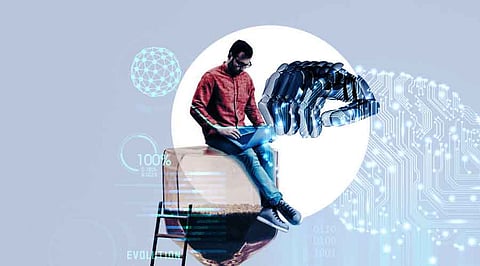
Machine learning (ML) is a branch of artificial intelligence (AI) that enables computers to learn from data and make predictions without explicit programming. ML can be applied to various domains and problems, such as natural language processing, computer vision, recommender systems, and more. One of the most promising and essential applications of ML is cybersecurity, where ML can help businesses improve their security by detecting and preventing cyberattacks, analyzing and responding to incidents, and enhancing their security posture.
1. Establishing Clear Objectives: When using machine learning in cybersecurity, having a specific goal in mind is crucial. Are you concentrating on virus detection, recognizing bogus emails, or anticipating potential internal threats? The decisions that follow will be guided by establishing this clarity up front. Setting a precise target reduction percentage is advised, for instance, if the main goal is to reduce the number of erroneous warnings.
2. Data Collection and Refinement: Any machine learning model's efficacy depends on the quality of the data it uses; therefore, for cybersecurity, it's essential to collect a wide variety of data in order to identify dangers and improve prediction. However, gathering data is only the beginning. Making sure the data are uniform is also critical. The machine learning model's training phase can be made more streamlined and effective by eliminating abnormalities or useless data.
3. Choosing the Right Machine Learning Techniques: The selection of ML algorithms will be based on your objectives. For instance, decision trees may be more effective at addressing straightforward rule-based anomalies than deep learning approaches may be for more intricate patterns. A cybersecurity and machine learning specialist should be consulted if there is any doubt in the decision-making process.
4. Training and Validating the Model: Data introduction to the machine learning model during training is the next stage after preparing the data. Determine this model's accuracy and reliability by validating it against a different dataset that it hasn't previously encountered. This validation process's feedback is used to improve the model's performance.
5. Ensuring Prompt Threat Analysis: The phase of integration is essential. The improved machine-learning solution ought to be easy to include in current technological frameworks. The system should also provide real-time monitoring and take immediate action in response to threats that are detected.
6. Prioritizing Continuous Model Evolution: Since the cyber environment is constantly changing, cybersecurity solutions also need to adapt. Even when there aren't any immediate threats, the model stays flexible and up to date by periodically supplying the system with fresh threat intelligence data and modifying its training cycles.
7. Continuous Monitoring and Refinement: Setting standards and regularly evaluating them are essential. Any variations offer a chance to reevaluate the approach and make the required modifications.
8. Compliance and Ethical Considerations: In the modern digital era, data protection laws like GDPR should be taken into consideration, especially when processing sensitive user data. Furthermore, it is crucial to use machine learning properly, assuring moral data processing and upholding individual privacy rights.
9. Keeping Security Personnel Engaged and Educated: To optimize the efficiency of the machine learning system, security employees must be knowledgeable about how it works. Facilitating a collaborative climate and holding regular training sessions so that security and data specialists who collaborate can take advantage of their combined knowledge.
10. Staying Updated with Industry Developments: Cybersecurity and machine learning are expanding fields. It's crucial to keep up with the most recent tactics, formulas, and best practices. Being an active participant in relevant communities, forums, and events may be pretty helpful for sharing perspectives and picking the brains of other business experts.