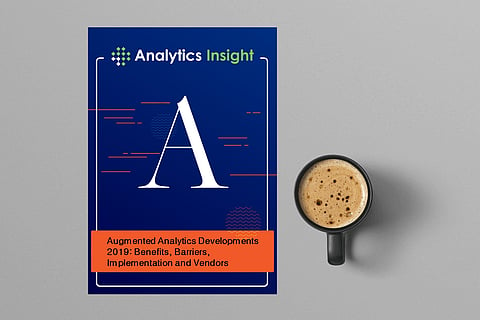
In this high paced competitive world, sticking to static reporting and dashboards will not pay better and possibly cause insights limitations. Companies need to know the variables and correlation that matter for their businesses and this is where augmented analytics comes into play. According to a recent Gartner report, "Augmented analytics leverages ML/AI techniques to transform how analytics content is developed, consumed and shared. It automates data preparation, insight discovery and sharing. It also automates data science and ML model development, management and deployment."
Through ML and AI, businesses can help prepare the data for analysis, analyze the data, and explain the insights. Augmented analytics companies are attempting to disrupt the analytics industry and address the shortage of data science talent by making data scientists and less-skilled people more productive.
To know more, let's explore what benefits Augmented Analytics offered in the past year.
The year 2019 observed that augmented analytics is intended for pacing up the data analytics to harness more value from the provided data. The technology is already causing disruption to the interpretation, creation and sharing of analytics. Here are some of the key benefits of augmented analytics:
Employing augmented analytics, data preparation techniques and ML algorithms, businesses will be able to clean the data in less time. Also, by automating iterative steps in any process, the entire data preparation and discovery time can be shortened by approximately 50-80 percent.
Post data preparation, augmented analytics detects signals that impact business models that data scientists with less business knowledge may not be adept at finding. Using this technology, data scientists don't have to determine the appropriate algorithm to use or write code to get results. Moreover, augmented analytics democratizes the insights and makes it easy for business users to extract complex insights and saving them significant time in doing so.
After the signals have been determined, next comes communicating results with executives. The traditional ways to carry out this task used to put additional burdens on executives who typically don't have time to log into a platform and do their own data interpretation, whereas augmented analytics tools will read the chart or report and translate the information into natural language statements. Such level of actionable insights enables data-driven decision making and increases adoption across the entire organization.
Apart from all the benefits, however, augmented analytics doesn't come without obstacles. Here are some significant barriers observed by Search Business Analytics in 2019, that come along the way while embracing augmented analytics.
Augmented data discovery tools have their own obstacles. PubNub's Blum said, "Processing data at scale can easily run up massive costs. Cognitive services, like IBM Watson and Amazon Comprehend, are incredibly powerful and easy to integrate through their APIs, but charging by each execution of the service can easily run up a giant monthly . It's important to not run the computation repeatedly on every piece of data, but only on the databases of unstructured data to be analyzed."
Moreover, it is important for managers implementing augmented data discovery, to think and understand the relevance of building trust: in the resulting insights and trust that employees won't lose their jobs. According to John Hagerty, vice president of product management for business analytics at Oracle, "It's critical that organizations be prepared to work with business teams to generate that trust by proving out the choices made via algorithms, so they embrace — not doubt — the recommendations the system makes."
It would be wise to address the concerns that current jobs will change once augmented analytics projects are off the ground or else employees may find creative ways to slow adoption of the tools, or worse. Hagerty further added, "Systems automatically doing the work that humans now do is frightening to some people." Therefore, the responsibility lies with managers who need to find ways that these tools assist people in their decision-making, rather than replace them. Now is the time to focus on how these tools can provide employees with more time for taking the best actions to optimize performance.
Also, augmented data discovery can risks of weaving biases into the data models generated by citizen data scientists and enterprise data can include collections of data tables with complex relationships. According to Ryohei Fujimaki, CEO and founder of dotData, a data science automation platform, "Accurately transforming these into a single table for generating insight often requires highly manual and time-consuming efforts from domain experts and data scientists." This expertise is often required to narrow down hypotheses that are based on domain expertise and informed bias honed by years of experience. Some augmented data discovery tools are starting to use artificial intelligence to help simplify the expertise required to create feature tables correlated with business outcomes. Such developments can be further enhanced with capabilities that generate natural language explanations and visual blueprints for data model features that are more understandable to business users.
