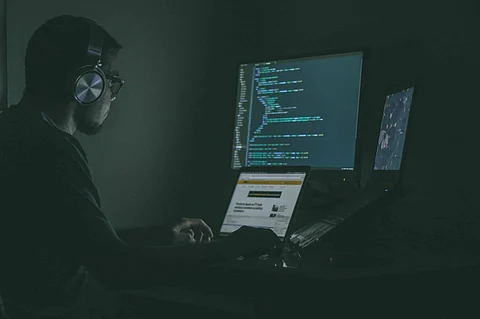
Over the past few years, there has been an incredible shift towards technology. Major companies have increasingly reoriented themselves around technologies such as artificial intelligence, machine learning and deep learning. While these technologies have garnered a lot of attention in recent years, they have been raved about as the most promising tech reinvigorating innovations across various range of business functions.
Machine learning and deep learning typically are forms of AI, but both have unique capabilities in terms of delivering services and benefits to the end-user. Undeniably, while machine learning had a vast history long before deep learning, researchers and service provider companies were making use of ML algorithms to build a variety of models to improve statistics, simplify speech and envisage risk, among other applications.
Machine learning typically simplifies a computer's ability to learn and essentially train itself in order to evolve as an effective tool to new and ever-changing data landscape. It has significantly advanced over the years by its ability to evaluate complex, sophisticated and big data.
The first ML program was invented by Arthur Samuel who coined the phrase machine learning in 1952. Upon joining IBM's Poughkeepsie Laboratory, Arthur would go on to build the first computer learning programs, which were developed to play the game of checkers. When each time checkers was played, the computer would always get better, fixing its mistakes and finding out better ways to win from that data. This automatic learning touted as one of the first examples of machine learning.
Today, the technology has become an integral part of processing data. When comparing it to deep learning, one of the main differences is that machine learning involves manual intervention in selecting which features to process, whereas deep learning does it intuitively. Experts believe that deep learning has catalyzed a revived interest in AI and stimulated the development of better tools, processes and infrastructure for all sorts of machine learning. Thanks to its unique outcomes gained in applications such as computer vision, speech recognition, natural language understanding (NLU), threat detection, etc., deep learning is increasingly becoming a red-hot buzz among businesses.
Startups can see deep learning as an advanced, sophisticated subdivision of AI with predictive competences enthused by the brain's ability to learn. The technology has the potential to identify an object in nearly milliseconds and with precision just as the human brain does.
Moreover, despite having a transformational impact of deep learning on business applications, software engineers still use traditional statistical machine learning algorithms to capture information about training data. Most ML applications within companies do not rely on neural networks and in its place capitalize on traditional machine learning models. Linear/logistic regression, random forests and boosted decision trees are the most common models. And these are the ones behind friend suggestions, ad targeting, user interest prediction, supply/demand simulation and search result ranking, among other services technology companies use.
Although deep neural networks are not utilized directly, they are indirectly driving fundamental changes in the field of machine learning. For instance, predictive capabilities of deep learning have stirred data science professionals to contemplate distinct ways of framing problems that arise in other types of machine learning.