decision-making, with algorithms presently being utilized in fields, for example, healthcare services with the objective of improving the speed and accuracy of decision-making.
In any case, the research shows that people, in general, don't yet have total trust in the technology– 69% say people should screen and check each decision made by AI programming, while 61% said they believed AI should not commit any errors, in any case.
What we need to stress over is making badly designed AI and depending on it truly, so we wind up trusting smart computer systems we don't comprehend, and haven't built to be responsible or even to explain themselves.
A team of analysts from MIT has built up a deep learning algorithm expected to help AIs adapt to adversarial models, which can make an AI conduct wrong predictions and do some unacceptable actions. The algorithm created by the MIT group can help AI frameworks keep up their precision and abstain from committing mistakes when confronted with confusing data points.
Adversarial inputs resemble optical illusions for an AI system. They are inputs that confound an AI in some form. Adversarial inputs can be created with the express objective of making AI commit errors, by representing data in a style that causes the AI to believe that the contents of a model are one thing rather than another. For example, it is feasible to make an adversarial model for a computer vision system by rolling out slight improvements in pictures of cats, making the AI misclassify the pictures as computer screens.
We are progressively depending on AI solutions for precise decision-making, regardless of whether that is improving the speed and precision of medical diagnoses, or improving street security through autonomous vehicles.
As a non-living substance, individuals normally expect that AI should work perfectly. Enormous numbers of individuals need to see improved guidelines and more noteworthy accountability from AI organizations.
The MIT analysts called their methodology "Certified Adversarial Robustness for Deep Reinforcement Learning," or CARRL. CARRL is made out of a reinforcement learning network and a traditional deep neural network consolidated. Reinforcement learning utilizes the subject of "rewards" to train a model, giving the model relatively more reward the closer it comes to hitting its objective. The reinforcement learning model is utilized to prepare a Deep Q-Network, or DQN. DQNs work like conventional neural networks, however, they likewise connect input values with a degree of reward, similar to reinforcement learning systems.
Artificial intelligence doesn't contain all the probable information known to mankind out of nowhere without communication with the world. It should make assumptions and test those suspicions that make it inclined to mistakes.
In case you're getting some information about current procedures within reinforcement learning, these procedures are error-prone, simply like humans. During training of those algorithms you have a trade-off between how great it will sum up on new data and how right it can address your questions. It will remember the questions and not really learn in the latter, called overfitting.