Apart from biases, enabling the wrong users can also be a challenge in the successful adoption of augmented analytics. The huge promise of abstracting insights away from the underlying technical aspects in order to enable users to elicit deep, actionable insights is also a danger. Chorus.ai's Breakstone said, "In the wrong hands, such high-power tools can be exploited either maliciously or through negligence to support pseudo-insights and harmful recommendations."
Besides, setting a right data governance plan is also a barrier in some cases. It's important to include a governance strategy around the data used in augmented data discovery. As correctly put up by Marge Breya, chief marketing officer at MicroStrategy, "If you create insights from unreliable data, you can't trust the results." Taking humans out of the data preparation and modeling processes can also be a risk to effective decision-making which may subsequently result in fostering biases because of the person creating the model.
Moreover, Breya recommends that managers incorporate a consistent semantic layer for tying together disparate data sources. Furthermore, the data should be augmented with additional telemetry, such as user and system information. She said, "If done well, augmented data discovery has the potential to create direct pathways to the insights every employee needs to make real-time, data-driven decisions that boost productivity."
The industry-demand for better business prospects and productivity in 2019 has translated into tremendous growth for augmented analytics. According to a report, the global augmented analytics market is on track to grow 24 percent annually through 2023, when it is expected to become a US$13 billion market.
Let's hear from experts who have cited the real-world augmented analytics examples which somehow tend to fall into following major categories of analytical enhancement.
Krzysztof Surowiecki, managing partner at data analytics company Hexe Data said, "Augmented and smart analytics reduces all the painstaking processes that data analysts need to do every time they receive new data sets to work with."
Surowiecki further explained that data analyst traditionally spend 80 percent of their time "cleaning" data through the extract, transform and load (ETL) process where, augmented analytics can be used to automate such process so that the people interacting with the data flip that ratio and spend more time thinking about the implications of the data, deriving insights from it and proposing recommendations to help the business.
According to Ben Gaines, director of product management for Adobe Analytics Cloud, "AI-enabled NLP really comes in handy here." He further added, "The ability to type or speak a question in one's own natural language and have a tool 'translate' that speech into a query that produces a meaningful result means that people who don't necessarily understand how to apply a filter or add a dimension to a report can now begin to understand their business on a data-informed level."
According to Surowiecki, "Companies will no longer require candidates with experience in statistics and mathematics background [to do BI]. They would be able to hire less-technical people who understand their business, can conduct insights and offer recommendations based on the delivered data. That would be a very interesting change that we can observe in the nearest future."
As analysed by Gaines, when well-employed, AI applications should lower the barrier of adoption to help non-technical business users feel data-oriented by offering them comfortable ways of looking at analysis created for them. This will further help them derive insights and start delving deeper by asking the less apparent next question of data analysis.
Gaines further added, "Static dashboards often aren't enough to answer deep questions. Where AI and ML can contribute is in knowing what that next question should be, ensuring that brands are leveraging data in the right way and making that next question easier for everyone — from the CEO to the front-line marketing manager."
According to him, "suddenly, analytics capabilities are a lot more than just pretty bar graphs and pie charts — they become a two-way conversation where the business can truly ask questions and get answers."
Heena Purohit, senior product manager for IBM Watson IoT said, at many industrial firms, the aging workforce is starting to become a big concern. She explained that in the oil and gas industry, a big chunk of their experienced workforce is expected to retire in the next five to 10 years. The fear is that the departure of these retiring gurus is going to put companies under severe risk of brain drain.
According to her views, "Companies are now looking for innovative ways to retain their tribal knowledge and expertise, and augmented intelligence is helping them in this pursuit."
While improving the automation of insights, the idea is to use repeatable machine learning tools to automate certain types of analyses that would take a data science team months to build on their own.
Gaines pointed to one B2B services company his firm was working with that had a major executive considering decreasing budget for paid search due to poor conversions from the investment.
He further explained, "The marketing team created a segment of visitors who came to the site via paid search and compared them to all other visitors using Segment IQ. They discovered that although these visitors were not as likely to convert directly, they were three times more likely to upsell on a previously purchased service. The marketing team was then able to focus their budget on just the upsell-related keywords and saw a 56% increase in service upsells."
