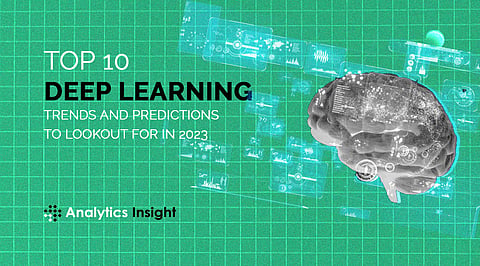
AI and machine learning are considered the foundations of tech transformation in the modern industry. Integrating machine learning algorithms into business operations has made enterprises more intelligent and efficient. As the next paradigm change in computing is underway, the evolution of deep learning has also caught the attention of industry specialists and tech giants. Currently, deep learning technology is an integral part of global industries. Artificial neural networks are at the core of the deep learning revolution. Deep learning trends predicted by experts reveal that this advancement of ML and its adjoining technologies has minimized the overall error rates and has also improved the performance of the networks for a particular task. In this article, we enlist the top deep learning predictions and trends that tech enthusiasts can watch out for in 2023.
Independent deep learning: Even though DL has excelled in several fields, the technology's rliaence on huge amounts of data and computing power has always been one of its limitations. But the year 2023 might witness the integration of unsupervised learning in DL, where instead of training a system with labeled data, it is trained to self-label the data itself using raw forms of information.
Integrating hybrid models: The year 2023 might witness the convergence of symbolic AI and deep learning. Symbolic AI initially dominated the tech domain back in the 70s or 80s, where the machine learned to interpret its environment by creating internal symbolic representations of the problem and analyzing the human decisions for the same. These hybrid models will be aimed to use the advantages of symbolic AI and integrate it with deep learning for enhanced solutions.
Using deep learning in neuroscience: Several research operations in neurology have revealed that the human brain is comprised of neural nerves. These artificial neural networks in the computer are synonymous with the ones humans possess in their brains. With the help of this phenomenon, scientists and researchers have been able to find out thousands of remedies and theories in neurology. Deep learning has provided the much-needed boost that neuroscience required a long time ago.
Full-stack deep learning: We are moving towards a future where the demand for full-stack deep learning will continue to grow. This will result in the creation of various frameworks and libraries that will enable tech users and engineers to automate shipments tasks and various other activities. It will also help engineers to quickly adapt to new business needs and processes.
General adversarial networks (GAN): GANs provide an approach to generative modeling using deep learning algorithms, and convolutional neural networks. It produces samples that can be used to check discriminative networks and unwarranted content to balance processes and increase accuracy.
System 2 deep learning: Experts believe that system 2 DL will enable the generalization of the distribution of data. Currently, the systems need to train and test datasets with a similar distribution. System 2 DL will make it possible by using real-world data, which are also non-uniform.
Dive into the use of convolutional neural networks: CNN models are extensively used in computer vision activities like identifying objects, faces, and images. But besides CNNs, human visual systems can also recognize them under different backgrounds, angles, and views. While trying to identify images in real-world object datasets, CNN experiences a 40%-50% drop in performance.
Increased use of edge intelligence: Edge intelligence transforms the methods of acquiring data and processing it. It converts the procedures from data storage devices in the cloud to the edge. The advent of EI has made the data storage devices somewhat independent by moving decision-making closer to the data source.
Multi-modal learning in DL: AI has gotten better in multi-modalities within a single ML model, such as text, vision, speech, and other technologies. Developers are now trying to integrate these modalities in machine learning and deep learning to improve networking and efficiency in tasks.
Higher level of NLP: Currently, ML-based NLP is at its nascent stage. But currently, there is no such algorithm that will enable NLP systems to identify the meanings of different words in different situations and act accordingly. Implementing DL will boost the efficacy of these NLP systems and help machines understand customer queries swiftly.
All it takes for Real-Time Deepfakes to Fail is a '90 Degree Turn'
How Can Machine Learning Chatbots Help Businesses?
How Companies Make Use of Unstructured Data for Business Intelligence?
Forget GPT-3, Now AI Can Upscale the Old Video Collection
Metawashing is the New Tactic Brands use to Whitewash the Sustainability Lies
Why Is it Profitable to Invest in Web3 Startups Before 2022 Ends?
Top 10 Data Warehousing Jobs Pros Should Apply for in August
Is Elon Regretting his Decision to Sell Bitcoin Too Early?
Top 10 Meme coins That Will Take Over Shiba Inu Buzz in 2023