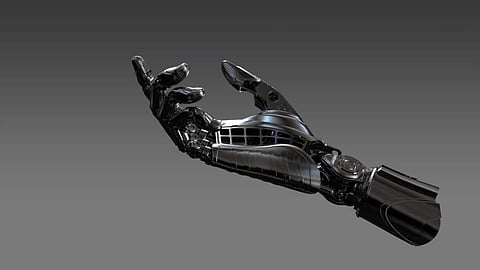
We, the people, can without much of a stretch tell how a thing looks like by basically touching, everything on account of our feeling of touch, which gives us that ability. Additionally, we can surely decide how a thing will feel just by taking a look at it.
However, doing likewise can be troublesome and a major challenge for the machines. Indeed, even the robots that are modified to see or feel can't do this. They can't utilize these material flags very as conversely.
Robots that have been customized to see or feel can't utilize these signals quite reciprocally. To better connect this tactile gap, scientists from MIT's Computer Science and Artificial Intelligence Laboratory (CSAIL) have thought of a predictive artificial intelligence (AI) that can figure out how to see by touching, and figure out how to feel by seeing.
The team's framework can make realistic tactile signals from visual sources of info, and foresee which thing and what part is being touched simply from those tactile information sources. They utilized a KUKA robot arm with an exceptional tactile sensor called GelSight, designed by another team at MIT.
Utilizing a simple web camera, the group recorded about 200 items, for example, tools, family unit items, fabric, and that's only the tip of the iceberg, being touched more than multiple times. Separating those 12,000 video cuts into static frames, the group arranged "VisGel," a dataset of more than 3 million visual/tactile paired pictures.
At the present time, the robot can just recognize things in a controlled environment. However, a few subtleties like the color and delicate quality of items, yet stay challenging for the new AI framework to close. In any case, the experts trust this new methodology will make way or more consistent human-robot coordination in assembling settings, particularly concerning activities that need visual information.
According to Yunzhu Li, CSAIL PhD student and lead creator on a new paper about the system believes that by taking a look at the scene, its model can envision the interaction of contacting a level surface or a sharp edge. By aimlessly touching around, their model can anticipate the connection with the earth simply from material sentiments. Uniting these two senses could enable the robot and decrease the information we may require for tasks including controlling and getting a hand on other objects
Late work to outfit robots with progressively human-like physical senses, for example, MIT's 2016 project utilizing deep learning out how to visually demonstrate sounds or a model that predicts articles' responses to physical powers, both utilize huge datasets that aren't accessible for understanding interactions among vision and contact. The group's method gets around this by utilizing the VisGel dataset, and something many refer to as generative adversarial networks (GANs).
The current dataset just has instances of interactions in a controlled domain. The group would like to improve this by gathering information in more unstructured zones, or by utilizing another MIT-designed material glove, to all the more likely increase the size and a decent variety of the dataset.
There are still details that can be dubious to gather from exchanging modes, such as telling the shade of a thing by simply touching it, or telling how soft a couch is without really pressing it. The analysts state this could be improved by making more strong models for vulnerability, to extend the distribution of potential results.
Later on, this sort of model could help with a more amicable connection between vision and robotics, particularly for object recognition, getting a handle on, better scene comprehension, and assisting with consistent human-robot incorporation in an assistive or manufacturing setting.
This is the first method that can convincingly interpret among visual and touch signals, says Andrew Owens, a postdoc at the University of California at Berkeley. Techniques like this can possibly be valuable for robotics, where you have to address questions like 'is this item hard or delicate?', or if I lift this mug by its handle, how good my hold will be?' This is an extremely challenging issue, since the signals are so unique, and this model has shown extraordinary ability.
Li composed the paper alongside MIT teachers Russ Tedrake and Antonio Torralba, and MIT postdoc Jun-Yan Zhu. It will be introduced one week from now at The Conference on Computer Vision and Pattern Recognition in Long Beach, California.
Join our WhatsApp Channel to get the latest news, exclusives and videos on WhatsApp
_____________
Disclaimer: Analytics Insight does not provide financial advice or guidance. Also note that the cryptocurrencies mentioned/listed on the website could potentially be scams, i.e. designed to induce you to invest financial resources that may be lost forever and not be recoverable once investments are made. You are responsible for conducting your own research (DYOR) before making any investments. Read more here.