As the market innovates, analytics vendors are upgrading their traditional solutions or perhaps replacing them with augmented analytics tools to expand their product portfolios and services by offering competitive products and solutions. Here are the top augmented analytics service providers analysed by Analytics Insight.
Microsoft Power BI offers a host of services that incorporates interactive dashboards, data preparation, data discovery, and augmented analytics from a single product. Power BI Report Server allows users to share reports but lacks some of the machine-learning capabilities found in Power BI SaaS.
Qlik offers a host of services that include agile analytics, BI solutions and governed data discovery via its lead product Qlik Sense. QlikView continues to be enhanced to make up a larger portion of the company's installed customer base, while Qlik Sense now accounts for more than 50 percent of license revenue.
Tableau empowers its users with drag and drop analytics without the need to know how to code to present their findings on an interactive dashboard. Tableau offers three primary products that are Tableau Desktop, Tableau Server and Tableau Online (on the cloud). Tableau has always been committed to its users giving the power to explore and find insights in data.
IBM is represented on this list with two products this year which include IBM Cognos Analytics and IBM Watson Analytics. Cognos Analytics version 11 and higher represents the rebranding of the Cognos Business Intelligence product (version 10.2.2 and earlier), to signify the integration of production reporting capabilities with self-service dashboards and ad hoc analysis within one modern analytics and BI platform Cognos Analytics is available both on-premises or as a hosted solution on the IBM cloud.
Salesforce rebranded its analytics portfolio from Einstein Analytics to Salesforce Analytics in November 2017 and the platform includes basic operational reports and dashboards for Salesforce data, Einstein Analytics-based packaged applications, Einstein Discovery and the Einstein Analytics Platform (formerly Wave). The Einstein Analytics Platform is for creating dashboards, point-and-click interactive visualizations, and analysis with integrated self-service data preparation for Salesforce and non-Salesforce data. The Einstein Discovery is an augmented analytics platform leveraging machine learning to generate smart suggestions for data preparation, to automatically find visualization to document important insights in a story for every user, without requiring them to build models or write algorithms.
SAP delivers a broad range of analytics and BI capabilities which are useful for both large IT-managed enterprises and business-user-driven modern deployments. SAP Analytics Cloud's machine learning technology augments the analytics process, helping users go from insight to action in a fraction of the time. Automated technology enables them to avoid agenda-driven and biased decision making by revealing the story of what drives the business.
SAS competes in the analytics and BI market with additional imprints in the data science and machine learning market. SAS Visual Analytics is the primary product offering that brings interactive discovery and dashboards in addition to reporting and scheduling for mainstream business users. The advanced analytics capabilities within SAS Visual Analytics are geared toward citizen data scientists, who don't need to be experts in the coding of specialist statistical skills.
TIBCO Spotfire was an early visual-based data discovery disruptor that transformed the market from traditional reporting to modern BI and analytics. Spotfire offers extensive capabilities for analytics dashboards which include interactive visualization and data preparation in a single design tool and workflow while offering flexible processing options either in-memory or in-database integration.
Sisense offers an integrated analytics and BI platform encapsulating the entire workflow from data collection to storage developed on a self-contained in-memory columnar database. These facilities are supported with visual data exploration, in-chip processing, dashboards and recently added embedded advanced analytics features. OEM and embedded analytics continue to be the core area accounting for more than half of the company's revenue.
MicroStrategy Version 10 combines self-service data preparation & exploration with native big data connectivity over enterprise analytics and BI. MicroStrategy offers a fully interactive visual exploration experience integrating the best-in-class enterprise reporting and mobile capabilities delivered in a single, integrated platform and workflow.
Join our WhatsApp Channel to get the latest news, exclusives and videos on WhatsApp
_____________
Disclaimer: Analytics Insight does not provide financial advice or guidance. Also note that the cryptocurrencies mentioned/listed on the website could potentially be scams, i.e. designed to induce you to invest financial resources that may be lost forever and not be recoverable once investments are made. You are responsible for conducting your own research (DYOR) before making any investments. Read more here.