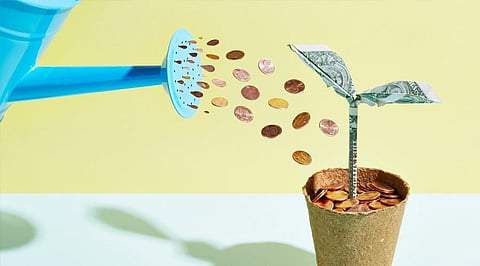
While AI has been around since the 1950s, certain trends such as the proliferation of data, growth of cloud computing, increased storage, etc. have pushed the adoption of it in the last five to ten years. With the advent of fintech, which has a specific emphasis on AI, the industry has experienced a disruption in some of its core practices. Likely the most influenced area is AI in asset management, which is expected to endure the biggest number of job cuts soon. Other challenges include growing passive investments, decreasing confidence, and decreasing investment fees.
The significant impact of the growing passive investments has been combined with regulatory pressure. The blend has designed a sensational decrease in investment fees. In Europe and the US, regulators have been planning to eliminate conflicts of interests, improve transparency and reveal the costs of investments. Some succeeded, others bombed.
A McKinsey report on data and analytics in asset management quotes, "The application of advanced analytics to specific business problems has begun to deliver value for traditional asset managers — not by replacing humans but by enabling them to make better decisions quickly and consistently."
Given the advancement of technology that comprehensively exists in all realms of society, it isn't astonishing that there continue to be new advances and use cases in asset management. Artificial intelligence, particularly, is helping to overcome the challenges of asset management and bring positive change. It will further improve efficiency, enhance decision-making, and manage risk.
Let's dive into some crucial areas where artificial intelligence asset management is effectively leveraged by investment firms.
AI in asset management industry can be utilized to perform matured fundamental analysis, including the utilization of text analysis and to streamline asset allotments in financial portfolios. In the midst of different difficulties of conventional portfolio optimization approaches, AI strategies give a better valuation of profits and covariances than more conventional techniques do. These evaluations would then be able to be utilized within conventional portfolio optimization systems. Additionally, AI can be utilized straightforwardly for asset allocation decisions to develop portfolios that meet performance targets more closely than portfolios made utilizing traditional techniques.
AI additionally has applications in risk management, concerning both market risk and credit risk. Market risk analysis consists of assessing, modeling and forecasting risk factors that impact the investment portfolio. Artificial intelligence investment management can utilize qualitative data for risk modeling, validate and test risk models, and generate precise predictions of aggregate financial or economic variables.
Asset supervisors have a guardian obligation to service customers at a lower cost. A vital use of AI asset management in operational functions is the monitoring, quality checking, and exception handling of the vast amounts of information on financial instruments that asset managers rely on. Data quality is absolutely critical as making fewer blunders lessens operational risk and secures the end customers. Data might be missing, old, or may contain errors. To help mitigate this, asset management machine learning can be utilized to identify and signal anomalies based on statistical assessments. For instance, a model may be able to take known data sources, for example, the average cost of a stock to decide whether the most recent cost received from a vendor is wrong.
Trading is another well-known field for AI use cases in asset management. Thinking about the growing speed and unpredictability of trades, AI for asset management is turning into a fundamental part of trading practice. An especially appealing component of AI is its capacity to process huge amounts of data to produce trading signals. Algorithms can be trained to automatically perform trades dependent on these signals, which has encouraged the rise of the industry of algorithmic (or algo) trading. Likewise, AI methods can lessen transaction costs by automatically analyzing the market and consequently recognizing the best time, size, and setting for trades.
Asset management firms should be more cost sensitive in the current operating landscape. Dealing with the expense of operations stays crucial to endure in the competitive field to counterbalance the rushes of new guidelines, fee pressures and the move to lower-cost passive products. Accordingly, numerous organizations are embracing huge change programs with an emphasis on outsourcing and process automation.
AI asset management is additionally filling in as an impetus for firms to transform conventional operational centers of excellence into services. Non-core operations can thus be externalized to expert service providers. Early AI asset management adopters will have the upside of changing over these "as a service" abilities into profit-centers and making an upper hand. Artificial intelligence asset management services that gain operational excellence can consistently improve at a particularly fast speed that it gets incomprehensible for contenders to get up to speed. By then, the service becomes both a defensible advantage and a sustained revenue source for firms
AI and ML tools can be used by investors to have better access to the financial markets and get digital advice. Considering attributes, for example, a client's age, pay, risk tolerance, and desired income in retirement, model-based AI digital tools can help financial investors select the proper asset allocation mix to meet their objectives. Digital advisors can fuse these new AI asset management tools and give an approach to people to get personalized investment advice at a lower cost than conventional advisory models.
Effectively embracing and implementing artificial intelligence requires smart, company-wide and operational risk contemplations for asset management firms. Data science leaders in this space should set aside time to comprehend and discover their difficulties and, alternately, the impact that is all the more often being witnessed when teams deploy data science and AI in investment management.
Join our WhatsApp Channel to get the latest news, exclusives and videos on WhatsApp
_____________
Disclaimer: Analytics Insight does not provide financial advice or guidance. Also note that the cryptocurrencies mentioned/listed on the website could potentially be scams, i.e. designed to induce you to invest financial resources that may be lost forever and not be recoverable once investments are made. You are responsible for conducting your own research (DYOR) before making any investments. Read more here.