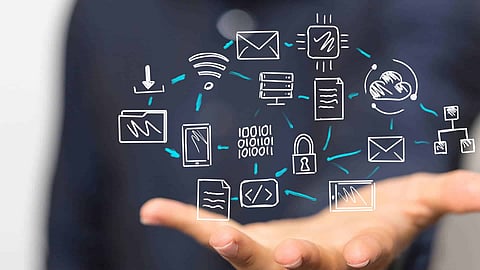
-------
"It is a capital mistake to theorize before one has data," said Sherlock Holmes. The words of literature's most famous detective ring true nearly 100 years later. Organizations today should take note, as the potential for data-driven business strategies and information products is greater than ever.
"The goal is to build a data-driven organization," says Mike Rollings, research vice president at Gartner. "And although digital business thrives on data and its analysis, we still see that data analytics only plays a supportive role when it comes to business initiatives. This has to change."
According to Gartner, "By 2020, 80% of organizations will initiate deliberate competency development in the field of data literacy, acknowledging their extreme deficiency."
Rollings adds that data and analytics leaders — especially chief data officers — should be at the forefront of that change, and shares key steps that data and analytics leaders can take to make their organization a data-driven enterprise.
Here are the 10 steps prescribed by Harvard Business Review to build an organization with data-driven culture.
Companies with strong data-driven cultures tend have top managers who set an expectation that decisions must be anchored in data — that this is normal, not novel or exceptional. They lead through example. At one retail bank, C-suite leaders together sift through the evidence from controlled market trials to decide on product launches. At a leading tech firm, senior executives spend 30 minutes at the start of meetings reading detailed summaries of proposals and their supporting facts, so that they can take evidence-based actions. These practices propagate downwards, as employees who want to be taken seriously have to communicate with senior leaders on their terms and in their language. The example set by a few at the top can catalyze substantial shifts in company-wide norms.
Leaders can exert a powerful effect on behavior by artfully choosing what to measure and what metrics they expect employees to use. Suppose a company can profit by anticipating competitors' price moves. Well, there's a metric for that: predictive accuracy through time. So a team should continuously make explicit predictions about the magnitude and direction of such moves. It should also track the quality of those predictions – they will steadily improve!
Data scientists are often sequestered within a company, with the result that they and business leaders know too little about each another. Analytics can't survive or provide value if it operates separately from the rest of a business. Those who have addressed this challenge successfully have generally done so in two ways.
By far the most common complaint we hear is that people in different parts of a business struggle to obtain even the most basic data. Curiously, this situation persists despite a spate of efforts to democratize access to data within corporations. Starved of information, analysts don't do a great deal of analysis, and it's impossible for a data-driven culture to take root, let alone flourish. Top firms use a simple strategy to break this logjam. Instead of grand — but slow — programs to reorganize all their data, they grant universal access to just a few key measures at a time.
Everyone accepts that absolute certainty is impossible. Yet most managers continue to ask their teams for answers without a corresponding measure of confidence. They're missing a trick. Requiring teams to be explicit and quantitative about their levels of uncertainty has three, powerful effects.
In analytics, promising ideas greatly outnumber practical ones. Often, it's not until firms try to put proofs of concept into production that the difference becomes clear. One large insurer held an internal hackathon and crowned its winner — an elegant improvement of an online process — only to scrap the idea because it seemed to require costly changes to underlying systems. Snuffing out good ideas in this way can be demoralizing for organizations. A better approach is to engineer proofs of concept where a core part of the concept is its viability in production. One good way is to start to build something that is industrial grade but trivially simple, and later ratchet up the level of sophistication.
Many companies invest in "big bang" training efforts, only for employees to rapidly forget what they've learned if they haven't put it to use right away. So while basic skills, such as coding, should be part of fundamental training, it is more effective to train staff in specialized analytical concepts and tooling just before these are needed — say, for a proof of concept. One retailer waited until shortly before a first market trial before it trained its support analysts in the finer points of experimental design. The knowledge stuck, and once-foreign concepts, such as statistical confidence, are now part of the analysts' vernacular.
It's easy to forget the potential role of data fluency in making employees happier. But empowering employees to wrangle data themselves can do this, as it enables them to follow the advice in a memorably titled book on programming: Automate the Boring Stuff with Python. If the idea of learning new skills to better handle data is presented in the abstract, few employees will get excited enough to persevere and revamp their work. But if the immediate goals directly benefit them — by saving time, helping avoid rework, or fetching frequently-needed information — then a chore becomes a choice. Years ago, the analytics team at a leading insurer taught itself the fundamentals of cloud computing simply so they could experiment with new models on large datasets without waiting for the IT department to catch up with their needs. That experience proved foundational when, at last, IT remade the firm's technical infrastructure. When the time came to sketch out the platform requirements for advanced analytics, the team could do more than describe an answer. They could demonstrate a working solution.
Many companies that depend on data harbor different "data tribes." Each may have its own preferred sources of information, bespoke metrics, and favorite programming languages. Across an organization, this can be a disaster. Companies can waste countless hours trying to reconcile subtly different versions of a metric that should be universal. Inconsistencies in how modelers do their work takes a toll too. If coding standards and languages vary across a business, every move by analytical talent entails retraining, making it hard for them to circulate. It can also be prohibitively cumbersome to share ideas internally if they always require translation. Companies should instead pick canonical metrics and programming languages. One leading global bank did this, by insisting that its new hires in investment banking and asset management knew how to code in Python.
For most analytical problems, there's rarely a single, correct approach. Instead, data scientists must make choices with different tradeoffs. So it's a good idea to ask teams how they approached a problem, what alternatives they considered, what they understood the tradeoffs to be, and why they chose one approach over another. Doing this as a matter of course gives teams a deeper understanding of the approaches and often prompts them to consider a wider set of alternatives or to rethink fundamental assumptions. One global financial services company at first assumed that a fairly conventional machine-learning model to spot fraud couldn't run quickly enough to be used in production. But it later realized the model could be made blazingly fast with a few simple tweaks. When the company started to utilize the model, it achieved astonishing improvements in accurately identifying fraud.
Join our WhatsApp Channel to get the latest news, exclusives and videos on WhatsApp
_____________
Disclaimer: Analytics Insight does not provide financial advice or guidance. Also note that the cryptocurrencies mentioned/listed on the website could potentially be scams, i.e. designed to induce you to invest financial resources that may be lost forever and not be recoverable once investments are made. You are responsible for conducting your own research (DYOR) before making any investments. Read more here.