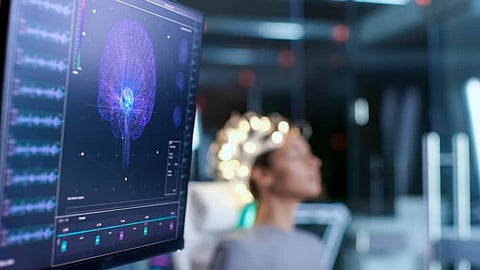
By establishing a LCA model for incucing slow, sleep wave, the neural networks would help in efficient working.
Just like Human Beings, continuous work can render any object, instrument and system to lose its focus, while performing the task. It not only makes them disoriented; incapable to deliver the desired results, but long hours of work without any residue of rest can also affect their performance. As a result, the tasks which can be accomplished in short duration of time, would require long hours of slogging with a compromise over the quality of work. Artificial Intelligence, is no different for facing this challenge. It has been observed by the researchers at Los Alamos University observed that continuous period of unsupervised learning renders the neural networks to become unstable. Hence, to rectify this issue, the researchers developed a neural network that would approximate the activites of the brain, by exposing the networks to an artificial analog of sleep for stabilizing it.
A study named, "Using Sinusoidally –Modulated Noise as a Surrogate for Slow-wave-sleep to Accomplish Stable Unsupervised Dictionary Learning in a Spike-Based Sparse Coding Model" by the Las Alamos National Laboratory used Locally Competitive Algorithm (LCA) to stimulate the neural networks for sleeping.
The study describes Locally Competitive Algorithm as a system that provides a biologically plausible implementation of sparse coding based on lateral inhibition. It is reformulated for reducing predictive coding error. The LCA describes a dynamics of neural networks which enables interactions of input stimuli by utilizing a local synaptic interaction between input stimuli.
Integrating the unconvolutional LCA with unsupervised learning, is observed as a method that support a large number of signal processing tasks.
The study states that by implementing unsupervised learning with non-spiking LCA, non-local computations, specifically transpose and normalization operations are utilized and requires signed outputs for representing the sparse reconstruction output. Thus with the help of a Nengo stimulator, the stability of a spiking LCA model due to unsupervised learning can be possible by incorporating the epochs of sinusoidally-modulated noise which are hypnothised as the analogous to slow-wave sleep.
It has been observed by researchers that in the absence of slow-wave sleep epochs, there can be an increase in the norm of [l2] individual feature of the model, due to unsupervised dictionary learning. Insertion of sinusoidally –modulated Gausian noise, would down regulate the [l2] norms of any activated neuron, thus render them inactivated due to noise.
As the Gausian noise uses waves and amplitudes, the study hypothesized that during the slow wave sleep, the noise can mimics the inputs received by biological neurons. It has been observed that the slow-wave sleep induces neural networks to maintain their stability without hallucinating.
Join our WhatsApp Channel to get the latest news, exclusives and videos on WhatsApp
_____________
Disclaimer: Analytics Insight does not provide financial advice or guidance on cryptocurrencies and stocks. Also note that the cryptocurrencies mentioned/listed on the website could potentially be scams, i.e. designed to induce you to invest financial resources that may be lost forever and not be recoverable once investments are made. This article is provided for informational purposes and does not constitute investment advice. You are responsible for conducting your own research (DYOR) before making any investments. Read more here.