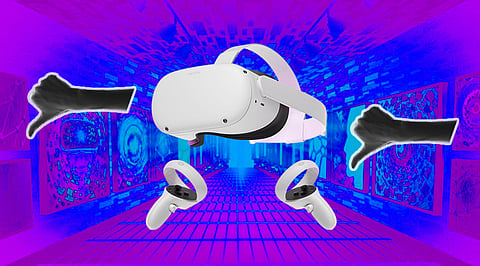
It will resemble a hybrid of today's online social experiences, expanded into three dimensions or projected into the physical world at times. It will allow you to share immersive experiences with others even when you are unable to be together and do things together that you would not be able to do in the physical world. It's the next step in a long line of social technologies, ushering in a new era for the company.
1.KILT Benchmarking Project – 11 knowledge-intensive datasets from 5 different tasks are combined into a single format by KILT, and the resulting Wikipedia dump is referred to as the KILT knowledge source. Large corpus pre-processing is a time-consuming operation that can significantly affect subsequent performance. Having all datasets mapped to a single corpus not only makes work in this area more convenient but also promotes fairer evaluation across different models.
2.COVID-19 Forecasting Project – To better understand how the virus spreads, Facebook AI works with professionals to create adaptable models and conducted research. The COVID-19 projections generated by the models provide information that can aid in early outbreak detection and resource planning for researchers, public health professionals, and organizations.
3.CICERO Project – The first artificial intelligence (AI) agent, CICERO, performed at a human level in the well-known strategy game. Because it involves players comprehending people's motivations and views, formulating intricate plans and change strategies, and using language to persuade individuals to form alliances, diplomacy has been seen as an almost insurmountable issue in AI.
4.Teaching AI advanced mathematical reasoning – Theorem proving in mathematics has long been viewed by the scientific community as an essential step in creating intelligent robots. Even the most sophisticated AI systems today struggle to demonstrate whether a given conjecture is true or incorrect since it calls for symbolic reasoning and navigating an unlimited number of options.
5.MultiRay: Optimizing efficiency for large-scale AI models – The universal models developed by MultiRay are honed to excel in a variety of tasks and industries. Teams across Meta can more quickly refine and iterate on machine learning (ML) models with MultiRay for a variety of uses, from topic-tagging posts to detecting hate speech. In addition, these tasks can be completed more effectively and with less labor than if each team were to construct substantial end-to-end models from scratch.
1.Meta Chatbots – Chatbots typically use a decision tree and are manually programmed. Creators specifically outline the steps they want the chatbot to perform in response to a user's input. Because it's practically difficult to pre-train a chatbot to function flawlessly in every circumstance, however, chatbots are unsuccessful.
2.Wave2letter Project – Utilize wav2letter++ to create a streaming voice recognition system. Simple memory loading of trained modules and effective processing graph. Numerous concurrent processing streams are possible. Loaded modules are compressed to maintain high throughput and extremely low latency while making efficient use of memory. However, this was not as successful as other projects.
3AI Research supercluster project – The design and construction of the AI Research Supercluster (RSC), which we believe is among the fastest AI supercomputers running today and will be the fastest AI supercomputer in the world when fully built. RSC has already been used by our researchers to train large models in natural language processing (NLP) and computer vision for research purposes, with the goal of one-day training models with trillions of parameters. It is argued that there are hidden loopholes in this.
4.Universal Speech Translator project – To provide real-time speech-to-speech translation across all known languages, including those that are largely spoken, the Meta project Universal Speech Translator (UST) created new AI approaches. One element of UST is the open-sourced AI translation system.
5.Project CAIRaoke – People will be able to speak naturally with their conversational assistants by models developed as part of Project CAIRaoke, so they will be able to bring up topics from earlier in a conversation, change the subject entirely, or mention things that require understanding a complex, nuanced context. Additionally, they will be able to communicate with them in novel ways, such as through gestures. However, this was not as successful as other projects.
Conclusion – However failures do not imply the complete failure of Meta AI, they are not successful as others. Thus, Meta AI came up with both successful and failed AI Projects this year.
Join our WhatsApp Channel to get the latest news, exclusives and videos on WhatsApp
_____________
Disclaimer: Analytics Insight does not provide financial advice or guidance. Also note that the cryptocurrencies mentioned/listed on the website could potentially be scams, i.e. designed to induce you to invest financial resources that may be lost forever and not be recoverable once investments are made. You are responsible for conducting your own research (DYOR) before making any investments. Read more here.