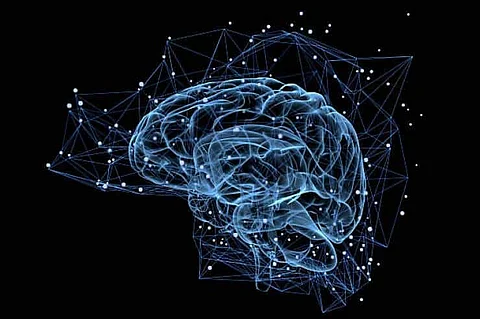
The Machine learning model classifies lithic assemblages of Eastern Africa to identify major incidents of history.
Archaic incidents have shaped the existence of human civilization. It tells about the evolution of humans over centuries. Understanding these incidents helps us to have a peek in the past. Moreover, it helps in analyzing the various processes that led to the development of humans. Archaeology is the science that highlights the incidents before human civilization shaped the world. For a long time, archaeologists, historians, researchers and scientists have tried to determine the transition between the middle stone age and later stone age. Explicitly they are trying to identify incidents that differentiated both these ages.
Undoubtedly, these time periods have significantly shaped human history. This was also the period which carved the artistic skills and sense of protection amongst humans. For example, the carving of weapons and tools enabled humans to have a heightened response against dangers.
So, knowing about these incidents plays a pivotal role to comprehend the formulation and development of human civilization. Archaeologists and researchers have resorted to using traditional methods such as fossils to classify the middle Stone Age and later Stone Age. However, as such methods failed to produce fruitful results, researchers are using artificial intelligence to classify assemblages based on MSA and LSA. In archaeology, the assemblage is defined as the aggregate of artefacts and other remains found on a site that is considered as material evidence in support of a theory concerning the culture or cultures inhabiting it.
According to a new research, machine learning is identified as the potential tool which can aid researchers in identifying incidents that differentiated MSA from LSA. A research paper titled "Neural Networks differentiate between Middle and Later Stone Age lithic Assemblages in Eastern Africa" states that trained neural networks ensembles classified over 94% of assemblages correctly, and identified 7 key technologies that significantly distinguish between assemblage classes. These results clarify both temporal changes within the MSA and differences between MSA and LSA assemblages in Eastern Africa.
The researchers state that the onset of transition from middle Stone Age to later Stone Age happened over 67000 years ago. The transition is often characterized archaeologically by the decrease in prepared-core technologies and the production of retouched points and by concomitant increases in microliths, prismatic blade and bipolar reduction method. In the Stone Age, bipolar reduction method is defined as the carving the stones or rocks into tools or weapons by removing some parts.
During this transition period, the frequencies of ochre use and bead produced had significantly increased. Various innovations which are employed to characterize LSA indicate "time-transgressive process that differed in both chronology and technology between regions.
Artificial Neural Networks are the computer models which mimics the neural network of the human brain. Like human neural networks, their processing power arises from multiple units. In order to classify different assemblage between MSA and LSA, researchers have used an artificial neural network. The researchers cite that the combination of multiple artificial neurons allows Multi-Layer Perceptrons (MLPs) to accurately represent and learn complex nonlinear relations between classes. It implies that change in one entity or class does not correspond with the change in other entity or class.
The back-propagation algorithm used aids in a fast, efficient method for training MLPs. The algorithm looks for the minimum value of the error function in weight space using a technique called delta rule and gradient descent. This method is known as the differences between the actual and the desired classifications and is fed back through the network in order to update the biases of individual neurons and the weights that connect them.
The researchers have used two separate sets of analysis for dividing the collected data into either two or three classes. This allowed them to simultaneously examine overall differences between the LSA and MSA and changes within the MSA itself. Almost 1,000 feed-forward ANNs were trained to distinguish between the two respective ages, and a further 1,000 networks to distinguish between LSA and MSA assemblages. The ANNs employed each have three layers: an input layer of 16 nodes (one for each technology), a hidden layer of 10 nodes, and an output layer of two or three nodes.
The researchers have majorly trained the networks using a Bayesian Regularization (BR) algorithm, which is observed to be a more robust back-propagation algorithm. BR is particularly useful for relatively small samples due to its internal regularization procedure. The researchers observe that BR networks retain for training only the non-trivial links between nodes, forming a more parsimonious network than would be used in a fully connected back-propagation network. Moreover, researchers have also used the Levenberg-Marquardt optimization to update the weight and bias values. Also known as 'damped least squares', this an algorithm tends to produce more generalized results. They monitored the performance during training through the sum of squared errors between the true and estimated classifications.
The researchers found that backed pieces appear in high proportions in both LSA (77%) and MIS3&4 MSA (71%) assemblages, and are considerably less prevalent in MIS5 MSA assemblages (23%). MIS3, MIS4 and MIS 5 stand for Marine Isotope 3,4 and 5 which reflects the changes in stone age temperature. Additionally, the ANN analysis revealed blade technology as an indicator of the LSA, with 94% of this technology usage in LSA. Moreover, scrapers are present in 77% of all assemblages in the dataset, with 79% presence in MSA, and 94% in LSA assemblages respectively.