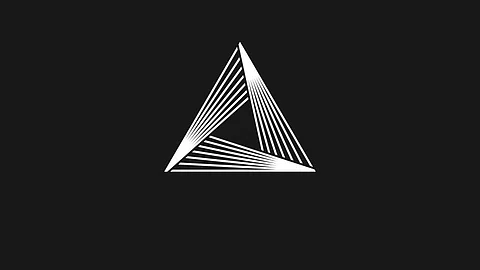
Practically any article you read about how automation will influence our future can be divided into one of two stories. The first is that it will lead to a superior future, as it always had since the industrial revolution. Obviously, a few people will lose their jobs, yet as history appears, new openings will be made. Furthermore, not just new openings, however better jobs. The other story is that this time is extraordinary. The robots are ending up increasingly smart and fit. Also, the number of jobs and enterprises they'll crush will far surpass the number of occupations they make. Obviously, it's difficult to tell which of the two stories will turn into a reality. What we can tell is that these stories share comparative initiation: an ever-increasing number of parts of our jobs and lives are being automated.
Data scientists help organizations figure out how to extricate valuable insights from an ocean of data to help examine and streamline their companies based on the discoveries. Data scientists focus on analyzing data, asking information-driven questions and applying statistics and mathematics so as to discover important outcomes.
Data scientists have foundations in advanced math and statistics, advanced analytics and progressively in AI and ML. For organizations who are hoping to run an AI project, having a data scientist in the group is advantageous to benefit from their data, modify algorithms and say something regarding data-driven choices.
In any case, extracting data from big data sets can be costly. To actualize any kind of big data project, an organization must form a data infrastructure first. Consider it various pieces of innovation that can run every one of the tools a data scientist needs. The issue is that for a long time building such a framework resembled assembling a vehicle just from parts. Conceivable, yet you required individuals with exceptionally specific skills and it took a great deal of money and time. Luckily, this is evolving. What we have found in previous couple of years is the presence of platforms that automate this procedure. Take for instance different cloud-based platforms that make it a lot simpler to create and maintain big data infrastructures, from my very own group to others in the market like Amazon Web Services (AWS), Google, Microsoft, and Anaconda.
Automated big data platforms are just a part of the story. Despite the fact that they empower us to set up and keep up data frameworks all the more effectively, regardless someone needs to compose lines of code to clean the data and test with ML models. This procedure can be very tedious and unnecessarily perplexing.
For organizations with huge datasets and complex use cases or a large-scale approach, all things considered, an organization will absolutely require more than one data scientist so as to complete the project in a sensible amount of time. However, if an organization is anticipating seeking after numerous smaller endeavors, it tends to be similarly as valuable to have just a couple of data scientists per team to work close by different individuals from a team. Contingent upon the need, the data scientist may most likely work intimately with developers so as to arrive at an ultimate objective instead of requiring everybody in the group to have that particular set of abilities. Data scientists can likewise work nearby and train individuals from the existing group to perform as native data scientists.
As the pertinence of artificial intelligence keeps on developing and the talent crunch around data scientists develops with it, numerous organizations are thinking about whether they can abandon one. It very well may be hard to locate decent data scientists, and their compensations are often steep. It is conceivable to move towards an AI future without having a data scientist ready, however, it truly relies upon the projects you're hoping to run.
There's still a requirement for a person who can build and decipher models. The issue is that these skills are rare. What's more, they're likewise costly to pay for. Perhaps the greatest obstacle organizations face when trying to construct advanced analytics projects is the absence of skilled data scientists. This is the place where the next point becomes an integral factor: automated machine learning. Some of these analytics organizations have gone above and beyond and began integrating automated machine learning frameworks into their platforms. The sort of systems wherewith simply some negligible intercession you can drop your information in and get accumulation models out.
The greatest advantage of these frameworks bring to the table is that they make predictive analytics open to a lot more extensive group of audience. They can be inconceivably amazing assets in helping non-technical employees to tackle simpler forecast issues like customer churn.
As the talent gap for data scientists keeps on increasing, there is no uncertainty that we will see new tools made out of a need that permits non-technical and business employees to run, test and analyze information. Business supervisors will start to learn fundamental data science to help oversee and move AI tasks forward. Conventional data scientists will even now be expected to run a complex analysis of information, however, generally fundamental analysis will move to resident data scientists' roles due to progressively easy-to-use tools.