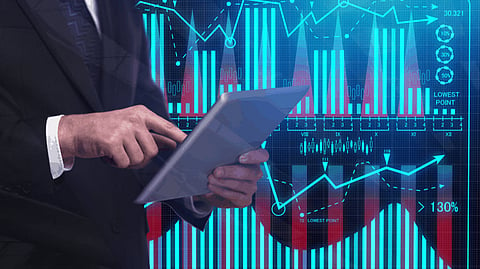
Predictive analytics is not confined to a particular niche; it finds its use cases and possible applications across industries and verticals. Analytics Insights brings you the 10 use cases from manufacturing, banking, healthcare, education, to name a few that combine AI technology with predictive analysis for improved efficiencies and improved customer experience:
Predictive analytics are increasingly deployed in the banking industry to identify potential fraud by studying and analyzing the most common operational patterns with regards to purchases, trades, and payments.
Predictive models work with both structured data (transactions) and unstructured data (reviews, emails, and forum entries) to understand the hidden data patterns. There are monitoring systems in place for some banks that scan data regularly for lead generation, identify opportunities for cross-selling and upselling, customer retention, customer relationship management.
Churn management is a concerning topic in the telecom industry. Predictive algorithms are used in the telecom industry to devise strategies to reduce or avoid churn. Telecom companies use predictive analytics for Analytical Customer Relationship Management (ACRM), Fraud Reduction, Bad Debt Reduction, Price Optimisation, and Call Centre Optimisation.
Predictive analytics is a buzz word in agriculture with the immense untapped capability to improve agronomic opportunities, such as product decisions, amounts of products, and profitability of decision making. Algorithms learning from historical and future data based on the measured field to field, acre to acre, and within acre variability can be readily deployed to impact processes and crop harvesting.
Retail industries operate in an intensely competitive environment, to improve customer conversion rates, predicting and avoiding customer churn, lowering customer acquisition costs, and personalizing marketing campaigns to increase revenue. All of this can be tackled with deeper, data-driven insights collated from customer data collected from mobile, social media, stores, e-commerce sites, and more purchases.
Weather forecasting has considerably been more accurate. Credit to the advanced predictive analytics models, helping governments and meteorological agencies to warn citizens and take necessary actions in case of natural calamities, floods, and by using predictive analytics algorithm models.
Satellite monitoring is used to collect data about the atmosphere and land which is then fed into weather forecasting models. Based on the model accuracy, weather changes are predicted for as long as a fortnight.
Machine downtime can cost companies thousands of dollars per hour. This makes predictive analytics so vital for the manufacturing industry to predict downtimes when a machine will go down to schedule preventive maintenance hours or days ahead of downtime.
Predictive analytics has a great untapped potential for the education sector in the field of personalizing the learning experiences. Educators can identify patterns of students' learning behaviors and customize the academic experience and course schedules which they would be most interested to learn.
In the event of a big product launch or any other activity where a call center is expecting a surge in volume, predictive analytics can be incredibly useful to determine the staff to handle excessive call surge. Once the predictive system determines the number of personnel needed in the coming days or week, the call center can move towards staffing appropriately to keep wait times at an acceptable minimum.
The efficiency of claim management increases drastically if data like customer behavior habits, financial health are known in advance. This can streamline the process which traditionally took weeks and even months helping the claims department to mitigate risks. This also allows insurers to analyze their claims processes based on historical data and make informed decisions to enhance efficiency.
Historical drug trial data can help pharmaceutical companies build predictive algorithms that compare their drug trails with the operations and procedures of past trials. For instance, a pharma scientist may also search for past drug trials for coronavirus based on the specifics of the drug being tested and find which practices may lead to a successful vaccine formulation.
The same holds if we speak about the discovery of new molecules and compounds which can produce new drugs. Predictive data analytics may help marketing teams as well. They can determine the geographies for a potential demand, and accordingly, target the marketing campaigns for new drugs.