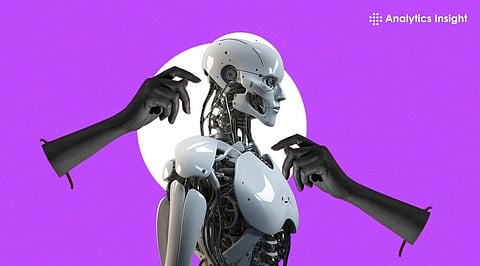
The introduction of Artificial Intelligence (AI) has changed the way we interact with data, creating new prospects for creativity and efficiency. With the emergence of DIY AI, people, and organizations may now use existing information to train AI models that are suited to their requirements. This article looks into the training techniques with databases, ensuring that you can fully realize the benefits of this powerful technology.
The data used to train an AI model serves as its basis. Before getting into training techniques, make sure you properly grasp your database. This includes determining the sort of data you have, its organization, and the insights you hope to get from it. Data can be either organized, like spreadsheet entries, or unstructured, like text or graphics. The nature of your data will determine the training method and type of AI model that is most suited to your goal.
Once you've grasped the data, the following stage is preparation. This comprises data cleansing, which removes inconsistencies or extraneous information, as well as data annotation, which labels data points to offer context to the artificial intelligence. For example, in image recognition tasks, you would label photographs so that the AI might learn to recognize the items inside them.
Choosing the correct AI model is similar to picking the right tool for the job. There are several models available, each with unique strengths and applications for different sorts of data and jobs. Neural networks, for example, are effective at recognizing complicated patterns, but decision trees may be more suitable for basic categorization tasks.
Feeding data to an AI model and letting it learn from it is the process of training it. This approach can be supervised, in which the model learns from labeled data, or unsupervised, in which the model detects patterns without explicit instruction. Tuning hyperparameters, which control the model's learning process, is also part of the training phase.
Following training, it is critical to assess your model's performance. This is accomplished by testing it with fresh, previously unknown data to evaluate how effectively it generalizes the information it has gathered. The evaluation criteria will vary depending on the job, however, they may include accuracy, precision, recall, or the F1 score.
AI model training is an iterative procedure. According to the performance review, you may need to go back and tweak your model, add more data, or perhaps choose a different model entirely. The objective is to increase the model's performance iteratively until it fulfills your needs.
Once you're satisfied with your model's performance, the following step is deployment. This entails incorporating the model into your application or system. After deployment, continual monitoring is required to ensure that the model works properly over time and to make any improvements.
Training AI models with your database also necessitates ethical concerns. It is critical to verify that your data is free of biases that might lead to unfair or discriminatory outcomes when the AI model is used.
AI is continuously changing, with new models and approaches being introduced regularly. Keeping up with the newest advances is critical to ensuring that your DIY AI remains successful and current.
DIY AI is an effective technique to harness your database for intelligent insights and automation. You may create AI models that are both successful and ethical by understanding your data, properly preparing it, selecting the right model, and adhering to best practices for training and assessment. With the correct strategy, DIY AI may alter your operations and provide a competitive advantage in today's data-driven environment. Remember that the key to effective AI is continual learning and progress, both for your models and for yourself as an expert in this exciting subject.
Join our WhatsApp Channel to get the latest news, exclusives and videos on WhatsApp
_____________
Disclaimer: Analytics Insight does not provide financial advice or guidance. Also note that the cryptocurrencies mentioned/listed on the website could potentially be scams, i.e. designed to induce you to invest financial resources that may be lost forever and not be recoverable once investments are made. You are responsible for conducting your own research (DYOR) before making any investments. Read more here.