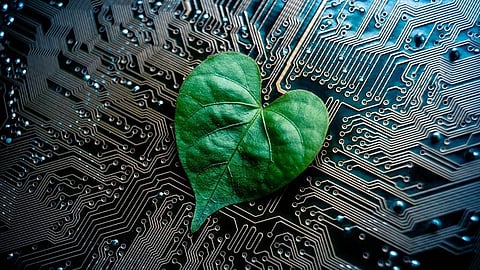
Artificial Intelligence has created a great hype in recent years. In some industries it is living up to it but its deployment in certain industries has been less touted. As it stands now, AI algorithms fuel the transport apps like Uber and Waze, digital assistants, commercial flights' autopilot and many more.
The impact of AI in energy efficiency and renewables has not been lauded enough. The technology is accelerating the value of wind and solar generation, energy storage and other areas through ML. In fact, Reinforcement Learning (RL) is one popular technique that is yielding impressive benefits in the energy space.
Through a dual approach of dataset training to optimize performance and mathematical function approximation to optimize solutions, reinforcement learning builds efficient algorithms to excels in large, complex decision spaces where restrictions and payoffs can be modelled.
Reinforcement Learning is performing effectively in power generation. DeepMind by Google is focusing on to make intermittent wind generation more predictable. Enabling function approximation on weather forecast data and training on turbine data, the RL algorithm forecasts power output 36 hours before the actual generation, subsequently allowing advance scheduling of electricity deliveries to the grid, changing load profile planning and effectively commoditizing the resource.
DeepMind AI has boosted the value of 700 MW of wind capacity supplying Google data centers by over 20 percent this year.
AI has a great impact on system management tool for solar plus storage installations. The technology provides enhanced control, flexibility, and value throughout the project lifetime. It also diversifies available value streams and balancing out the effect of variable rates. Specifically, RL in solar plus storage uses historical rate, capacity, and other data alongside creative function approximation to deliver predictive optimization for generation, storage, and purchasing.
In fact, a solution provider Stem Technologies estimates that its Athena AI platform adds 5-20 percent project value from demand charge and load shift savings through storage. It adds another 10 percent from optimized integration into utility demand response, wholesale, and other programs.
All together this can develop nearly 50 percent additional developer revenue, and 1.5-2 times the developer profit.
Although unnecessary hypes can easily lead to disappointment but potential of AI is being realized and incorporated in the energy sector. RL is one such AI technique that creates major efficiencies across the value chain, which will grow as software solutions. It will become more sophisticated and widely adopted. Several AI-powered companies haven't connected to separate AI applications into complete end-to-end automated processes but as soon as it happens full potential of AI will be unlocked.
AI's career as a disruptive technology in energy space is still at its infancy but it is the time that AI should be observed in a new light as substantial technology.
Join our WhatsApp Channel to get the latest news, exclusives and videos on WhatsApp
_____________
Disclaimer: Analytics Insight does not provide financial advice or guidance. Also note that the cryptocurrencies mentioned/listed on the website could potentially be scams, i.e. designed to induce you to invest financial resources that may be lost forever and not be recoverable once investments are made. You are responsible for conducting your own research (DYOR) before making any investments. Read more here.