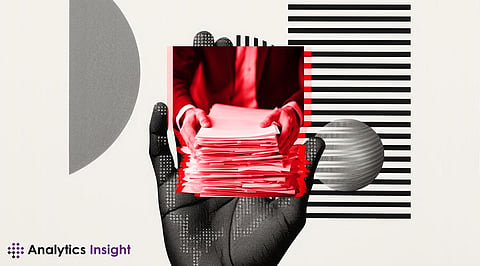
Data science interviews are becoming increasingly common as organizations seek to leverage data-driven insights for strategic decision-making. These interviews often include case studies that assess a candidate's ability to apply data science techniques to real-world problems. Let's explore some top case studies in data science interviews.
Customer Segmentation: Companies often want to understand their customer base better to tailor their marketing strategies. In this case study, candidates may be asked to segment customers based on various factors such as demographics, purchasing behavior, or geographic location.
Churn Prediction: Predicting customer churn is critical for businesses looking to retain customers and maximize revenue. Candidates may be presented with data on customer interactions and asked to build a predictive model to identify customers at risk of churning.
Recommendation Systems: Recommendation systems are widely used in e-commerce, streaming services, and social media platforms to personalize user experiences. Candidates may be tasked with designing a recommendation algorithm based on user preferences and historical data.
Predictive Maintenance: Predictive maintenance helps companies anticipate equipment failures and minimize downtime. Candidates may be given sensor data from machinery and asked to develop a model that predicts when maintenance is required.
Fraud Detection: Fraudulent practices can lead to considerable financial losses for businesses. Candidates may be provided with transactional data and tasked with building a fraud detection model that can identify suspicious patterns and anomalies.
Sentiment Analysis: Sentiment analysis involves analyzing text data to determine the sentiment or opinion expressed within it. Candidates may be asked to analyze customer reviews or social media posts to gauge public sentiment towards a product or brand.
Time Series Forecasting: Time series forecasting involves predicting future values based on past observations. Candidates may be given historical data, such as stock prices or sales figures, and asked to develop a forecasting model to predict future trends.
Image Classification: Image classification involves categorizing images into predefined classes or categories. Candidates may be provided with a dataset of images and asked to build a classification model that can accurately identify objects or patterns within the images.
Natural Language Processing (NLP): NLP techniques are used to extract insights from unstructured text data. Candidates may be tasked with building a text classification model, performing named entity recognition, or generating text summaries.
A/B Testing: A/B testing is a method used to compare two versions of a product or service to determine which performs better. Candidates may be presented with A/B testing results and asked to interpret the findings and make recommendations based on the data.
In conclusion, case studies in data science interviews offer candidates the opportunity to showcase their problem-solving skills, analytical abilities, and domain knowledge. By preparing for these case studies and understanding the underlying concepts, candidates can increase their chances of success in data science interviews and contribute effectively to organizations leveraging data science for decision-making.