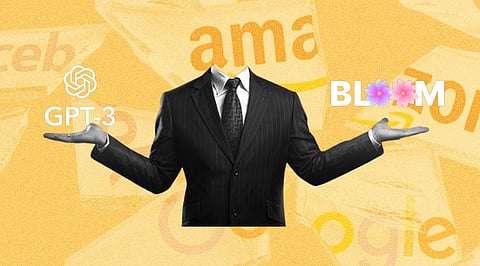
In the history of language models, BLOOM is going to be a revolutionary wave in determining the course of democratizing language models. BLOOM true to its name, The Big Science Language Open-Science Open-access Multilingual model, is out for everyone who wants to try. Trained on around 176 billion parameters, it is considered a direct competitor to the ever-magnificent Open AI's GPT-3 model. Ever since GPT-3 is out, it has created a huge debate over the secrecy of training NLP models in particular and the unpredictability of language models in general. Since the beginning, technology companies have kept the details of the training algorithms hugely undercover. In the last few years, though large machine learning models have changed the course of AI research, only a few AI research teams have been able to study these models due to high computational costs and huge amounts of data required. With the information of metadata, coding, and training data out of reach of many, the models and companies have come under fire for being monopolistic. In this regard, BLOOM is a step toward unraveling the hard-kept secrets of ML training, as a part of Big Science's general mission to make artificial intelligence open. Researchers are quite optimistic about its potential to even up and open other research avenues by extracting information from historical texts or making classifications in biology, etc. Thomas Wolf, the co-founder of Hugging Face, as quoted by Nature.com, says, "We think that access to the model is an essential step to do responsible machine learning."
With code sourced from around 1,000 academic volunteers from research organizations across the globe, it amounts to nearly US$ 7 million worth of publicly funded computing time. It comes with 70 layers and uses multi-head attention, a feature not found in its predecessors. Its rival GPT-3 is trained on 175 billion parameters, a count only slightly lower than that of BLOOM's 176 billion parameters, it pales before the latter in different departments.
BLOOM is a multilingual model, that can generate text in 45 natural languages and 13 programming languages. Even for tasks, it has not been trained for such as writing recipes, extracting data from news articles, or creating sentences using newly invented words, it takes prompts, features which are largely absent in GPT-3. Besides, BLOOM is said to have use cases outside AI, such as digging out information that internet browsers cannot. Moreover, these models come with huge environmental impacts which BLOOM promises to address by putting emission data in the public domain.
Given the fact that GPT-3 is trained on around 500 billion words scrapped from the internet, it is natural that we expect superior results. It is a myth that the more the number of words a language model takes in, the more accurate results it generates. It is the quality of data that determines the accuracy, not the quantity. BLOOM has taken a step ahead in this regard by handpicking only 500 sources for its 341-billion-word dataset. The team supplemented the data with a multilingual web crawl, filtered it for quality, and applied redaction for privacy. To reduce the unnecessary sexist associations by the model it reduced the over-representation of porn sites.
Although it is free to use, downloading and running it requires pricey hardware, which BLOOM has set to work on by announcing the release of a less hardware-intensive version apart from developing a web application that doesn't require hardware or a download. GPT-3 is nowhere near to ensuring the kind of accessibility BLOOM is vigorously trying to provide its users.
On the evaluation front, again it is BLOOM that scores. The benchmarks it is evaluated hugely differ from the usual ones. Now that it is open source, it would be easy to evaluate how it makes stereotyped associations, or how biased it is. Ellie Pavlick, a natural language learning researcher at Brown University, is hopeful that the multilingual training would impart in BLOOM the ability to generalize to a diversity of tasks. And when the ultimate litmus test for transparency and responsibility is called upon, BLOOM scores cent percent. With its documentation and code of ethics in place, one cannot ask for more. BLOOM's aim is not to destroy its predecessors nor to compete with them. However, with the kind of openness BLOOM is planning to deploy its model, not only GPT-3 but any other model developed on the premise of secrecy will struggle to survive.
Join our WhatsApp Channel to get the latest news, exclusives and videos on WhatsApp
_____________
Disclaimer: Analytics Insight does not provide financial advice or guidance. Also note that the cryptocurrencies mentioned/listed on the website could potentially be scams, i.e. designed to induce you to invest financial resources that may be lost forever and not be recoverable once investments are made. You are responsible for conducting your own research (DYOR) before making any investments. Read more here.