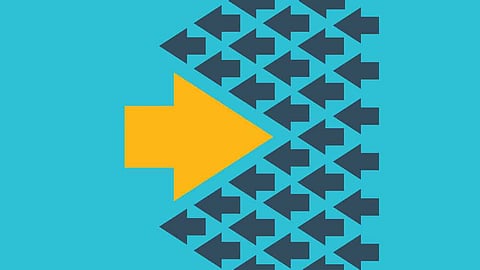
Data is an indispensable asset in modern-day business, irrespective of a large MNCs or a small, locally owned business. If an organization doesn't have an effective data strategy in place, they will miss out on the huge potential business value that data offers. Big data and analytics can be a promising investment for enterprises as it allows them to glean and process large datasets to derive valuable insights. However, leveraging data can also bring confusion and deter businesses from realizing its full potential. So, before getting started into data projects, enterprises must consider the top 6 big data mistakes and avoid them.
Keeping with ever-changing environments, businesses are required to adapt themselves to the advanced strategies and solutions. Most companies still use conventional key performance indicators that may hold them back from those that are exploring new tools and technologies. Hence, to prosper in today's fast-moving digital world, organizations need to use novel and more suitable tools to make advanced data analytics tools that reflect the current performance of the business and identify what really drives a business forward.
Security and governance are the major crucial business concerns. While organizations are started leaning towards big data analytics projects, they are moving towards it without security and governance baked in. In this way, they need to consider a multifaceted approach for securing big data. This should involve an understanding of the data possessed, auditing the manipulations of data, and holding control over the privileged users. In addition, they must comprise compliance, governance and security conversations that started at the beginning of the project.
In order to get a data project off the ground, there is a need for a significant amount of change, that many companies gravely underestimate it. Undoubtedly, planning technical costs to deploy a data strategy is vital, but overlooking to budget for items outside of technical costs can create potential challenges. Along with technical costs, businesses need to plan budgeting for skills development, training and change management within the organizations that can bring a cultural change to the effective utilization of big data and analytics.
Today, data comes from various sources and in many more forms than just databases and spreadsheets. Most data businesses collect are unstructured or raw data such as photos, sound recordings, text files and so on. Thus, having a robust data strategy needs to account for structured and unstructured data that can excerpt meaningful insights. However, overlooking external data sources including data repositories, governments and data brokers can put businesses' data projects at standstill. They must deliberate every type of source of data that can deliver value for the business.
According to Michael Stonebraker, Co-Founder and CTO of Tamr and Turing award winner, leaders often feel like they are on top of data science, and things like algorithm development, because they have hired data scientists. But data scientists typically spend most of their time assessing and cleaning data and integrating it with other sources. But it's also important to realize how data scientists are actually spending their time. So, having a clear strategy for dealing with data cleaning and integration, and having a chief data officer on staff can help address a company's data science problems.
There is no wonder that AI and machine learning have a considerable impact on all industries. The technologies will disrupt all aspects of businesses and improve operations and employee productivity. But most organizations still lack behind realizing the capabilities of AI and machine learning technologies. So, companies that want to be on the disrupting end of AI must be willing to pay for their talent. This means they must pay higher on AI and ML expertise that can help them become disruptors, instead of being disrupted.
Join our WhatsApp Channel to get the latest news, exclusives and videos on WhatsApp
_____________
Disclaimer: Analytics Insight does not provide financial advice or guidance. Also note that the cryptocurrencies mentioned/listed on the website could potentially be scams, i.e. designed to induce you to invest financial resources that may be lost forever and not be recoverable once investments are made. You are responsible for conducting your own research (DYOR) before making any investments. Read more here.