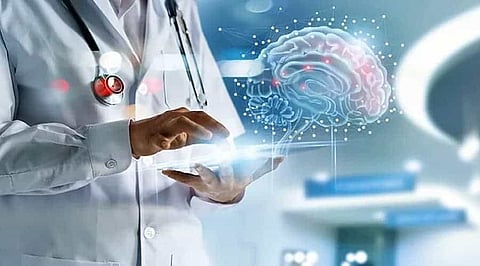
Healthcare professionals work very hard to serve every patient equally. So, when artificial intelligence (AI) comes into the picture, there is something to be worried about. Research on AI in healthcare has shown how beneficial it is to reduce costs and make healthcare more accurate, fast, and reliable. The racial issues with artificial intelligence are found in certain circumstances. For instance, a study finds that last year six algorithms used on about 60-100 million patients in the U.S were prioritising care for white patients over black patients for the same disease. But, AI cannot be entirely blamed.
Artificial intelligence's widely used application machine learning (ML) can help AI learn and make decisions based on data taken in and whatever algorithm the AI is using. It's important to understand that AI does not have any political plan, so it lacks motivation to show a bias. It's merely learning and making decisions based on its algorithm, and available data is being fed to it.
Having narrow data feeding any system indicates getting poor results. Data points, such as race, education, and, location can force machine learning to form biases. This bias is based on data and not on any hidden agenda that artificial intelligence has. In short, if the dataset is biased, AI will also be biased. To resolve this issue, you have to fix the kind of data that is being collected.
If the datasets are narrow, there are gaps in the data used to train AI. These gaps will manifest in the output of AI. "There is a case where facial recognition software was trained only on white people, cites Dr. John Frownfelter, Chief Medical Information Officer at Jvion.
"When it was put to use, it recognised people of all races, but only as white people." Therefore the training datasets will distort the output if it is not represented by the population upon which it is being used.
Narrow datasets also can expose artificial intelligence to any bias related to the data. In the case of racially-biased AI, the problem was that the AI melded the patients' health risk with the amount spent on their care in previous insurance claims. "Historically, black patients are underserved by healthcare; hence they have fewer insurance claims," he opines. As they are underserved, they have a greater risk. The presumption the AI was based on was wrong, but it was reflected in the output.
As artificial intelligence in healthcare deals with disease prediction and diagnosis, there is a potential bias. A lot of machine learning uses data related to medical history and symptoms. Thus, age and race would still be valid data points for AI to use.
Dermatology, for instance, is being introduced with many AI apps. These can analyse a picture of a skin lesion or mole and predict what the diagnosis is. Many outperformed dermatologists are identifying skin cancer. However, most datasets come from photos of the diseases on Caucasian skin. Therefore, the performance of these applications in darker skin types may be less than adequate.
On the other hand, "The market of artificial intelligence in healthcare has grown exponentially from $600 million to $6 billion in the past few years," says Dr. Frownfelter. "Gartner projects that 75% of health provider organisations will invest in AI by 2021 to improve operational performance or clinical outcomes."
Javion's AI that analyses more than 4,500 factors for each patient to avoid bias in any one dataset affecting the integrity of AI's output is now in use at over 300 hospitals and 40 health systems, with a database encompassing more than 30 million patients.
"AI is being experimented in radiology, where machine learning models are expected to recognise malignancies and other abnormalities in MRIs, X-rays, and mammograms," Dr. Frownfelter reveals.
Jvion navigates new patients over 99% of the time, regardless of race, gender, or age, allowing the data to speak for itself. They leverage machine learning power to figure out new associations and correlations within the data that otherwise would not be apparent.
Join our WhatsApp Channel to get the latest news, exclusives and videos on WhatsApp
_____________
Disclaimer: Analytics Insight does not provide financial advice or guidance. Also note that the cryptocurrencies mentioned/listed on the website could potentially be scams, i.e. designed to induce you to invest financial resources that may be lost forever and not be recoverable once investments are made. You are responsible for conducting your own research (DYOR) before making any investments. Read more here.