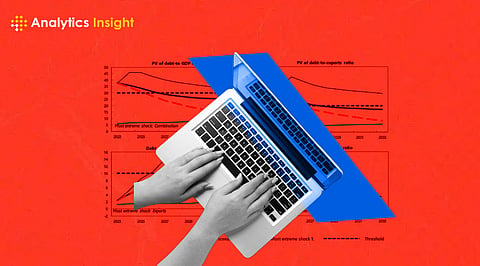
The challenges in Big Data are the natural execution obstacles. These require prompt consideration and must be handled because if not handled, the innovation may be disappointed, which can moreover lead to some repulsive results. Big data challenges incorporate storing and analyzing extensive and fast-growing data.
To understand the challenges of big data, you must understand what the word "Big Data" implies. When we hear "Big Data," we might wonder how it differs from the more common "information." The term "information" alludes to any natural character or image that can be recorded on media or transmitted through electronic signals by a computer. Crude information, however, is only possible once it is handled somehow.
The most frequent challenge in significant data endeavors is the detachment of information sets from outside sources. Sharing data can cause significant challenges. It incorporates the requirement for inter and intra-organizational lawful documents.
Accessing information from open stores leads to different difficulties. Information needs to be accessible in a precise, total, and timely way. If information in the company's data framework is to be utilized to make exact choices in time, then it becomes essential for information to be accessible in this manner.
Privacy and security are other imperative challenges with Big Data. This challenge incorporates delicate, conceptual, specialized, and legitimate significance. Due to the expansive amounts of information available today, most organizations need to be more capable of maintaining regular checks. In any case, it must be essential to perform security checks and observations in real-time since they are most beneficial.
Some data about an individual, when combined with huge outside information, may lead to a few realities about an individual that may be secretive, and he might not need the owner to know this data about that person. Some organizations collect data on individuals in order to add value to their trade. This is done by introducing experiences into their lives that they’re uninformed of.
There are a few tremendous analytical challenges with big data. These raise some primary questions, like how to deal with an issue if the information volume gets too large, how to discover the vital information points, or how to utilize information to the best advantage.
The vast amount of information on which this sort of examination is to be done can be organized (organized information), semi-structured (Semi-organized information), or unstructured (unorganized information). There are two strategies for making choices: either incorporating enormous information volumes in the analysis or deciding upfront which enormous information is relevant.
There are a few major challenges with big data, including technical challenges. Let’s learn about them.
When an expansive amount of information is collected and stored, it comes at a cost. Enormous companies, trade pioneers, and IT pioneers continuously need massive information storage. For superior results and conclusions, vast information, rather than unessential information, centers on quality information storage.
This encourages the emergence of an address for guaranteeing that information is significant, how much information would be sufficient for choice-making, and whether the stored information is precise or not. It is one of the technical challenges in big data.
Fault tolerance is another specialized challenge, and fault resistance computing is complicated, including complicated algorithms. Nowadays, a few of the new advances, like cloud computing and big data, continuously expect that whenever disappointment happens, the damage done must be within the satisfactory limit. That is, the entire task must not start from scratch.
Big data ventures can develop and advance quickly. The adaptability issue of Huge Information has led to cloud computing. It leads to different challenges, such as running and executing different occupations so that the objective of each workload can be accomplished cost-effectively. It also requires proficiently managing the framework disappointments. This leads to an enormous address again about what sorts of capacity gadgets are to be used.
Summertime is here, and so is the time to skill up! More than 5,000 learners have completed their journey from the essentials of DSA to advanced-level advancement programs such as Full-Stack, Backend Advancement, and Data Science.
As the number of Web clients grew throughout the final decade, Google was challenged with how to store so much client information on its conventional servers. With thousands of search questions raised each moment, the recovery handle was consuming hundreds of megabytes and billions of CPU cycles. Google required an extensive, conveyed, highly fault-tolerant record framework to store and prepare the inquiries. In response, Google created the Google File System (GFS).
GFS engineering comprises one master and numerous chunk servers or slave machines. The master machine contains metadata, and the chunk servers/slave machines store information in a conveyed design. Whenever a client on an API needs to study the information, the client contacts the ace, which reacts with the metadata data. The client employs this metadata data to send a read/write request to the slave machines to create a response.
1. What is Big Data?
The definition of big data is data that contains greater variety, arriving in increasing volumes and with more velocity. This is also known as the three “Vs.” Put simply, big data is more extensive, more complex data sets, especially from new data sources.
2. What are the main challenges associated with Big Data?
Common challenges in Big Data management include data privacy, security, storage, data integration, data quality, and data accessibility. The only significant challenge is data security. Big Data management only faces challenges when the volume of data is uncontrollable.
3. How is data storage a challenge in Big Data?
One of the main challenges of big data storage is the scalability of the infrastructure. As the data volume increases, the storage infrastructure must be flexible to scale up and down to accommodate the changing needs of the organization.
4. Why is data processing a significant challenge in Big Data?
Available data is growing exponentially, making data processing a challenge for organizations. One processing option is batch processing, which looks at large data blocks over time. Batch processing is proper when there is a longer turnaround time between collecting and analyzing data.
5. How does data quality impact Big Data analytics?
Data quality is a crucial factor for any data analysis project, as it affects the validity, reliability, and usability of the results. Poor data quality can lead to inaccurate, misleading, or biased conclusions and undermine the value of your data insights.
Join our WhatsApp Channel to get the latest news, exclusives and videos on WhatsApp
_____________
Disclaimer: Analytics Insight does not provide financial advice or guidance. Also note that the cryptocurrencies mentioned/listed on the website could potentially be scams, i.e. designed to induce you to invest financial resources that may be lost forever and not be recoverable once investments are made. You are responsible for conducting your own research (DYOR) before making any investments. Read more here.