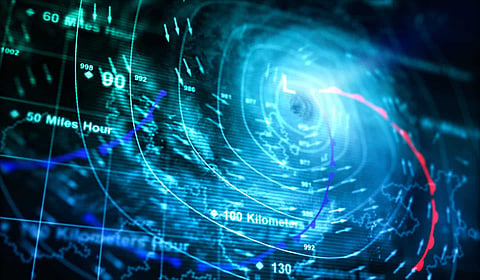
The last time you bothered to check weather forecast updates was for perhaps when you were planning for the next weekend getaway with your friends or a trip to Hawaii. Then you either typed the same in your search engine or listened to the local news weather report or called an old pal to enquire. But weather forecast updates might mean different for business people, government and farmers. It helps them prepare more meticulously in response to the possible natural disaster that might occur. It can help farmers make decisions about farming, like planting and harvesting. Even airline companies will benefit in charting the routes in advance or decide about the need to cancel the flights in case of a storm or make an emergency landing. But imagine how pissed off everyone can get when a meteorologist's predictions come wrong.
Well, the traditional models of weather forecasting are based on statistical measures of numeric models, and it does not give answers in binary. The data collected can be from deep space satellites (e.g., NOAA's Deep Space Climate Observatory (DSCOVR)), weather balloons, radar systems, nowcasting weather warnings and environmental analytics and sometimes from IoT based sensors. But with ever-increasing data set, changing atmospheric conditions, the accuracy of the predictions may fluctuate, especially for more extended periods. This is where AI can prove an asset in augmenting the accuracy and reliability of the weather forecasting while reducing the workload on the human forecasters.
The AI predictions are primarily based on machine learning algorithms. By processing more complex data in a shorter span of time using linear regression principles, now meteorologists can make predictions with improved accuracy and thus saves lives and money. Machine learning can abet with other forecasts as well, including temperature, wave height, and precipitation. One such popular model is the Numerical Weather Prediction (NWP). The model studies and manipulates vast data sets relayed from weather satellites, relay stations, and radiosondes to deliver short-term weather forecasts or long-term climate predictions. Other AI techniques for weather predictions are Artificial Neural Network, Ensemble Neural Network, Backpropagation Network, Radial Basis Function Network, General Regression Neural Network, Genetic Algorithm, Multilayer Perceptron, Fuzzy clustering.
A researcher team at NOAA found that "applying AI techniques along with a physical understanding of the environment can significantly improve the prediction skill for multiple types of high-impact weather." This type of weather includes events like severe thunderstorms, tornadoes, and hurricanes. Last year another team of researchers at Penn State, AccuWeather, Inc., and the University of Almería in Spain designed a computer model that can detect the movement of "comma-shaped" clouds in the satellite images. These clouds are hard to be noticed and are associated with cyclone formations. This model can forecast results with a 99 percent accuracy, at an average of 40 seconds per prediction.
Back in 1996, IBM was one of the first companies to use computer systems to improve predictions about the weather. Now, after purchasing The Weather Company in 2016 and its properties, including weather.com, Weather Underground, the Weather Company Brand, and WSI, IBM plans to use Weather Company's extensive data set with IBM Watson's advanced cognitive computing capabilities and Cloud platform to transform the weather forecasting future. The result of this merger is IBM Deep Thunder. Deep thunder offers its' business customers hyper-local weather forecasts with a 0.2 to 1.2-mile resolution.
Google's AI forecast tool that is based on the UNET convolutional neural network (CNN) allows researchers to generate accurate rainfall predictions six hours ahead of when the precipitation occurs. CNN is a sequence of layers of mathematical operations arranged in an encoding phase. It takes the input satellite imagery and then transforms them into output images. The layers iteratively decrease the resolution of the images passing through them in an encoding phase, and the low-dimensional representations of the image created by the encoding phase are expanded back to higher resolutions in the following decoding phase.
In his team's research paper, ML for Precipitation Nowcasting from Radar Images, Google Research's Senior Software Engineer, Jason Hickey says, "If it takes 6 hours to compute a forecast, that allows only 3-4 runs per day and results in forecasts based on 6+ hour old data, which limits our knowledge of what is happening right now." This UNET proposed tool of Google outperformed, NOAA's numerical one-hour High-Resolution Rapid Refresh (HRRR), an Optical Flow (OF) algorithm, and a persistence model, by using precision and recall graphs.
Monsanto's Climate Corporation is being used to provide agricultural weather predictions. It uses satellite imagery and hyper-local weather data along with machine learning models to enhance weather predictions, especially for the farmers. Meanwhile, researchers of École Polytechnique Fédérale de Lausanne, Switzerland used standard meteorological data and machine learning to build a simple system that can predict lightning strikes to the nearest 10 to 30 minutes inside a radius of about 18.6 miles. The team collected data about past lightning strikes from 12 Swiss weather stations in urban and mountain environments between 2006 and 2017. Then used the same data to build an algorithm to make predictions about new lightning strikes, in a process called hindcasting. The algorithm has an accuracy of 80 percent. Last year, Panasonic's AI models helped in making the most accurate predictions about Hurricane Irma's landfall on Marco Island, Florida, especially in determining where the storm would head four to seven days in advance.
The weather forecasting features of AI are multifold. From helping in disaster management to logistics and retail industry to agriculture. Someday we can use it for radar imagery to detect storm centers, high precipitation in the world and pest seasons. But one should not forget that despite boosting the accuracy levels, weather forecasting can never be a hundred percent specific. The idea is to overcome the current shortcoming in the prediction and analysis process, which is where AI is filling the gaps.