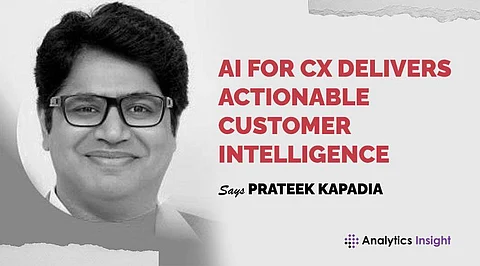
Businesses, often find themselves in the tricky situation of retaining low-value customers, and the most viable solution they have is upselling or cross-selling. Sometimes these techniques sound pushy even though the strategies are carefully crafted. What if marketing departments are equipped with data-based models and analytics powered by artificial intelligence to understand the purchase behavior of customers? Flytxt's 'AI for CX' is a customer intelligence solution specially designed to help companies achieve not only lifetime customer retention but also assured product profitability. Analytics Insight has engaged in an exclusive interview with Dr. Prateek Kapadia, CTO, of Flytxt.
The fast-growing Subscription Economy requires businesses to focus on the lifetime value generated by their customers. The customer lifetime value (CLTV) metric allows a business to reasonably predict how much revenue a customer will contribute over the average estimated length of time that they'll continue to be a loyal customer. Maximizing CLTV is a difficult task despite access to a vast amount of customer data and computing resources. Many enterprises lack the technical capability and in-house skills to measure, monitor, and influence the CLTV.
Flytxt makes it easy for subscription and usage-based businesses to maximize CLTV through its core technology consisting of the CLTV data model, analytics packages, and specialized AI. 'AI for CX' is a cloud solution that can deliver accurate and actionable customer intelligence to any CRM and Martech systems with ease via APIs. The solution leverages expertly curated, proven, well-trained and explainable AI trained on trillions of accurately labeled data; and hence can be adapted faster compared to general-purpose solutions delivering accurate results.
The AI for CX solution supports a variety of use cases across different CX workflows- sales, marketing, portfolio management, or customer care. For example, the solution helps improve the upsell/cross-sell process by determining the product affinity of customers and then suggesting offers fitting their needs and preferences. The solution provides accurate insights into customers' usage/purchase behavior to influence marketing processes like segmentation, campaign design, or retention. While launching new products and offers, product managers are given predictive intelligence to choose the right bundling or pricing options to optimize revenue or margin. It can even map the right agents to address customers' inquiries most efficiently and even provide human or virtual agents with recommendations to improve their efficiency and productivity. And we have seen a significant impact on business outcomes across these use cases – be it an increase in revenue and margin or reduction in customer churn or an improvement in the performance of customer care agents.
Artificial Intelligence is shaping the future of business in nearly every vertical and will continue to act as a technological innovator for the foreseeable future. As per Gartner research, by 2024, 10% of all digital commerce orders will be predicted and initiated by AI, and by 2025 75% of companies will "break up" with poor-fit customers as the cost of retaining them eclipses good-fit customer acquisition costs. But it is taking time for organizations to adopt AI due to a lack of technical know-how and required skill sets.
At Flytxt we have posed this challenge to ourselves and we have a solution – offering AI as a product. We envisaged it as an end-to-end product that provides tangible value-addition to its users. Value-addition could be automation minimizing user effort, assistance providing accurate faster solutions, or personalization scaling to several user personas. However, encapsulating ML models into AI products that can be configured according to the needs of an enterprise opens up several challenges. Hence, we adopted a lifecycle approach while productizing our AI with three key phases: design (product conception, scoping, and data selection); development (model-engineering and validation); and deployment (ongoing usage and monitoring).
Our lifecycle approach offers early validation of the AI value proposition while providing the flexibility to start with even heuristic or human-in-the-loop solutions to validate the actual user need. Key learning is that building and operationalizing large-scale AI products is more than important and requires a correct blend of technology, processes, human resource, and organizational structures. Specialized data management strategies and semantic data models with built-in automation for data quality checks are essential for AI success at scale.
Finally, customers expect AI systems to work like any other software product, and are oblivious to the inherent uncertainty in AI predictions. Thus, it is important to build trust in AI with techniques like privacy preservation and explainability, while leveraging tools and processes offered by MLOps frameworks to iteratively derive value from AI.
First, let us consider the advancements in AI on the cloud, which give several benefits like:
Faster ROI: Traditional ML projects require good infrastructure and resource management; they take a longer time to generate value. But AI/ML on the cloud generates value in a much shorter time. And then cost advantage, a pay-per-use model/on-demand computing is good for sporadic AI/ML workloads. However, shared computing in the case of multi-tenant solutions like ours further optimizes the cost, while providing flexibility to experiment and build AI features iteratively. And then there is the ease of scalability, AI on the cloud makes it easy to transition from a small pilot base to a production base with millions of users.
