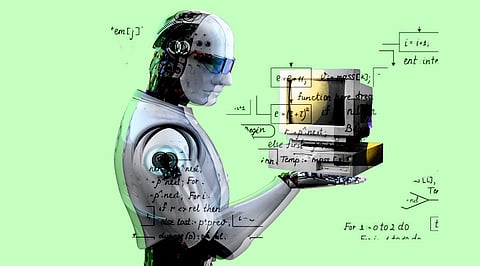
DataOps has evolved as a vital concept in the digital transformation era, ensuring seamless data flow through an organization. It entails orchestrating data processing and data quality checks to guarantee that data is correct, consistent, and easily accessible. It is especially essential in the field of AI and Machine Learning, where the quality and accessibility of data can have a substantial impact on model performance. To understand patterns and generate accurate predictions, machine learning algorithms rely significantly on high-quality data. As a result, including DataOps in AI and Machine Learning initiatives can result in more efficient data processing, better data quality, and, ultimately, more accurate and trustworthy machine learning models. Here are the 5 applications of AI and machine learning for DataOps.
Here are two crucial considerations for data operations teams to consider regarding the impact of manual efforts. What is the cycle time from when a new data set is discovered to when it is loaded, cleaned, joined, and listed in the data catalog in the organization's data lake? Are you using monitoring and automation to detect and adjust to changes in the data format once you've established a data pipeline? When manual data processing procedures are required to load and support data pipelines, data teams can use this time to improve cycle speeds for new data sources while recovering from data pipeline difficulties.
Broken data pipelines occur when DataOps engineers fail to employ monitoring, alarms, and automation to identify and fix issues swiftly. DataOps observability technologies and methods for logging data integration events and monitoring data pipelines are examples of proactive remediations. Data observability seeks to provide consistent and dependable data pipelines for real-time decision-making, dashboard updates, and use in machine learning models. It's one method for DataOps teams to manage service-level objectives, a concept developed in site reliability engineering and applicable to data pipelines.
In the future, as generative AI DataOps capabilities become more common, they have the potential to enable data observability at scale by identifying data issue patterns and recommending remediations or triggering automated cleansing, recommending code fixes and suggestions to data pipelines, and documenting data pipelines and improving the information captured for data observation.
Data operations teams can also use AI and machine learning to examine and classify data as it flows through data pipelines. Identifying personally identifiable information (PII) and other sensitive data in datasets that aren't designated as containing this type of information is one of the most basic classifications. Once the source has been determined, data governance teams can develop automation rules to categorize it and activate other business rules. Another use case for data compliance is security. Tyler Johnson, co-founder and CTO of PrivOps, spoke with me about how identity and access management is an often-overlooked area where DataOps can add value using automation and AI.
Identifying sensitive information in a data stream and other anomalies is a fundamental data governance use case, but what business teams truly want is faster access to cleansed data. Real-time updates to client data records are a primary use case for marketing, sales, and customer care teams, and one technique for centralizing customer information is to stream data into a customer data profile (CDP) database. A second way to manage customer data is master data management (MDM), in which DataOps sets the criteria for recognizing the primary customer records and fields from numerous data sources. Expect more generative AI capabilities in CDP and MDM systems, particularly around augmenting customer records with information from documents and other unstructured data sources.
DataOps can employ AI and machine learning to change their primary responsibility from data cleansing and pipeline maintenance to offering value-added services like data enrichment. Ashwin Rajeeva, co-founder and CTO of Acceldata, discusses how machine learning (ML) may enable continual data quality improvements by learning from patterns.
Join our WhatsApp Channel to get the latest news, exclusives and videos on WhatsApp
_____________
Disclaimer: Analytics Insight does not provide financial advice or guidance. Also note that the cryptocurrencies mentioned/listed on the website could potentially be scams, i.e. designed to induce you to invest financial resources that may be lost forever and not be recoverable once investments are made. You are responsible for conducting your own research (DYOR) before making any investments. Read more here.