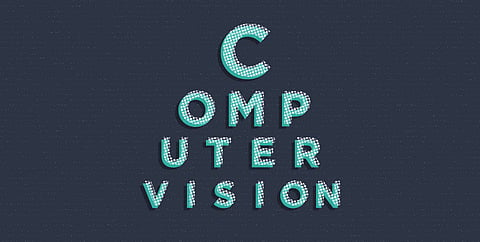
Recent developments in computer vision provide tools to the data scientists to automate a broader range of tasks. Yet companies keep wondering how best to recruit machine learning for their particular niche. One of the most common problems is recognizing how a machine learning model will perform the task uniquely than an individual would.
Computer vision is an integrative field that enables computers to recognize, process, and analyse images. It uses algorithms that can process both static images and videos. Professionals endeavour to deliver a computer version of human sight while reaping the benefits of digitization and automation. Sub-disciplines of computer vision include image restoration, object recognition, and anomaly detection. Wherein modern computer vision systems rely foremost on machine learning upon, there are also some pre-activated solutions for performing easy and simple tasks.
The below case studies show computer vision in action:
To diagnose Diabetic Retinopathy generally requires a proficient ophthalmologist. The rise in obesity globally, so is the threat of diabetes. As the World bank hints at a potential crisis of obesity in world development, including Latin American countries. Obesity increases the risk of 80-85% to develop type 2 diabetes, which needs proper diagnosis.
Deepsense.ai delivered a system that diagnoses diabetic retinopathy, which has accuracy equal to a trained human expert by applying algorithms to analyse the retina's digital images.
The algorithms are trained to spot the difference between healthy and diseased retinas. These are equally capable of finding blemishes on old films and making the classics bright again. Camcorded on a celluloid film, old movies are endangered by two factors. One is the fading technology of reading tapes, which allows users to watch them out. Another one is the nature of tape that degenerates gradually with age.
When trained on two versions of a film- digital noise version and the perfect one- the trained model spots the disturbances and omit them during the AI movie restoration process.
A push towards digitization comes via industrial installation documentation. Like films, documentation is trimmed to inconsistencies in the symbol used to be lost in the myriad of lines and other write-ups, which end up in the documentation. Humans must make sense out of it. Digitizing industrial documentation requires a skilled engineer capable of intense working up to ten hours. On the other hand, machine learning can take up a mere 30 minutes to finish off the same amount of work.
Despite seeming similarities, satellite images, and fully-functional maps that provide actionable information are two different things. The differences are vague as much as during a natural disaster such as a flood or hurricane, which can quickly render irrelevant maps.
Using image recognition technology, deepsense.ai has developed a solution to instantly turn satellite images into maps containing buildings, roads, trees, and the innumerable obstacles that emerge during a crisis. They used the model's architecture to create similar maps used to restore films and diagnose diabetic retinopathy.
Techniques of computer version can also work on aerial images as they operate on satellite images. Last year, a computer vision system was launched to support the US NOAA in recognizing each North Atlantic Right whales from aerial images.
With nearly 411 whales alive, the species is highly endangered. Therefore it is pivotal to recognize each individual so that its well-being can be traced. Before delivering the AI-based system, its identification was manually handled using a catalogue of whales. As the whales dive and rise to the ocean's surface, tracing them from aircraft is monumentally difficult. Such obstacles made the process not only time-consuming but also prone to error. Deepsense.ai introduced an aerial image recognition technology that improves identification with accuracy and takes only 2% of the NOAA's time once spent on manual tracking.
Join our WhatsApp Channel to get the latest news, exclusives and videos on WhatsApp
_____________
Disclaimer: Analytics Insight does not provide financial advice or guidance. Also note that the cryptocurrencies mentioned/listed on the website could potentially be scams, i.e. designed to induce you to invest financial resources that may be lost forever and not be recoverable once investments are made. You are responsible for conducting your own research (DYOR) before making any investments. Read more here.