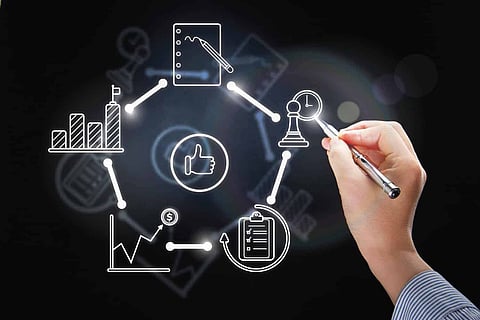
In the modern enterprise, data analytics has become more persuasive making enterprises buy the notion of what is that they need to extract from data? Even granular data will not tell the why behind the purpose.
A viable combination of qualitative and quantitative methods is what makes an analytics strategy truly apt and ready for enterprise adoption according to its unique requirements. Each analytical method has something unique to offer, says Joel Shapiro, a clinical associate professor of data analytics at Kellogg. He adds, "Quantitative analysis helps you identify broader trends, while qualitative analysis digs into human motivation, but the insights are hard to scale."
Though there are countless analytical tools and approaches that an enterprise can adopt what can it truly miss from its analytical strategy adoption?
Although much has been said about the data greatness, like it is the new oil, the raid and humongous inflows of this resource often leave the enterprises in quandary. When searching for new approaches to address a business problem, collecting and analysing data can pose to be a tougher challenge. Just for the reason, that data exists with many data points and which one is important and which one to discard can result in an enterprise dilemma!
Formulating wrong problems or chasing the wrong opportunities data analysts can have a tougher time to focus and build their data strategies to break these vast amounts of data silos.
As enterprises move to leverage the power of data analytics, finding an interesting trend or relationship in the data can prove to be tedious especially when big data is rapidly changing over time
"All predictions are based on past relationships," Shapiro says. "But the environment is constantly shifting. What is true of Amazon shoppers today might be true tomorrow, but for how long? It's hard to say. So, a business has to ask itself: 'What are all of the reasons this might not be true tomorrow, or next year?"
Data insights derived from big data's multiple data points may not prove to be of any purpose unless an enterprise focuses on what their actual business problem is and how would this data help to scale up the data models for a strategic uplift especially on large scale quantitative analysis.
"You use 'quant' to figure out what happened," Shapiro says. "You use 'qual' to figure out why. Then at some point, you need to explicitly test your hypotheses about people's motivations—to see if they scale into cost-effective solutions."
"It's always a process of triangulating what you learn in the qualitative research with the factors indicated by the data when 'qual' and 'quant' are presented as self-contained methods of analysis, they can lead to bad assumptions. Ultimately, the two should be linked in this dynamic, ongoing process of using data to solve problems." Shapiro concludes.
The modern enterprises have a massive data analytics problem at their disposal, and how they turn a crisis to an opportunity, will determine who rules the data competition.
Join our WhatsApp Channel to get the latest news, exclusives and videos on WhatsApp
_____________
Disclaimer: Analytics Insight does not provide financial advice or guidance. Also note that the cryptocurrencies mentioned/listed on the website could potentially be scams, i.e. designed to induce you to invest financial resources that may be lost forever and not be recoverable once investments are made. You are responsible for conducting your own research (DYOR) before making any investments. Read more here.