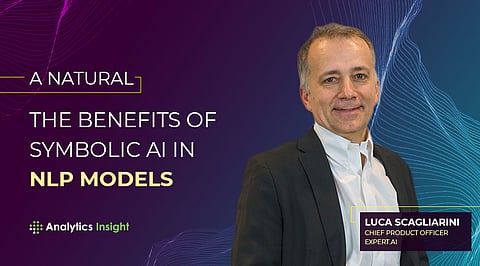
Natural language contains many ambiguities. As children, we learned about the world via symbolism, and with each new encounter, our minds added logical rules and formed relationships around concepts, objects, and more. While humans can easily disambiguate meaning in context by leveraging experiences and learning, the automatic interpretation of language remains a challenge for technology.
Artificial intelligence (AI) is increasingly delivering on its potential. For instance, through natural language processing (NLP), a segment of AI, computers can now interpret human language. In turn, they can effectively communicate with virtual assistant machines to serve customers and prospects. Sounds great, but such advancements come at a steep cost and consume a lot of data, time and processing resources when driven by machine learning (ML). And after all this investment, you still run the risk of less-than-accurate results.
Yet, equipped with the right AI strategy, enterprises can sidestep these challenges. Known as symbolic AI, this approach for NLP models delivers both lower computational costs and more insightful and accurate results.
As mentioned, we learn language context through associated relationships from childhood and through education. A symbolic approach works the same way, but for AI systems. It embeds a knowledge graph, a repository built to include the concept of a language and the relationships between these concepts. Commonly used for NLP and natural language understanding (NLU), symbolic AI then leverages the knowledge graph, to understand the meaning of words in context and follows IF-THEN logic structure; when an IF linguistic condition is met, a THEN output is generated.
This method helps to address a major flaw often associated with AI – the black box, where a system performs tasks and reaches results that can't be audited or explained creates a huge problem, particularly when it comes to concerns over privacy and bias. The black box scenario is endemic of the most known AI technique, ML, and presents an unacceptable level of risk for enterprise whereas issues could damage a reputation, deter or even harm consumers, and undermine support for future AI initiatives.
Symbolic AI makes it easy to create clear and explainable rules for full transparency. And with the flexibility to write your own rules, the logic behind symbols delivers a host of benefits.
When you build an algorithm with ML alone, changes to input data can produce model drift, requiring you to retrain the model and test it again. On the other hand, a symbolic approach to NLP allows you to easily overcome this because you can understand the logic, identify the issue and revise rules, saving time and computational resources.
A lack of language-based data can be a major problem when it comes to training an ML model, too. ML needs massive amounts of data just to get up and running, never mind for continually working. With a symbolic approach to AI, the ability to develop and refine rules can enable enterprises to work with smaller data sets.
What's more, a symbolic approach delivers greater accuracy out of the box. It accomplishes this by assigning a meaning to each word based on context and embedded knowledge. This is called disambiguation and it's proven key to the best NLP/NLU models.
Symbolic AI and ML aren't mutually exclusive. In fact, they work best combined in a hybrid model, enabling companies to capitalize on the strengths of each. Some AI platforms are designed with the flexibility to accommodate such a hybrid approach, incorporating symbolic, ML, and even deep learning.
This makes NLP tasks such as categorization and data mining easier. Further, you can develop an NLP solution using the symbolic technique for one set of data, use ML for another, then merge them both in a pipeline format for maximum performance.
The ability to harness unstructured language data from documents, social content, and emails and turn it into actionable insights is useful in a range of use cases. Here are some examples of how enterprises are applying symbolism and hybrid to address pain points.
Symbolic AI is fortifying NLP with its flexibility, implementation ease, and newfound accuracy. It performs well when paired with ML in a hybrid approach. And it's all accomplished without high computational costs. Moving ahead, while making a case for symbolic AI will no longer be an issue, explaining to the c-suite why you didn't could very well be.
Luca Scagliarini
As Chief Product Officer (CPO), Luca is responsible for leading the product management function and overseeing the company's product strategy. Previously, Luca held the roles of EVP Strategy & Business Development and CMO at expert.ai and served as CEO and co-founder of semantic advertising spinoff ADmantX. In his career, he held senior marketing and business development positions at Soldo, SiteSmith, Hewlett Packard, and Think3. Luca received an MBA from Santa Clara University and a degree in Engineering from the Polytechnic University of Milan, Italy. He blogs regularly about the real-world applications of AI and cognitive computing for today and tomorrow.
Join our WhatsApp Channel to get the latest news, exclusives and videos on WhatsApp
_____________
Disclaimer: Analytics Insight does not provide financial advice or guidance. Also note that the cryptocurrencies mentioned/listed on the website could potentially be scams, i.e. designed to induce you to invest financial resources that may be lost forever and not be recoverable once investments are made. You are responsible for conducting your own research (DYOR) before making any investments. Read more here.