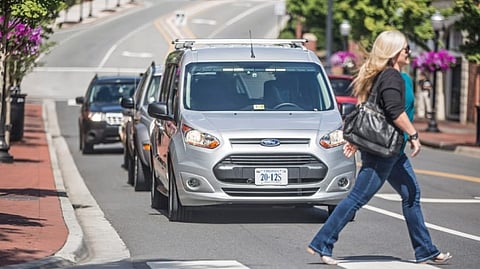
The beginning of the 20th century saw the automobile as mainly a plaything for the rich. To be able to own a car, you had to have a chauffeur conversant with the mechanical nuances of the models. Then Henry Ford entered the scene. He was not the inventor of the motor car but he worked on his determination to build a simple and an affordable car for the average American worker. This saw the birth of the assembly line production technique. This led to other automobile companies of the time to come up with innovations promoting faster, efficient and cheaper models.
With the advent of artificial intelligence, the automotive sector is now poised for another breakthrough in transportation with cars that can drive themselves. This time around, the race for supremacy is mostly concentrated amongst the corporate and technology giants – Tesla, Uber, Waymo, Ford, and General Motors.
If you ask any person to name an AI-driven system, chances are the person would come up with 'self-driving vehicles' as an answer most of the times. Typically, a driverless car is characterized by a large-scale deep learning system. Deep learning systems require huge datasets. So, where do we get this data about the environment? For this, we have something known as advanced sensors, used in mapping, localization and consequently obstacle avoidance. As much as it is costly, LIDAR (Light Detection & Ranging) is the main type of sensor used in autonomous vehicles. A LIDAR typically consists of a laser, a scanner and a specialized GPS receiver. Its remote sensing technology makes use of light in the form of pulsed laser to acquire data relating to ranges (variable distances) over broad areas. Natural and man-made environments can be examined with accuracy, precision and flexibility using LIDAR. In any case, a sensor fails to detect an object in front of the car, a 'radar' will take over the job. A sensor used in obstacle avoidance, a radar is usually directly hooked up to the control system and can recognize objects up to a distance of 10 meters.
The second part is the machine learning pipelines for perception. In order to successfully follow a trail, we can define three components for perception. First is localizing your vehicle and then based on this information making navigation decisions. The second component is object recognition wherein we apply the deep learning technology to make extensive use of camera data and as a result, leads to recognizing objects around the vehicles. A monocular image from a forward-looking camera can be considered as an input for the same. And, the final component is object tracking where again deep learning technology is used to track the cars and objects next to you.
Path planning and predictions form the decision pipeline. Planning or pathfinding is basically finding the shortest route between two points. Dijkstra's algorithm for finding the shortest path on a weighted graph is the basis for this field of research. Finding a path between two nodes in a graph and the shortest path problem—to find the optimal shortest path, are the two primary problems of pathfinding. The first one is addressed by eliminating all possibilities using basic algorithms such as breadth-first and depth-first. An exhaustive approach known as the Bellman-Ford algorithm is used for the second problem which yields a quadratic time. Also, paths can be strategically eliminated through heuristics or dynamic programming using algorithms like A* and Dijkstra's algorithm. The prediction algorithms are used to measure the probability of crashing into or avoiding nearby objects. Rule-based engines are currently in large use but soon there'll be a time when engines with autonomous decision-making capabilities will replace these.
Despite the development of sophisticated systems, there is currently no plan B in force for human road users. Humans make a small mistake while driving to which self-driving cars simply can't adapt. Last year in July, Google's self-driving car was hit by a human driver from the behind while waiting at a traffic signal. Despite possessing a sophisticated array of sensors, the car couldn't do much to avoid the incident. But it served as a stark reminder of the risk faced by autonomous cars when surrounded by human road users. Dealing with the unpredictable behavior of humans as both pedestrians and drivers present a significant challenge for this technology.
Join our WhatsApp Channel to get the latest news, exclusives and videos on WhatsApp
_____________
Disclaimer: Analytics Insight does not provide financial advice or guidance. Also note that the cryptocurrencies mentioned/listed on the website could potentially be scams, i.e. designed to induce you to invest financial resources that may be lost forever and not be recoverable once investments are made. You are responsible for conducting your own research (DYOR) before making any investments. Read more here.