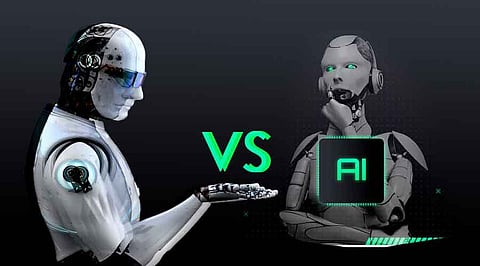
In today's fast-paced technological landscape, terms like "Machine Learning" and "Artificial Intelligence" are frequently used interchangeably. While they are undoubtedly related, they represent distinct concepts and play unique roles in the world of technology and innovation. This article delves into the key differences between Machine Learning (ML) and Artificial Intelligence (AI) to shed light on their separate but interconnected domains.
Artificial Intelligence, often abbreviated as AI, is a broad field of computer science dedicated to creating systems or machines that can perform tasks requiring human intelligence. These tasks encompass a wide range of activities, from problem-solving and language understanding to decision-making and perception. AI aims to develop systems that can mimic human cognitive functions, and it encompasses various subfields, including Machine Learning.
Machine Learning is a subset of Artificial Intelligence. It is a specific approach or methodology that enables computers to learn and improve from experience without being explicitly programmed. Instead of relying on static, rule-based algorithms, ML systems use data and statistical techniques to identify patterns, make predictions, and enhance their performance over time.
AI: AI encompasses a broader range of capabilities and goals. It aspires to replicate human intelligence in various domains, including natural language understanding, reasoning, problem-solving, and even creativity.
ML: ML focuses on the development of algorithms and models that can learn and make predictions from data. While it is a subset of AI, ML is a more specific and practical approach.
AI: AI systems may incorporate pre-programmed rules and logic, making them capable of responding to specific scenarios without learning from data. However, some AI systems incorporate ML techniques to enhance their adaptability.
ML: ML systems rely on data-driven learning. They improve their performance by processing and analyzing data, identifying patterns, and adjusting their behaviour accordingly. ML is inherently data-dependent.
AI: Examples of AI applications include virtual personal assistants (like Siri and Alexa), self-driving cars, and recommendation systems (Netflix and Amazon).
ML: ML is used in spam email filtering, image and speech recognition (as seen in facial recognition technology), and predictive text suggestions.
AI: AI systems may use various techniques, including symbolic reasoning, expert systems, and neural networks. They can employ both rule-based and learning-based methods.
ML: ML primarily relies on learning-based approaches. This involves feeding data into algorithms, allowing the system to identify patterns and relationships within the data.
AI: AI systems are designed to mimic human-like behaviour and intelligence. They aim to perform tasks in ways that resemble human decision-making and problem-solving.
ML: ML systems, while capable of learning and improving, do not necessarily aim to replicate human-like behaviour. Their focus is on data-driven predictions and decision-making.
AI: Developing AI systems often requires a combination of expertise in computer science, linguistics, psychology, and other fields. It can be a complex and resource-intensive endeavour.
ML: ML development is data-centric. It involves data collection, preprocessing, model training, and evaluation. While it still demands expertise, ML development is typically more focused on data analysis and modelling.
It's essential to recognize that AI and ML are not mutually exclusive but rather interrelated. Machine Learning is a crucial subset of AI, and many AI applications incorporate ML techniques to enhance their capabilities. In fact, ML has become one of the most prominent and practical approaches within the field of AI.
In summary, while the terms "Machine Learning" and "Artificial Intelligence" are closely related, they represent distinct concepts within the field of technology. Artificial Intelligence encompasses a broader scope of replicating human intelligence, while Machine Learning is a specific approach that empowers computers to learn from data and improve their performance. These distinctions are essential for understanding the roles and applications of AI and ML in today's rapidly evolving technological landscape. As AI continues to advance, Machine Learning remains a critical tool in achieving its ambitious goals of mimicking human intelligence and enhancing automation across various domains.
Join our WhatsApp Channel to get the latest news, exclusives and videos on WhatsApp
_____________
Disclaimer: Analytics Insight does not provide financial advice or guidance. Also note that the cryptocurrencies mentioned/listed on the website could potentially be scams, i.e. designed to induce you to invest financial resources that may be lost forever and not be recoverable once investments are made. You are responsible for conducting your own research (DYOR) before making any investments. Read more here.