However, taking advantage of these advancements requires the right product architecture that exploits cloud elasticity, while handling privacy & security challenges as per the application needs. And Flytxt's platform provides application-level multi-tenancy while using a mix of shared and on-demand computing resources for AI workloads depending on model complexity, data volume, and response time/SLAs.
Second, let us consider the advancements in AI which now allow enterprises to work on datasets that they don't own. This raises a concern about data privacy and aggravates issues of trust in AI. To mitigate this, Flytxt has come up with a Federated Marketing solution that is powered by its patented and proven Privacy-Preserving Analytics technology. This solution ensures that B2C enterprises can share their intelligence on common customers' usage behavior among themselves to serve their customers well without compromising data privacy. With this unique technology, we delivered value to a Telco client by harnessing their data and one of their partners' data independently to determine the potential churn risk of common customers with 86% accuracy.
Flytxt AI empowers the world's biggest brands to solve one of the fundamental challenges of doing business today – how to maximize customer lifetime value (CLTV). AI platform providers or service providers focus on building models in the most efficient way, whereas our AI solutions are focused on maximizing desired business outcomes
across CX workflows.
Our partnerships provide subscription and usage businesses with the AI-driven solutions they need to measure, predict and influence customer behavior. This creates a deeper understanding of each customer's needs, enabling enterprises to deliver game-changing customer experiences with agility and precision, at scale. We have an AI that is trained and fine-tuned using real-world insights and patterns from more than a billion consumers and trillions of data points over 10+ years. Thus, this well-trained AI can analyze and derive complex patterns from usage and purchase data with greater accuracy, precision, and recall.
Moreover, our AI is proven to drive tangible business outcomes like growth in revenue, margin, or retention rate across our global deployments. For example, our AI-recommended offers have increased the monthly opt-in rate by 50% and AI-recommended campaigns contributed 40% higher revenue than the usual campaigns.
We wish to influence those business decisions across customer and product journeys with our AI that can maximize long-term customer value creation. Flytxt is investing in developing future-ready AI-powered solutions to support a variety of use cases across CX workflows spanning Sales, Marketing, Product Design, Customer Care, and so on. This is powered by our cutting-edge research in advanced areas of AI/ML along with our academic partner institutions, TNO Netherlands and IIT Delhi.
One recent example is in the area of launching new products or offers in the market. One of the key challenges that businesses face while launching them is ensuring their market performance and predictability in revenue or margin. We developed an AI using counterfactual reasoning that helps in predicting the performance of products or offers and makes recommendations as to how a product/offer needs to be packaged and priced to optimize revenue, margin, or coverage by looking at the performance of look-alike products/offers in the past. This use case was showcased as part of one of the selected catalyst projects at this year's TMForum's annual digital transformation world event in Copenhagen.
And then the other talk of the town in marketing is consumer data privacy, especially in the inevitable context of a third-party cookie-less world. This year, we received a patent for our privacy-preserving analytics technique that is uniquely designed to address this challenge for enterprises. It will allow two enterprises to share intelligence on common customers without actually moving any customer data between them thus honoring the privacy of data fully.
We are also advancing our AI to make it more autonomous and explainable to enable decisions at scale and for building trust and transparency in the decisions or recommendations given by AI.
Typically, analytics and AI are adopted as ML models that generate point predictions and insights. But the leaders in AI focus on creating end-to-end AI products.
Now, AI-as-a-product opens up several challenges as I briefly discussed earlier also.
How to embed an AI product into a user workflow that operates under shifting circumstances? How do we scale up AI from a small pilot base to millions of users? How can we continuously augment AI to increase its value-addition? How do we earn trust, despite the inherent uncertainties in AI predictions?
We tackled these challenges by taking a lifecycle approach while productizing our AI as I explained earlier. Our learning algos were drawn from modern AI research on lifelong learning and reinforcement learning. This allowed our AI to learn and generalize from experiences across deployments. Thus, early validation of the AI value proposition was possible. Furthermore, we used Privacy-Preserving Analytics and explainability to build trust in AI. Also, we leveraged tools and processes from MLOps for iterative value generation from AI. I am happy that at Flytxt we are solving some of these universal challenges and this will open up new opportunities especially in realizing business value for enterprises with a vast amount of first-party data.
Join our WhatsApp Channel to get the latest news, exclusives and videos on WhatsApp
_____________
Disclaimer: Analytics Insight does not provide financial advice or guidance. Also note that the cryptocurrencies mentioned/listed on the website could potentially be scams, i.e. designed to induce you to invest financial resources that may be lost forever and not be recoverable once investments are made. You are responsible for conducting your own research (DYOR) before making any investments. Read more here.