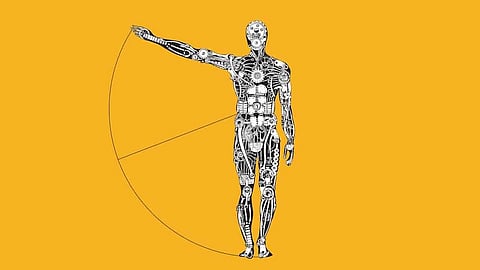
As AI develops progressively modern and pervasive, the voices warning against its present and future traps become stronger. Regardless of whether it's the increasing automation of specific jobs, sexual orientation and racial inclination issues coming from obsolete data sources or autonomous weapons that work without human oversight (to give some examples), anxiety proliferates on various fronts. We're still in the early stages.
In the previous few years, AI has advanced our technology at an amazing rate. From totally robotizing labor-intensive jobs to diagnosing lung disease, AI has gained accomplishments that we thought are impossible. However, in inappropriate hands, an algorithm can be a destructive weapon. To guarantee that malicious actors don't unleash havoc in our society, there are a few key challenges which we need to understand.
The real risk of AI isn't the ascent of a sentient algorithm like SkyNet taking control over the world. Despite the fact that this situation is totally sci-fi, there are a few authentic issues. Rather than fearing innovation, we should deliberately recognize these and take responsibility for handling them.
Regardless of whether any of those dangers are real is hotly bantered among researchers and thought pioneers. Yet, AI algorithms additionally present increasingly inevitable dangers that exist today, in manners that are less obvious and barely comprehended. The dangers of AI algorithms can show themselves in algorithmic bias and dangerous feedback loops and they can extend to all segments of everyday life, from the economy to social collaborations, to the criminal justice system.
While the utilization of mathematics and algorithms in decision-making is the same old thing, ongoing advances in deep learning and the expansion of black-box AI frameworks enhance their effects, both great and awful. What's more, if we don't comprehend the current dangers of AI, we won't have the option to benefit from its points of interest.
If you have ever applied for a job in the last few years, most likely you have been influenced by algorithmic bias, either positively or negatively. It may be a smart thought to create an AI-based algorithm for screening candidates for a job application, notwithstanding, there are noteworthy issues with this. Machine learning needs historical data to understand which candidates are worthy enough to get hired. The issue is, information about the past accepts and rejects is vigorously influenced by the characteristic human bias, for the most part towards women and underrepresented minorities. The algorithm just gains based on what is introduced to it and if past hiring practices were discriminative (similar to the case often), the algorithm will carry on along these lines.
A brilliant and provocative exhibit of this marvel is the Survival of the Best Fit educational online game, which is worth looking at. This issue influences numerous other application areas like credit score estimation, law enforcement, and so forth. As a result of the sheer effect of these in the lives of the individuals in question, these choices should not include algorithms, except if we can ensure fairness. The efforts towards this led to another and extremely dynamic research area.
Nonetheless, ensuring the independence of the prediction from variables, for example, gender, ethnicity, and similar ones affected by negative bias, the key is to create data where these are absent. To unravel this, a lot bigger endeavors are required. To totally expel inclination from information, we likewise need to expel bias from our intuition, since eventually, data is a result of our activities.
There are a lot of instances of AI algorithms making idiotic shopping recommendations, misclassifying pictures, and doing other senseless things. However, as AI models become increasingly more instilled in our lives, their mistakes are moving from benevolent to damaging.
Examples incorporate credit scoring frameworks that illegitimately punish individuals, recidivism algorithms that give heavier sentences to respondents dependent on their race and ethnic foundations, teacher-scoring systems that wind up terminating great performing educators and rewarding cheaters and trade algorithms that make billions of dollars to the detriment of low-salary classes.
There are two additional elements that make the harm of dangerous AI algorithms considerably destructive.
To begin with, the data. AI algorithms depend on quality information for training and precision. If you need an image classifier to precisely identify pictures of cats, you should give it many labeled pictures of cats. Similarly, a credit application algorithm would require heaps of historical records of loan applications and their result (paid or defaulted).
The issue is, the individuals who are harmed by AI algorithms are regularly the individuals on whom there's insufficient quality information. This is the reason loan application processors offer better types of assistance to the individuals who as of now have sufficient access to banking and punish the unbanked and underprivileged who have been to a great extent denied of the financial system.
The subsequent issue is the feedback loop. At the point when an AI algorithm begins to settle on tricky choices, its behavior produces progressively incorrect information, which is thus used to additionally sharpen the algorithm, which causes considerably more prejudice and the cycle proceeds perpetually.
When you make a bigger picture of how all these unique but then interconnected AI frameworks feed into one another, you'll perceive how the genuine damage happens. These issues are not strange, they are as of now present in our lives. With appropriate awareness, preparation and a community effort, these issues can be handled.