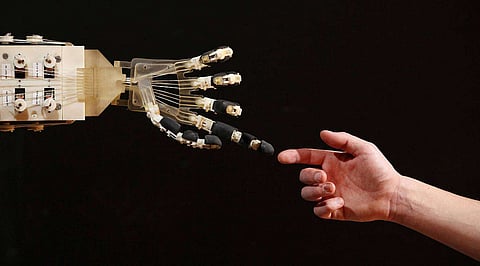
-------
Researchers at FZI Forschungszentrum Informatik in Karlsruhe, Germany, have formulated an approach that enables an anthropomorphic robotic hand to grasp objects, like humans using spiking neurons. The research paper titled, "Soft-grasping with an anthropomorphic robotic hand using Spiking Neurons" is published in IEEE magazine.
Soft grasping is an imperative capability of robots that enables them to grasp objects by working on a combination of control loops. Primitives are an integral part of a robotic system, ensuring control in robot movements while performing a task.
Researchers state that a traditional robotic system impaneled with deep learning control requires extensive data and stimulation time for training. Additionally, other prior approaches involved soft or flexible hardware or software using sensor feedback with robust modeling or using online learning for adaptive control or using cerebellar principles for compliance control in robotic movements. But none of the above-mentioned approaches exhibited the principles of biological phenomenon for motor control.
Henceforth researchers state that the proposed model employs the biological principles for motion in anthropomorphic robotic hands, using motor primitives, adaptive and compliant control, and event-based computation through spiking neural networks. The spiking neuron model is closer to the characteristics of real neurons present in the human brain. The four main components of this model are finger primitives, hand primitives, affordance activation, and reflexes. The finger primitives represent the joint synergies in a finger for a closing motion. The hand primitives represent different affordances coordinating the fingers. The affordance activation mechanism creates the activation patterns for the hand primitives. There are two types of reflexes activated by contact, one inhibits the movement of the fingers, and the other activates the compliant controller
The spiking neural network combines two control loops to generate complex grasping behavior. The first loop generated by the trajectory control is adaptive and closes the hand using human affordances. The second loop generated by compliant control is adaptive and uses the motor currents. The compliant control uses controls the force applied by the fingers for grasping an object.
The motions are represented by a hierarchy of motor representatives, with finger primitives representing synergies between joints and hand primitives representing a different level of coordination between the fingers. The reflex is triggered by a contact detection mechanism modeled as the circuits of interneurons in the spinal cord. This modeling simplifies the control of the hand and generalizes each grasp for different objects. Objects with different shapes, stiffness, and sizes are graspable without knowing their exact properties. The hand fits the grasping motions to the different objects using the compliant controller. The SNN is developed using the neural engineering framework.
Through this approach, the researchers were able to model sphere, cylinder, and pinch grasping techniques. In addition to this, only one or two demonstrations are required by SNN to train the primitives in different objects. The researchers observe that this approach can be continued for improving the controller, incorporating the visual information, and integrating the arm motion. The researchers also cite that the system implemented with SNN imitates biological brain neural responses for the grasping process and provides new insights into its sub-processes.
Join our WhatsApp Channel to get the latest news, exclusives and videos on WhatsApp
_____________
Disclaimer: Analytics Insight does not provide financial advice or guidance. Also note that the cryptocurrencies mentioned/listed on the website could potentially be scams, i.e. designed to induce you to invest financial resources that may be lost forever and not be recoverable once investments are made. You are responsible for conducting your own research (DYOR) before making any investments. Read more here.