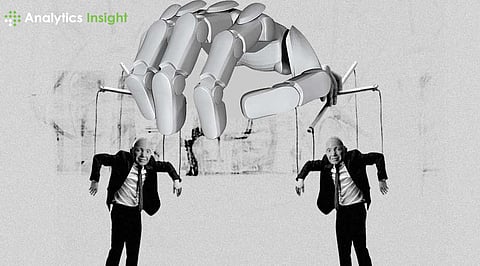
It is no secret that artificial intelligence (AI) is one of the most revolutionary technologies of our time. However, like all new technologies, there have been challenges along the way. Many of these challenges have been caused by a lack of knowledge about AI and a lack of realistic expectations about what it can do. Therefore, it is important to understand that the road to successful AI adoption will be long. Here are some of the biggest AI Blunders we all might have faced so far:
Chatbots were at the forefront of Artificial Intelligence (AI) for much of the late 90's and early 2000's. Chatbots were programmed to perform basic tasks over the Internet using natural language processing (NLP). This meant that they could engage in basic conversations with humans.
Now, AI blunders like chatbots failed to gain traction for a variety of reasons. For one, they were unreliable and often did not understand their human users. They were also difficult to train and expensive to build and maintain. It was not until deep learning technology was developed that chatbots became a commercial product. Today, chatbots are used by large companies such as Amazon, Walmart, and others to automate customer service processes. They are also used by e-commerce businesses to help customers shop online and make recommendations based on customer browsing history.
While chatbots are still far from being able to compete with human assistant in terms of their capabilities, they are one of the greatest success stories to date in terms of AI adoption.
Over the past year, Tesla on autopilot has been involved in a total of 273 crashes. The majority of these crashes were caused by the driver losing control while using Autopilot. However, many drivers have reported that their Teslas failed to recognize a stoplight and collided with the vehicles ahead of them. This could be due to the confusion the system may experience when a light changes, but it could also be due to human error. The question remains: Why didn't the Teslas respond to the traffic lights? Is it software updates? Or is it just bad engineering on Tesla's part?
Self-driving cars have been on the roads for a while now, but there are still many challenges that must be overcome before they can truly become autonomous. One such challenge is the development of advanced artificial intelligence that can recognize and react to a wide range of scenarios.
IBM’s Watson is the closest thing to a human-like supercomputer we have today. It can answer complex medical questions, help with foreign language translation, play the stock market, and even cook better meals. But its biggest accomplishment so far is beating two of the greatest champions in the history of the TV game Jeopardy. Just like Google’s image search, Watson faced criticism as it began to take on more and more of the human labor force’s tasks. There were reports of “humorous blunders,” “inaccurate diagnoses,” and “confusing answers.” Unlike Google, though, Watson hasn’t had much success in any commercial environment, and it is even been said to be virtually worthless outside of academia.
The main reason for this is that Watson is not yet able to communicate with humans naturally. As a result, it often has difficulty understanding its environment and is often unable to understand simple commands given to it by a human operator. The challenge for researchers in the future will be to find ways to train computers to think like humans so that they can assist us in solving some of the world’s most challenging challenges.
Google's Image Search was a groundbreaking application of artificial intelligence (AI). It was able to identify images by searching for patterns of color, shape, and texture. What many people did not realize was that the search engine’s algorithm was inherently flawed. It frequently failed to identify specific objects or color combinations, sometimes leading to embarrassing mistakes like the “potato” that showed up in a stock image of a typical Thanksgiving dinner. The search engine also failed to account for context and common photography tricks such as depth of field or color manipulation, which were detected as AI failures. As a result, Google made numerous mistakes, including misinterpreting things like the “World Trade Center” or even Abraham Lincoln’s face. Fortunately, Google was able to fix these issues and eventually became the dominant provider of online search. Some of the lessons that Google learned along the way are now invaluable to the advancement of future artificial intelligence technologies.
Amazon has made another face recognition mistake. The company’s AI system was supposed to identify criminals based on their facial images. But when it was tested using a set of photos of Congress members, it was found not only to be wrong but also racially biased, this was one of the biggest AI blunders found. According to the American Civil Liberties Union (ACLU), nearly 40% of the matches in our test for Recognition were of people of color, even though only 20% of Congress members are people of color. Whether it was a mistake with non-white faces or whether the training data was biased, it is hard to say. But relying solely on AI to determine a person’s criminal identity would be a foolhardy move.
There are still many AI blunders that need to be overcome before they can become fully autonomous. One of these challenges is the development of advanced artificial intelligence that will be able to recognize and react to a wide range of different scenarios.
Join our WhatsApp Channel to get the latest news, exclusives and videos on WhatsApp
_____________
Disclaimer: Analytics Insight does not provide financial advice or guidance on cryptocurrencies and stocks. Also note that the cryptocurrencies mentioned/listed on the website could potentially be scams, i.e. designed to induce you to invest financial resources that may be lost forever and not be recoverable once investments are made. This article is provided for informational purposes and does not constitute investment advice. You are responsible for conducting your own research (DYOR) before making any investments. Read more here.