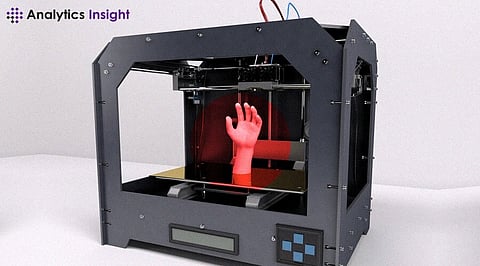
Machine learning, a subset of artificial intelligence, has witnessed widespread adoption across various industries, revolutionizing processes and enhancing efficiency. In the realm of manufacturing, machine learning in 3D printing has given rise to a paradigm shift in the way products are conceived, designed, and produced. In this article, we explore the transformative applications of machine learning in the dynamic field of 3D Technology.
Machine learning algorithms are excellent at analyzing large datasets and discovering patterns. In the context of 3D printing, these algorithms can optimize the design process by analyzing existing designs, material properties, and performance data. By doing so, machine learning aids in creating more efficient and structurally sound designs, ensuring that the final 3D-printed objects meet the desired criteria with minimal material usage.
Generative design, powered by machine learning algorithms, is a groundbreaking application that transforms the design phase. Machine learning models can generate multiple design options based on specified parameters and constraints. In 3D printing, this translates to the creation of intricate and optimized structures that traditional design methods might overlook, leading to lighter, stronger, and more resource-efficient components.
Machine learning plays a vital role in optimizing the 3D printing process itself. By analyzing real-time data from the printing environment, machine learning algorithms can identify patterns that indicate potential issues. This predictive maintenance approach allows for timely intervention, minimizing downtime and ensuring the quality of printed objects. Process optimization also involves adjusting parameters such as temperature, speed, and layer height to achieve the desired print quality efficiently.
3D printing materials come in various compositions, each with unique properties. Machine learning can analyze material data, user preferences, and performance requirements to recommend the most suitable materials for specific applications. This customization not only enhances the functionality of 3D-printed objects but also contributes to sustainability by minimizing material waste.
Ensuring the quality of 3D-printed objects is paramount. Machine learning algorithms can be trained to identify anomalies and defects in printed items by analyzing visual data. Whether it's irregularities in layer adhesion, surface finish, or dimensional accuracy, machine learning-based quality control systems enhance the reliability and consistency of 3D-printed products.
Integrating machine learning into the 3D printing process enables real-time monitoring of inventory, production, and demand. Predictive analytics can help streamline the supply chain by optimizing inventory levels, reducing lead times, and minimizing waste. This application is particularly advantageous in industries where on-demand production and customization are essential.
The intersection of 3D printing and machine learning is particularly impactful in the healthcare sector. Machine learning algorithms can analyze patient data to create personalized medical implants, prosthetics, and even pharmaceuticals. This not only improves patient outcomes but also contributes to advancements in personalized medicine.
In the field of bioprinting, where living tissues and organs are 3D printed, machine learning aids in the complex task of replicating biological structures. Machine learning algorithms can analyze cellular behavior, material properties, and biomechanics to optimize the bioprinting process. This has profound implications for regenerative medicine and organ transplantation.
Machine learning models can optimize the energy consumption of 3D printers by analyzing historical data and real-time variables. By dynamically adjusting printing parameters and scheduling jobs during periods of lower energy demand, machine learning contributes to the sustainability of 3D printing processes.
Machine learning algorithms can enhance the user experience on 3D printing marketplaces by analyzing user preferences, historical data, and market trends. This enables the recommendation of relevant and popular designs, fostering a dynamic ecosystem where users can discover, customize, and print designs that align with their preferences.
The fusion of machine learning and 3D printing heralds a new era of innovation and efficiency in manufacturing. From optimizing designs and materials to enhancing quality control and reshaping entire industries, the applications of machine learning in 3D printing are both diverse and transformative.
Join our WhatsApp Channel to get the latest news, exclusives and videos on WhatsApp
_____________
Disclaimer: Analytics Insight does not provide financial advice or guidance. Also note that the cryptocurrencies mentioned/listed on the website could potentially be scams, i.e. designed to induce you to invest financial resources that may be lost forever and not be recoverable once investments are made. You are responsible for conducting your own research (DYOR) before making any investments. Read more here.